I want your feedback to make the book better for you and other readers. If you find typos, errors, or places where the text may be improved, please let me know. The best ways to provide feedback are by GitHub or hypothes.is annotations.
Opening an issue or submitting a pull request on GitHub: https://github.com/isaactpetersen/Principles-Psychological-Assessment
Adding an annotation using hypothes.is.
To add an annotation, select some text and then click the
symbol on the pop-up menu.
To see the annotations of others, click the
symbol in the upper right-hand corner of the page.
Chapter 16 Test Bias
16.1 Overview of Bias
There are multiple definitions of the term “bias” depending on the context. In general, bias is a systematic error (Reynolds & Suzuki, 2012). Mean error is an example of systematic error, and is sometimes called bias. Cognitive biases are systematic errors in thinking, including confirmation bias and hindsight bias. Method biases are a form of systematic error that involve the influence of measurement on a person’s score that is not due to the person’s level on the construct. Method biases include response biases or response styles, including acquiescence and social desirability bias. Attentional bias refers to the tendency to process some types of stimuli more than others.
Sometimes bias is used to refer in particular to systematic error (in measurement, prediction, etc.) as a function of group membership, where test bias refers to the same score having different meaning for different groups. Under this meaning, a test is unbiased if a given test score has the same meaning regardless of group membership. For example, a test is biased if there is differential validity of test scores for groups (e.g., age, education, culture, race, sex). Test bias would exist, for instance, if a test is a less valid predictor for racial minorities or linguistic minorities. Test bias would also exist if scores on the Scholastic Aptitude Test (SAT) under-estimate women’s grades in college, for instance.
There are some known instances of test bias, as described in Section 16.3. Research has not produced much empirical evidence of test bias (Brown et al., 1999; Hall et al., 1999; Jensen, 1980; Kuncel & Hezlett, 2010; Reynolds et al., 2021; Reynolds & Suzuki, 2012; Sackett et al., 2008; Sackett & Wilk, 1994), though some item-level bias is not uncommon. Moreover, where test bias has been observed, it is often small, unclear, and does not always generalize (N. S. Cole, 1981). However, just because there is not much empirical evidence of test bias does not mean that test bias does not exist. Moreover, just because a test does not show bias does not mean that it should be used. Furthermore, just because a test does not show bias does not mean that there are not race-, social class-, and gender-related biases in clinical judgment during the assessment process.
It is also worth pointing out that group differences in scores do not necessarily indicate bias. Group differences in scores could reflect true group differences in the construct. For instance, women have better verbal abilities, on average, compared to men. So, if women’s scores on a verbal ability test are higher on average than men’s scores, this would not be sufficient evidence for bias.
There are two broad categories of test bias:
Predictive bias refers to differences between groups in the relation between the test and criterion. As with all criterion-related validity tests, the findings depend on the strength and quality of the criterion. Test structure bias refers to differences in the internal test characteristics across groups.
16.2 Ways to Investigate/Detect Test Bias
16.2.1 Predictive Bias
Predictive bias exists when differences emerge between groups in terms of predictive validity to a criterion. It is assessed by using a regression line looking at the association between test score and job performance. For instance, consider a 2x2 confusion matrix used for the standard prediction problem. A confusion matrix for whom to select for a job is depicted in Figure 16.1.

Figure 16.1: 2x2 Confusion Matrix for Job Selection. TP = true positive; TN = true negative; FP = false positive; FN = false negative.
We can also visualize the confusion matrix in terms of a scatterplot of the test scores (i.e., predicted job performance) and the “truth” scores (i.e., actual job performance), as depicted in Figure 16.2. The predictor (test score) is on the x-axis. The criterion (job performance) is on the y-axis. The quadrants reflect the cutoffs (i.e., thresholds) imposed from the 2x2 confusion matrix. The vertical line reflects the cutoff for selecting someone for a job. The horizontal line reflects the cutoff for good job performance (i.e., people who should have been selected for the job).
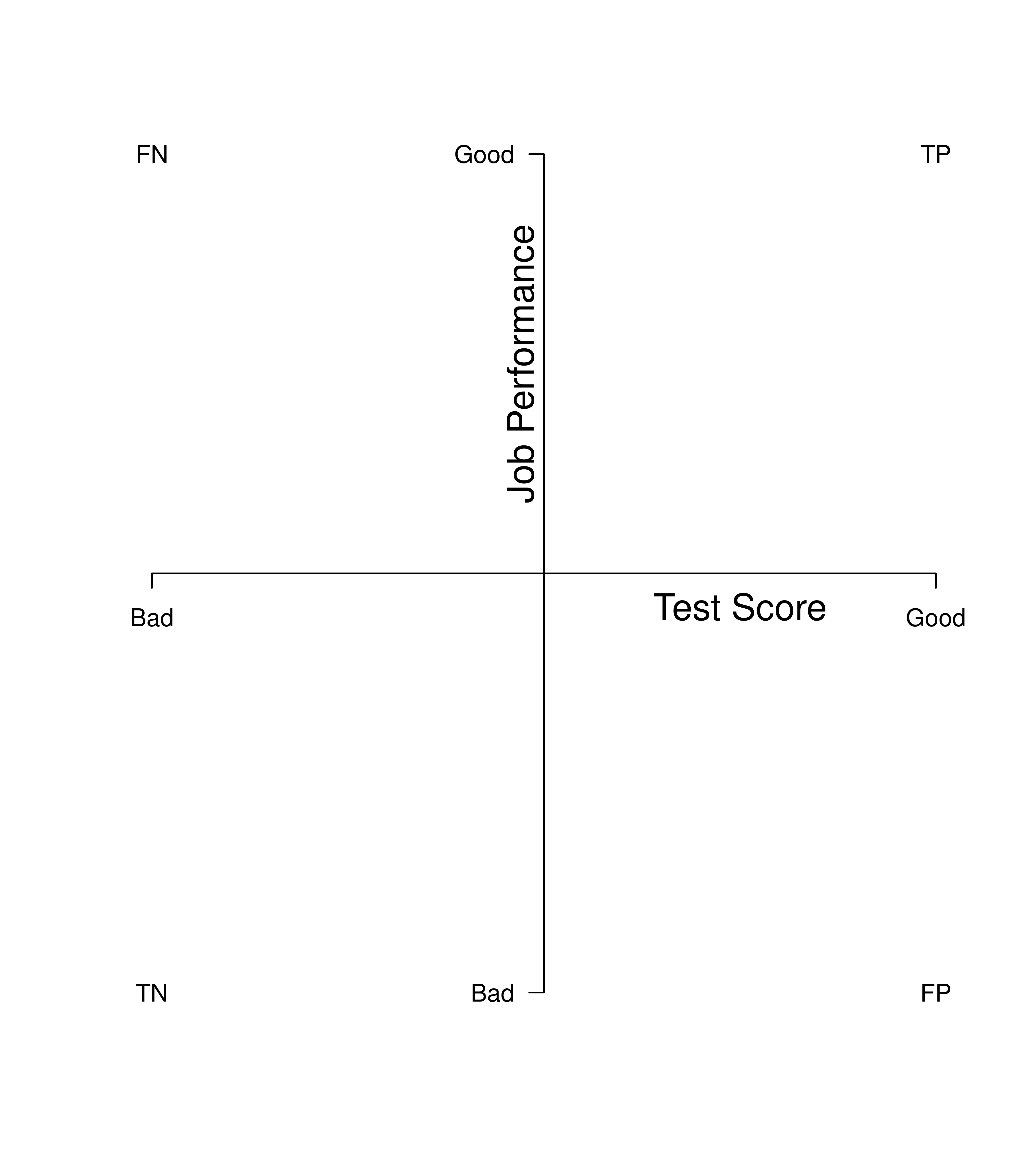
Figure 16.2: 2x2 Confusion Matrix for Job Selection in the Form of a Graph With Predicted Performance on the x-Axis and Actual Job Performance on the y-Axis. TP = true positive; TN = true negative; FP = false positive; FN = false negative.
The data points in the top right quadrant are true positives: people who the test predicted would do a good job and who did a good job. The data points in the bottom left quadrant are true negatives: people who the test predicted would do a poor job and who would have done a poor job. The data points in the bottom right quadrant are false positives: people who the test predicted would do a good job and who did a poor job. The data points in the top left quadrant are false negatives: people who the test predicted would do a poor job and who would have done a good job.
Figure 16.3 depicts a strong predictor. The best-fit regression line has a steep slope where there are lots of data points that are true positives and true negatives, with relatively few false positives and false negatives.
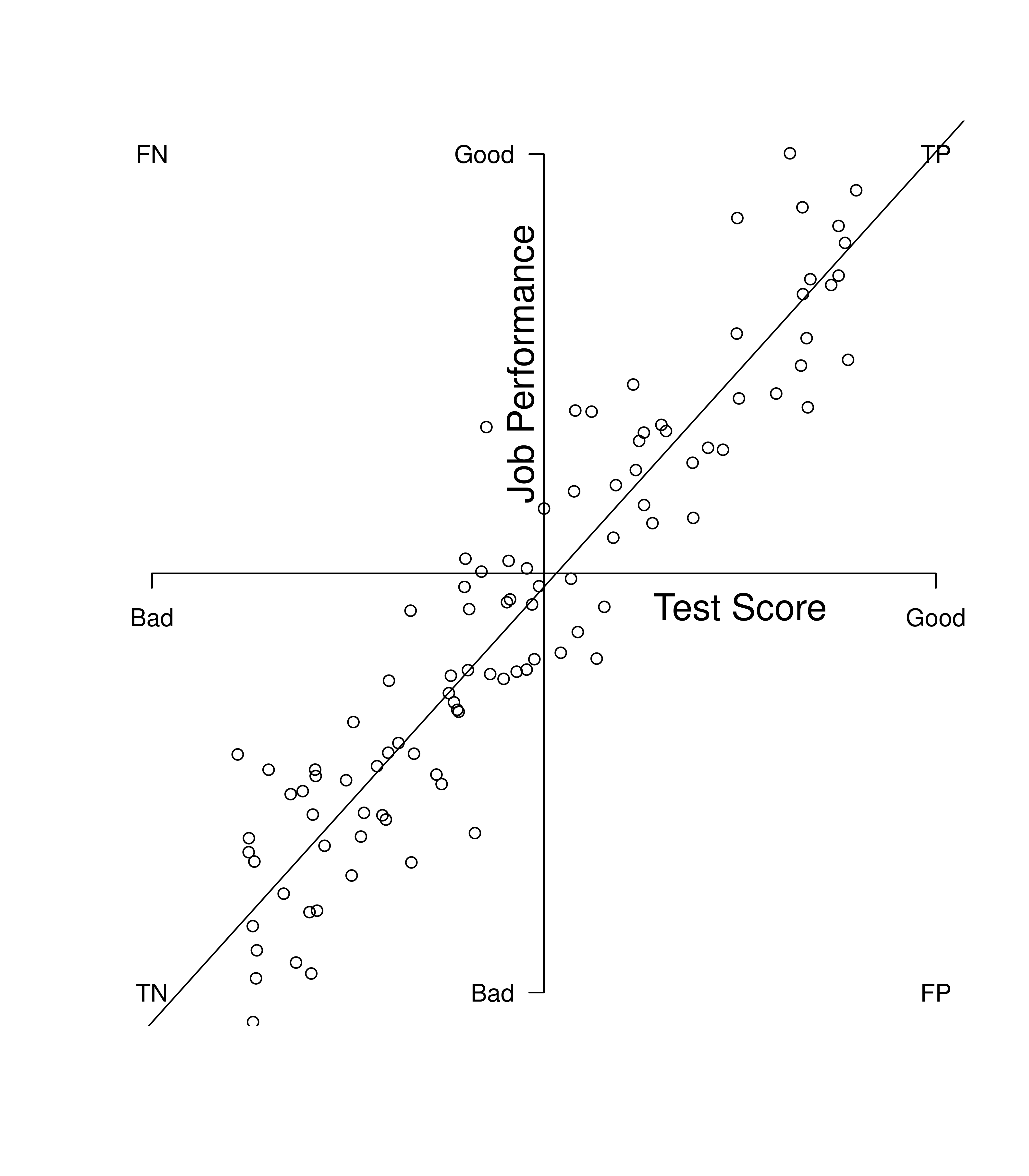
Figure 16.3: Example of a Strong Predictor. TP = true positive; TN = true negative; FP = false positive; FN = false negative.
Figure 16.4 depicts a poor predictor. The best-fit regression line has a shallow slope where there are just as many data points that are in the false cells (false positives and false negatives) as there are in the true cells (true positives and true negatives). In general, the steeper the slope, the better the predictor.
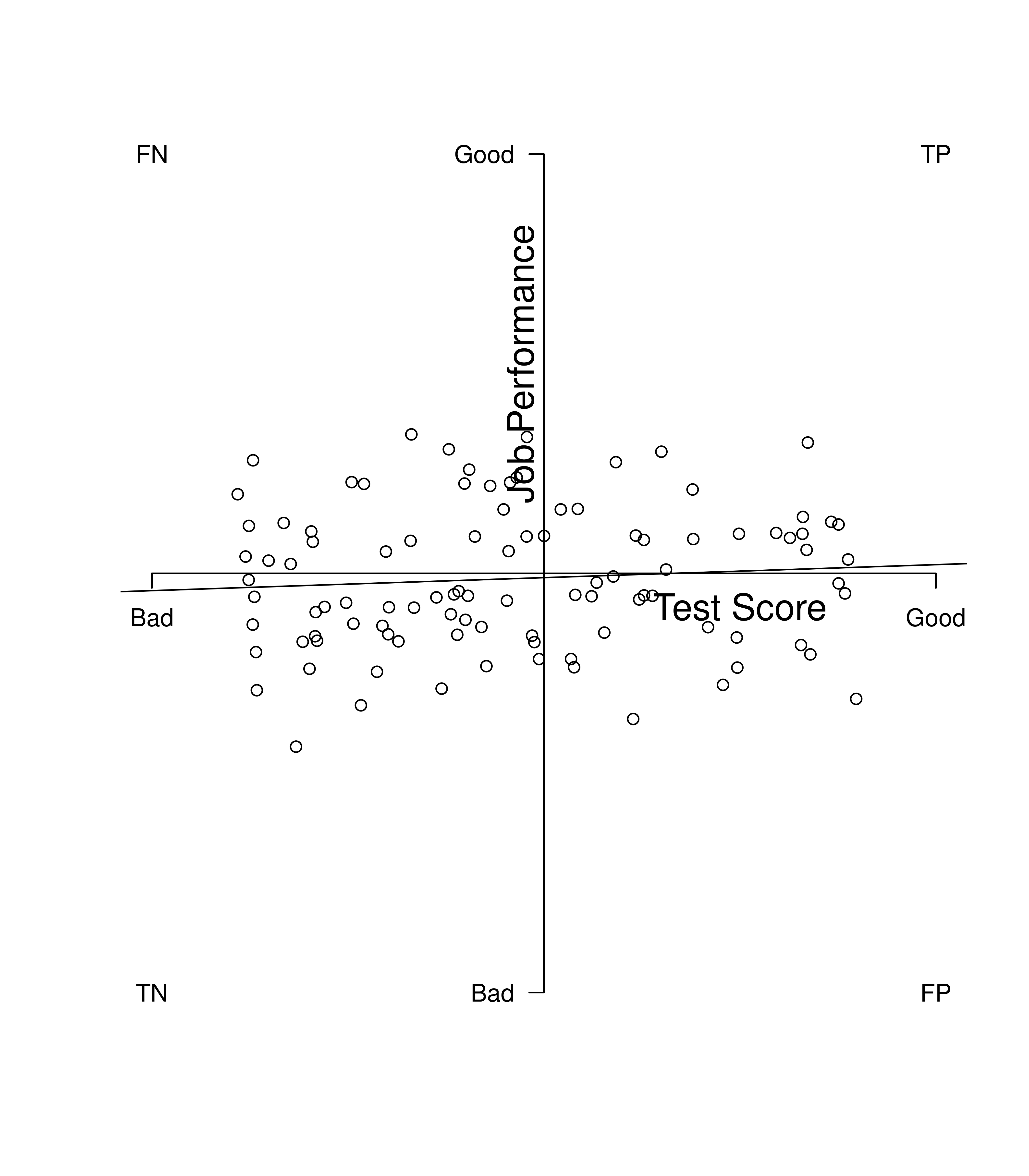
Figure 16.4: Example of a Poor Predictor. TP = true positive; TN = true negative; FP = false positive; FN = false negative.
We can evaluate predictive bias using a best-fit regression line between the predictor and criterion for each group.
16.2.1.1 Types of Predictive Bias
There are three types of predictive bias:
The slope of the regression line is the steepness of the line. The intercept of the regression line is the y-value of the point where the line crosses the y-axis (i.e., when \(x = 0\)). If a measure shows predictive test bias, when looking at the regression line for each group, the groups’ regression lines differ in either slopes and/or intercepts.
16.2.1.1.1 Different Slopes
Predictive bias in terms of different slopes exists when there are differences in the slope of the regression line between minority and majority groups. The slope describes the direction and steepness of the regression line. The slope of a regression line is the amount of change in \(y\) for every unit change in \(x\) (i.e., rise over run). Differing slopes indicate differential predictive validity, in which the test is a more effective predictor of performance in one group over the other. Different slopes predictive bias is depicted in Figure 16.5. In the figure, the predictor performs well in the majority group. However, the slope is close to zero in the minority group, indicating that there is no association between the predictor and the criterion for the minority group.
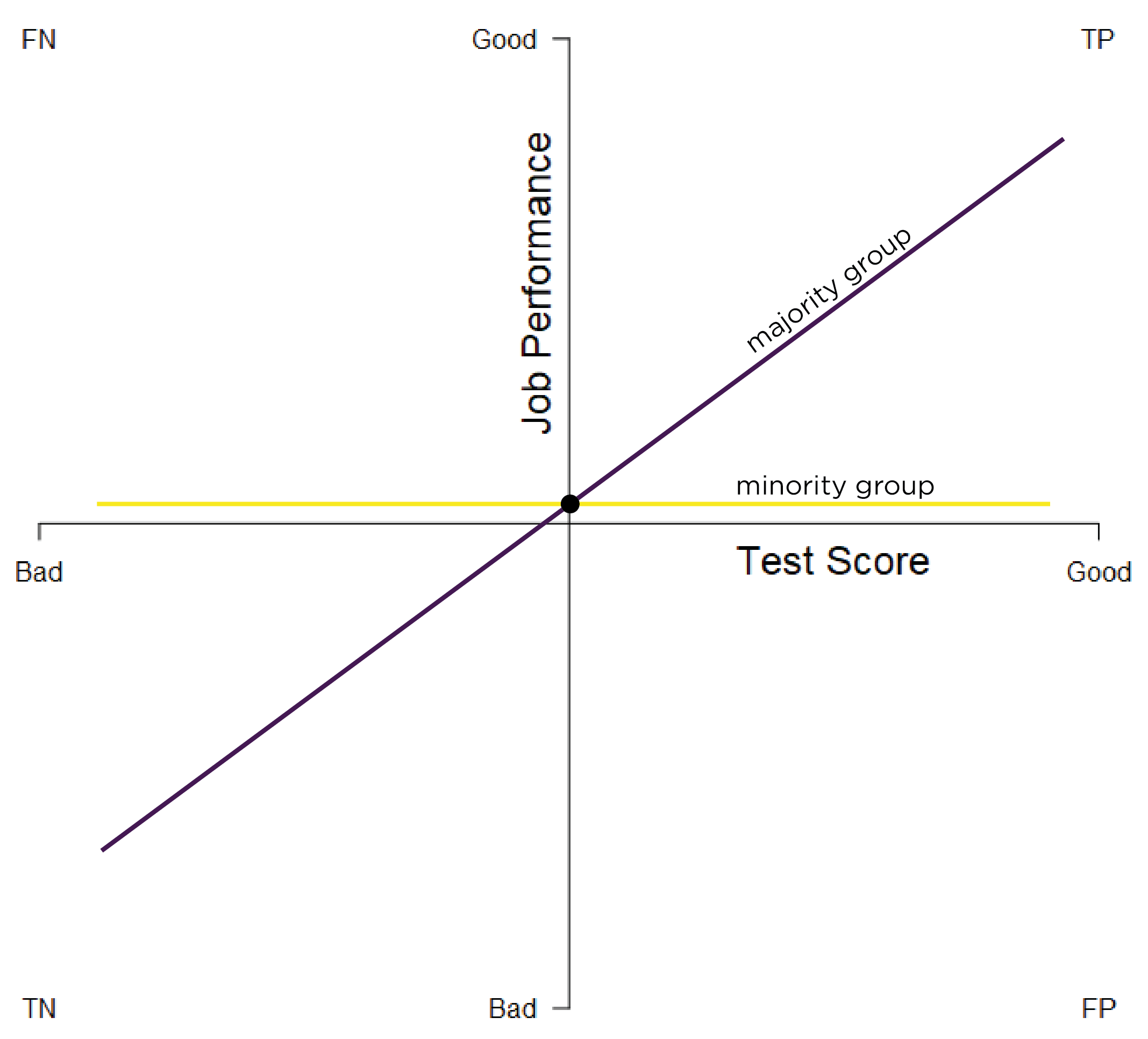
Figure 16.5: Test Bias: Different Slopes. TP = true positive; TN = true negative; FP = false positive; FN = false negative.
Different slopes can especially occur if we develop our measure and criterion based on the normative majority group. Not much evidence has found empirical evidence of different slopes across groups. However, samples often do not have the power to detect differing slopes (Aguinis et al., 2010). Theoretically, to fix biases related to different slopes, you should find another measure that is more predictive for the minority group. If the predictor is a strong predictor in both groups but shows slight differences in the slope, within-group norming could be used.
16.2.1.1.2 Different Intercepts
Predictive bias in terms of different intercepts exists when there are differences in the intercept of the regression line between minority and majority groups. The \(y\)-intercept describes the point on the \(y\)-axis that the line intersects with the \(y\)-axis (when \(x = 0\)). When the distributions have similar slopes, intercept differences suggest that the measure systematically under- or over-estimates group performance relative to the person’s ability. The same test score leads to systematically different predictions for the majority and minority groups. In other words, minority group members get different tests scores than majority group members with the same ability. Different intercepts predictive bias is depicted in Figure 16.6.
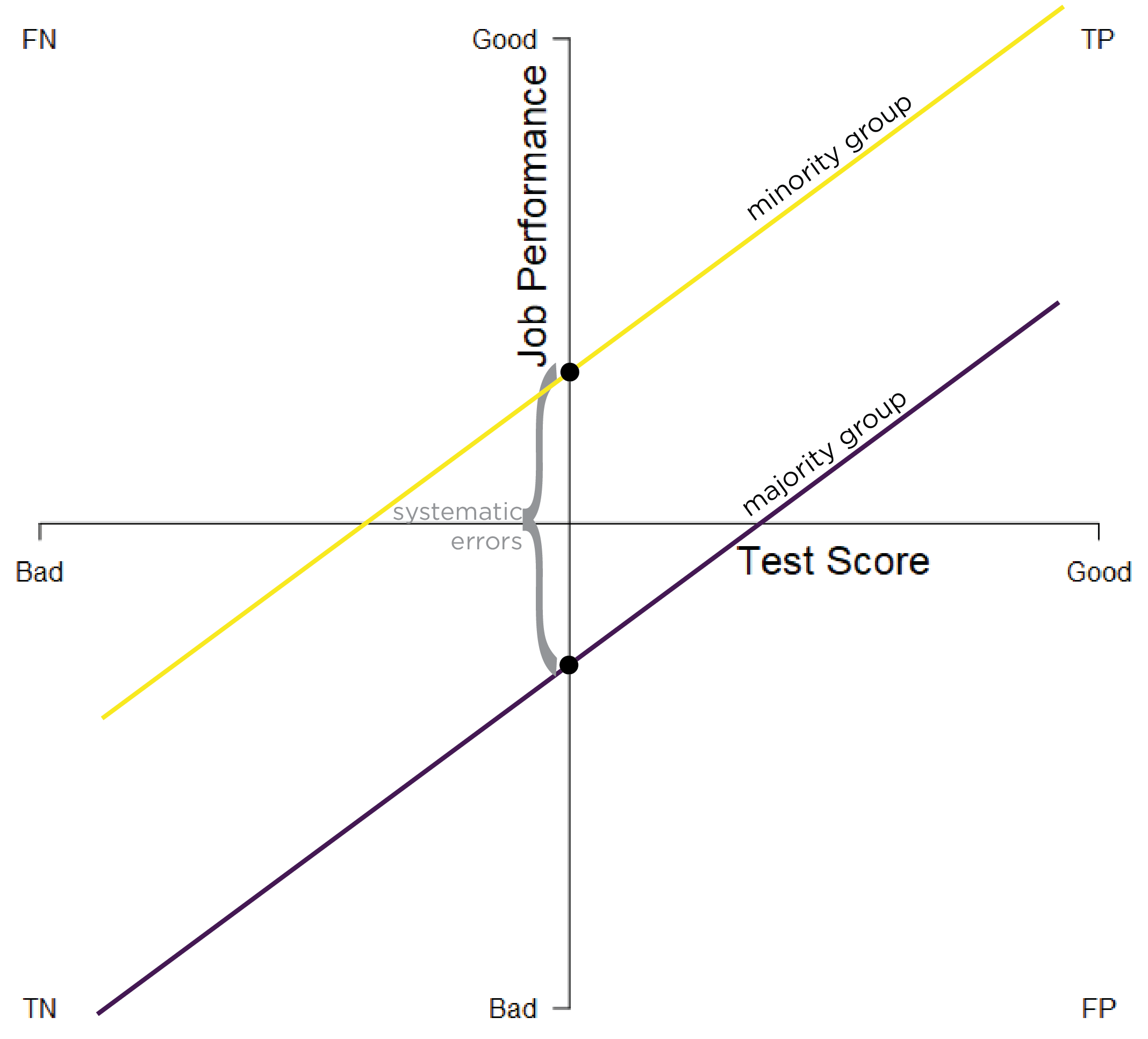
Figure 16.6: Test Bias: Different Intercepts. TP = true positive; TN = true negative; FP = false positive; FN = false negative.
A higher intercept (relative to zero) indicates that the measure under-estimates a person’s ability (at that test score)—i.e., the person’s job performance is better than what the test score would suggest. A lower intercept (relative to zero) indicates that the measure over-estimates a person’s ability (at that test score)—i.e., the person’s job performance is worse than what the test score would suggest. Figure 16.6 indicates that the measure systematically under-estimates the job performance of the minority group.
Performance among members of a minority group could be under- or over-estimated. For example, historically, women’s grades in math and engineering classes tended to be under-estimated by the Scholastic Aptitude Test [SAT; M. J. Clark & Grandy (1984)]. However, where intercept differences have been observed, measures often show small over-estimation of school and job performance among minority groups (Reynolds & Suzuki, 2012). For example, women’s physical strength and endurance is over-estimated based on physical ability tests (Sackett & Wilk, 1994). In addition, over-estimation of African Americans’ and Hispanics’ school and job performance has been observed based on cognitive ability tests (N. S. Cole, 1981; Reynolds & Suzuki, 2012; Sackett et al., 2008; Sackett & Wilk, 1994). At the same time, the Black–White difference in job performance is less than the Black–White difference in test performance.
The over-prediction of lower-scoring groups is likely mostly an artifact of measurement error (L. S. Gottfredson, 1994). The over-estimation of African Americans’ and Hispanics’ school and job performance may be due to measurement error in the tests. Moreover, test scores explain only a portion of the variation in job performance. Black people are far less disadvantaged on the noncognitive determinants of job performance than on the cognitive ones. Nevertheless, the over-estimation that has been often observed is on average—the performance is not over-estimated for all individuals of the groups even if there is an average over-estimation effect. In addition, simulation findings indicate that lower intercepts (i.e., over-estimation) among minority groups compared to majority groups could be observed if there are different slopes but not different intercepts in the population, because different slopes are likely to go undetected due to low power (Aguinis et al., 2010). That is, if a test shows weaker validity for a minority group than the majority group, it could appear as different intercepts that favor the minority group when, in fact, it reflects shallower slopes of the minority group that go undetected.
Predictive biases in intercepts could especially occur if we develop tests that are based on the majority group, and the items assess constructs other than the construct of interest which are systematically biased in favor of the majority group or against the minority group. Arguments about reduced power to detect differences are less relevant for intercepts and means than for slopes.
To correct for a bias in intercepts, we could add bonus points to the scores for the minority group to correct for the amount of the systematic error, and to result in the same regression line. But if the minority group is over-predicted (as has often been the case where intercept differences have been observed), we would not want to use score adjustment to lower the minority group’s scores.
16.2.1.1.3 Different Intercepts and Slopes
Predictive bias in terms of different intercepts and slopes exists when there are differences in the intercept and slope of the regression line between minority and majority groups. In cases of different intercepts and slopes, there is both differential validity (because the regression lines have different slopes), as well as varying under- and over-estimation of groups’ performance at particular scores. Different intercepts and slopes predictive bias is depicted in Figure 16.7.
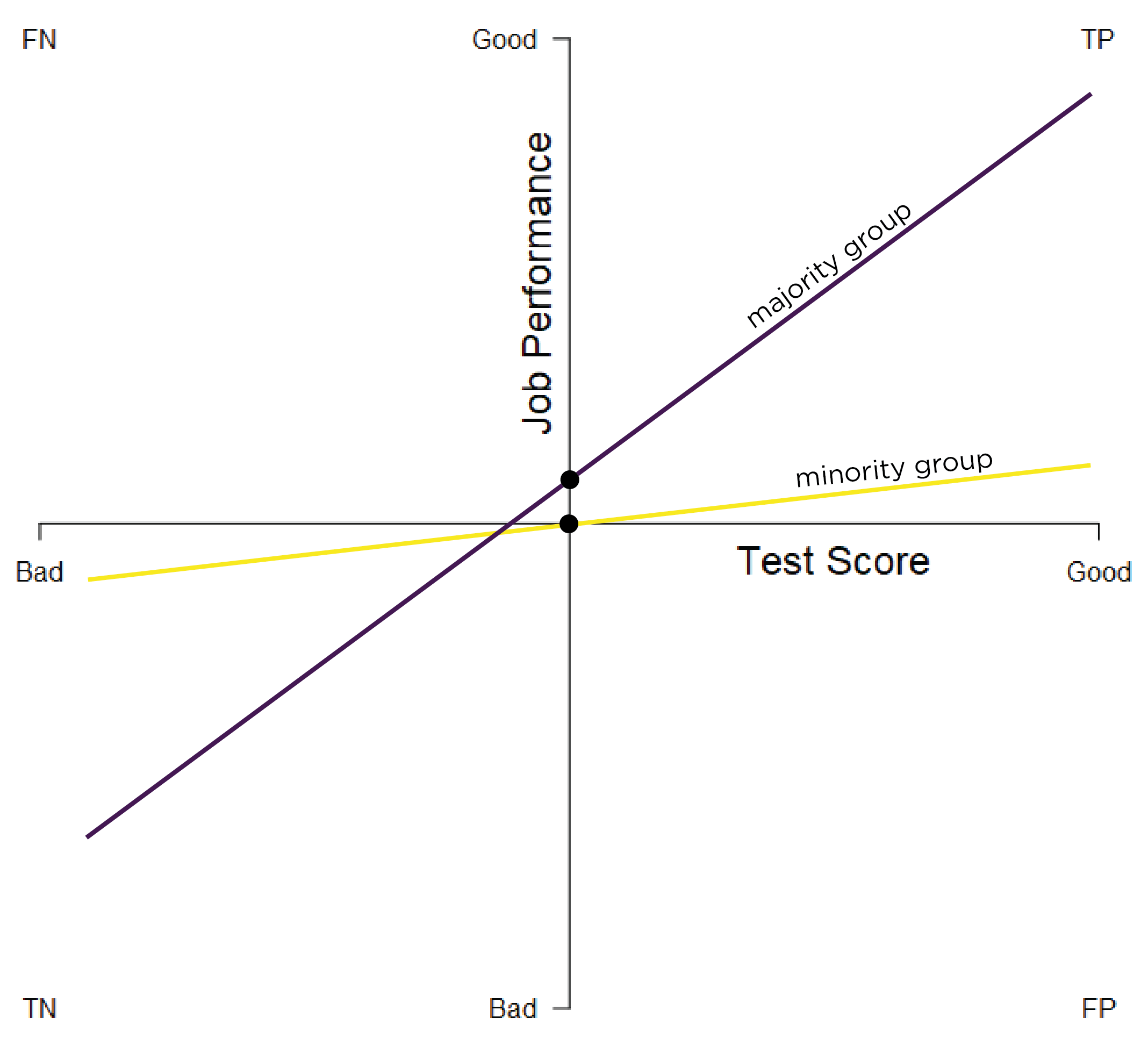
Figure 16.7: Test Bias: Different Intercepts and Slopes. TP = true positive; TN = true negative; FP = false positive; FN = false negative.
In instances of different intercepts and slopes predictive bias, a measure can simultaneously over-estimate and under-estimate a person’s ability at different test scores. For instance, a measure can under-estimate a person’s ability at higher test scores and can over-estimate a person’s ability at lower test scores.
Different intercepts and slopes across groups is possibly more realistic than just different intercepts or just different slopes. However, different intercepts and slopes predictive bias is more complicated to study, represent, and resolve. It is difficult to examine because of complexity, and it is not easy to fix. Currently, we have nothing to address different intercepts and slopes predictive bias. We would need to use a different measure or measures for each group.
16.2.2 Test Structure Bias
In addition to predictive bias, another type of test bias is test structure bias. Test structure bias involves differences in internal test characteristics across groups. Examining test structure bias is different from examining the total score, as is used when examining predictive bias. Test structure bias can be identified empirically or based on theory/judgment.
Empirically, test structure bias can be examined in multiple ways.
16.2.2.1 Empirical Approaches to Identification
16.2.2.1.1 Item \(\times\) Group tests (ANOVA)
Item \(\times\) Group tests in analysis of variance (ANOVA) examine whether the difference between groups on the overall score match comparisons among smaller items sets between groups. Item \(\times\) Group tests are used to rule out that items are operating in different ways in different groups. If the items operate in different ways in different groups, they do not have the same meaning across groups. For example, if we are going to use a measure for multiple groups, we would expect its items to operate similarly across groups. So, if women show higher scores on a depression measure compared to men, would also expect them to show similar elevations on each item (e.g., sleep loss).
16.2.2.1.2 Item Response Theory
Using item response theory, we can examine differential item functioning (DIF). Evidence of DIF, indicates that there are differences between group in terms of discrimination and/or difficulty/severity of items. Differences between groups in terms of the item characteristic curve (which combines the item’s discrimination and severity) would be evidence against construct validity invariance between the groups and would provide evidence of bias. DIF examines stretching and compression of different groups. As an example, consider the item “bites others” in relation to externalizing problems. The item would be expected to show a weaker discrimination and higher severity in adults compared to children. DIF is discussed in Section 16.9.
16.2.2.1.3 Confirmatory Factor Analysis
Confirmatory factor analysis allows tests of measurement invariance (also called factorial invariance). Measurement invariance examines whether the factor structure of the underlying latent variables in the test is consistent across groups. It also examines whether the manifestation of the construct differs between groups. Measurement invariance is discussed in Section 16.10.
Even if you find the same slope and intercepts across groups in a prediction model, the measure would still be assessing different constructs across groups if the measure has a different factor structure between the groups. A different factor structure across groups is depicted in Figure 16.8.
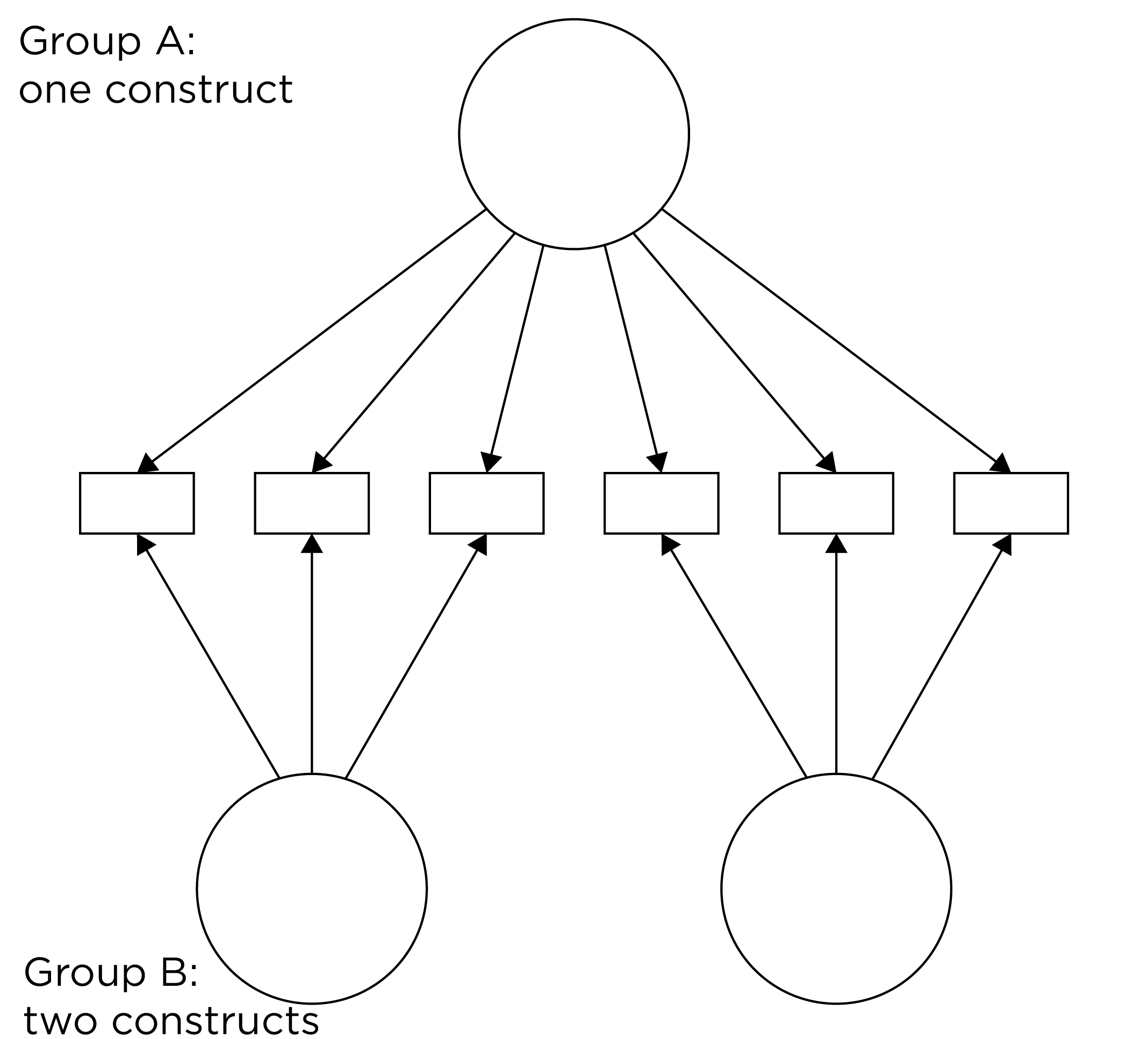
Figure 16.8: Different Factor Structure Across Groups.
An example of a different factor structure across groups is the differentiation of executive functions from two factors to three factors (inhibition, working memory, cognitive flexibility) across childhood (Lee et al., 2013).
There are different degrees of measurement invariance (for a review, see Putnick & Bornstein, 2016):
- Configural invariance: same number of factors in each group, and which indicators load on which factors are the same in each group (i.e., the same pattern of significant loadings in each group).
- Metric (“weak factorial”) invariance: items have the same factor loadings (discrimination) in each group.
- Scalar (“strong factorial”) invariance: items have the same intercepts (difficulty/severity) in each group.
- Residual (“strict factorial”) invariance: items have the same residual/unique variances in each group.
16.2.2.1.4 Structural Equation Modeling
Structural equation modeling is a confirmatory factor analysis (CFA) model that incorporates prediction. Structural equation modeling allows examining differences in the underlying structure with differences in prediction in the same model.
16.2.2.1.5 Signal Detection Theory
Signal detection theory is a dynamic measure of bias. It allows examining the overall bias in selection systems, including both accuracy and errors at various cutoffs (sensitivity, specificity, positive predictive value, and negative predictive value), as well as accuracy across all possible cutoffs (the area under the receiver operating characteristic curve). While there may be similar predictive validity between groups, the type of errors we are making across groups might differ. It is important to decide which types of error to emphasize depending on the fairness goals and examining sensitivity/specificity to adjust cutoffs.
16.2.2.1.6 Empirical Evidence of Test Structure Bias
It is not uncommon to find items that show differences across groups in severity (intercepts) and/or discrimination (factor loadings). However, cross-group differences in item functioning tend to be small and not consistent across studies, suggesting that some of the differences may reflect Type I errors that result from sampling error and multiple testing. That said, some instances of cross-group differences in item parameters could reflect test structure bias that is real and important to address.
16.2.2.2 Theoretical/Judgmental Approaches to Identification
16.2.2.2.1 Facial Validity Bias
Facial validity bias considers the extent to which an average person thinks that an item is biased—i.e., the item has differing validity between minority and majority groups. If so, the item should be reconsidered. Does an item disfavor certain groups? Is the language specific to a particular group? Is it offensive to some people? This type of judgment moves into the realm of whether or not an item should be used.
16.2.2.2.2 Content Validity Bias
Content validity bias is determined by judgments of construct experts who look for items that do not do an adequate job assessing the construct between groups. A construct may include some content facets in one group, but may include different content facets in another group, as depicted in Figure 16.9.
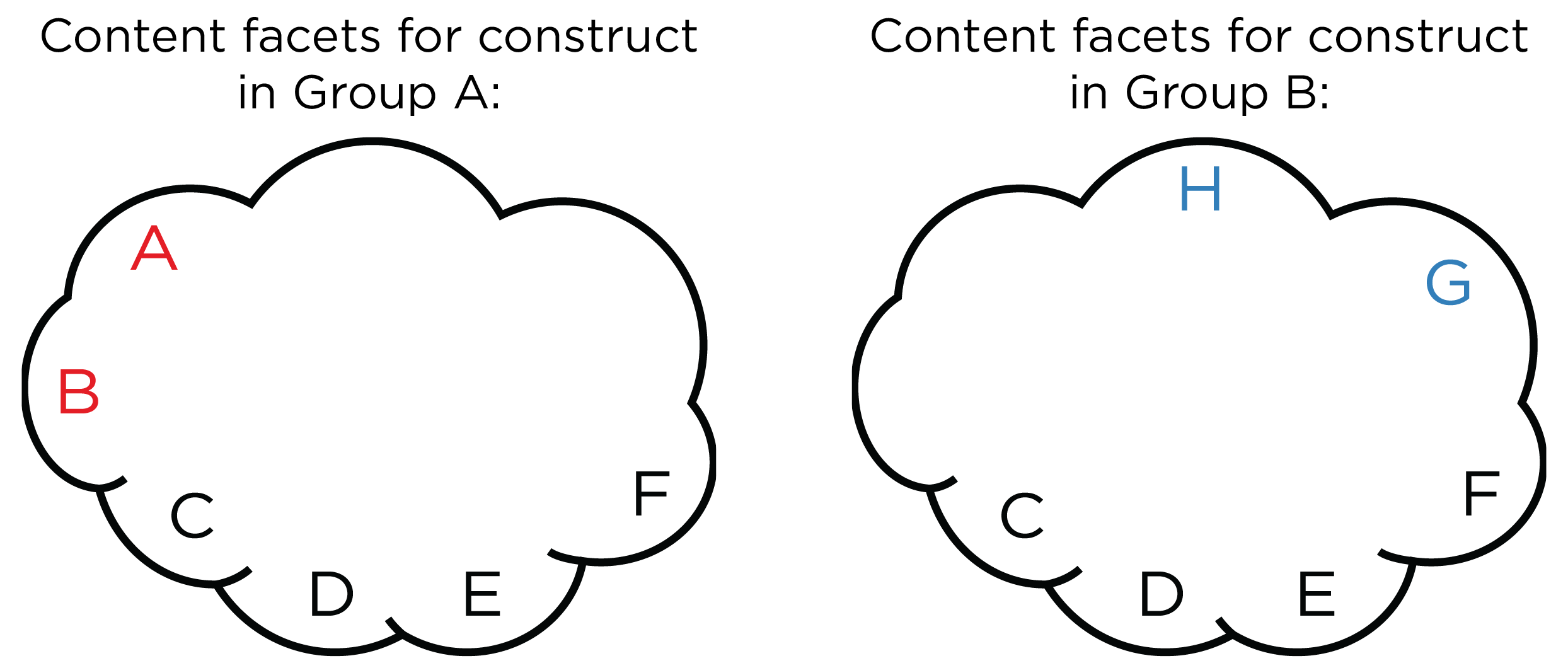
Figure 16.9: Different Content Facets in a Given Construct for Two Groups.
Examples include information questions and vocabulary questions on the Wechsler Adult Intelligence Scale. If an item is linguistically complicated, grammatically complex or convoluted, or a double negative, it may be less valid or predictive for rural populations and those with less education.
Also, stereotype threat may contribute to content validity bias. Stereotype threat occurs when people are or feel at risk of conforming themselves to stereotypes about their social group, thus leading them to show poorer performance in ways that are consistent with the stereotype. Stereotype threat may partially explain why some women may perform more poorly on some math items than some men.
Another example of content validity bias is when the same measure is used to assess a construct across ages even though the construct shows heterotypic continuity. Heterotypic continuity occurs when a construct changes in its behavioral manifestation with development (Petersen et al., 2020). That is, the same construct may look different at different points in development. An example of a construct that shows heterotypic continuity is externalizing problems. In early childhood, externalizing problems often manifest in overt forms, including physical aggression (e.g., biting) and temper tantrums. By contrast, in adolescence and adulthood, externalizing problems more often manifest in covert ways, including relational aggression and substance use. Content validity and facial validity bias judgments are often related, but not always.
16.3 Examples of Bias
As described in the overview in Section 16.1, there is not much empirical evidence of test bias (Brown et al., 1999; Hall et al., 1999; Jensen, 1980; Kuncel & Hezlett, 2010; Reynolds et al., 2021; Reynolds & Suzuki, 2012; Sackett et al., 2008; Sackett & Wilk, 1994). That said, some item-level bias is not uncommon. One instance of test bias is that, historically, women’s grades in math and engineering classes tended to be under-estimated by the Scholastic Aptitude Test [SAT; M. J. Clark & Grandy (1984)]. Fernández & Abe (2018) review the evidence on other instances of test and item bias. For instance, test bias can occur if a subgroup is less familiar with the language, the stimulus material, or the response procedures, or if they have different response styles. In addition to test bias, there are known patterns of bias in clinical judgment, as described in Section 25.3.11.
16.4 Test Fairness
There is interest in examining more than just the accuracy of measures. It is also important to examine the errors being made and differentiate the weight or value of different kinds of errors (and correct decisions). Consider an example of an unbiased test, as depicted in Figure 16.10, adapted from L. S. Gottfredson (1994). Although the example is of a White group and a Black group, we could substitute any two groups into the example (e.g., males versus females).
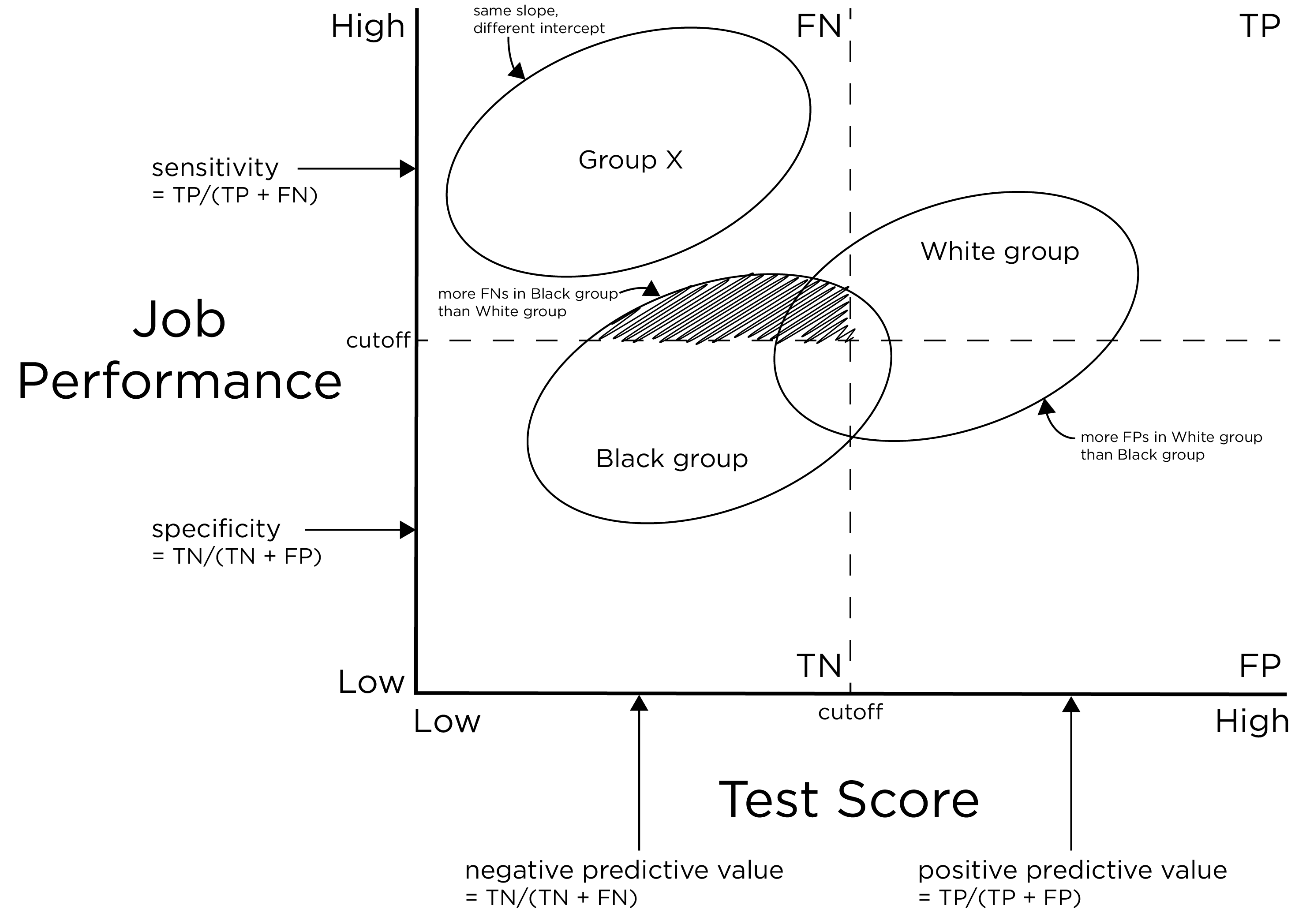
Figure 16.10: Potential Unfairness in Testing. The ovals represent the distributions of individuals’ performance both on a test and a job performance criterion. TP = true positive; TN = true negative; FP = false positive; FN = false negative. (Adapted from L. S. Gottfredson (1994), Figure 1, p. 958. Gottfredson, L. S. (1994). The science and politics of race-norming. American Psychologist, 49(11), 955–963. https://doi.org/10.1037/0003-066X.49.11.955)
The example is of an unbiased test between White and Black job applicants. There are no differences between the two groups in terms of slope. If we drew a regression line, the line would go through the centroid of both ovals. Thus, the measure is equally predictive in both groups even though that the Black group failed the test at a higher rate than the White group. Moreover, there is no difference between the groups in terms of intercept. Thus, the performance of one group is not over-estimated relative to the performance of the other group. To demonstrate what a different intercept would look like, Group X shows a different intercept. In sum, there is no predictive validity bias between the two groups. But just because the test predicts just as well in both groups does not mean that the selection procedures are fair.
Although the test is unbiased, there are differences in the quality of prediction: there are more false negatives in the Black group compared to the White group. This gives the White group an advantage and the Black group additional disadvantages. If the measure showed the same quality of prediction, we would say the test is fair. The point of the example is that just because a test is unbiased does not mean that the test is fair.
There are two kinds of errors: false negatives and false positives. Each error type has very different implications. False negatives would be when the test predicts that an applicant would perform poorly and we do not give them the job even though they would have performed well. False negatives have a negative effect on the applicant. And, in this example, there are more false negatives in the Black group. By contrast, false positives would be when we predict that an applicant would do well, and we give them the job but they perform poorly. False positives are a benefit to the applicant but have a negative effect on the employer. In this example, there are more false positives in the White group, which is an undeserved benefit based on the selection ratio; therefore, the White group benefits.
In sum, equal accuracy of prediction (i.e., equal total number of errors) does not necessarily mean the test is fair; we must examine the types of errors. Merely ensuring accuracy does not ensure fairness!
16.4.1 Adverse Impact
Adverse impact is defined as rejecting members of one group at a higher rate than another group. Adverse impact is different from test validity. According to federal guidelines, adverse impact is present if the selection rate of one group is less than four-fifths (80%) the selection rate of the group with the highest selection rate.
There is much more evidence of adverse impact than test bias. Indeed, disparate impact of tests on personnel selection across groups is the norm rather than the exception, even when using valid tests that are unbiased, which in part reflect group-related differences in job-related skills (L. S. Gottfredson, 1994). Examples of adverse impact include:
- physical ability tests, which produce substantial adverse impact against women (despite over-estimation of women’s performance),
- cognitive ability tests, which produce substantial impact against some ethnic minority groups, especially Black and Hispanic people (despite over-estimation of Black and Hispanic people’s performance), even though cognitive ability tests tend to be among the strongest predictors of job performance (Sackett et al., 2008; Schmidt & Hunter, 1981), and
- personality tests, which produce higher estimates of dominance among men than women; it is unclear whether this has predictive bias.
16.4.2 Bias Versus Fairness
Whether a measure is accurate or shows test bias is a scientific question. By contrast, whether a test is fair and thus should be used for a given purpose is not just a scientific question; it is also an ethical question. It involves the consideration of the potential consequences of testing in terms of social values and consequential validity.
16.4.3 Operationalizing Fairness
There are many perspectives to what should be considered when evaluating test fairness (American Educational Research Association et al., 2014; Camilli, 2013; Committee on the General Aptitude Test Battery et al., 1989; Dorans, 2017; Fletcher et al., 2021; Gipps & Stobart, 2009; Helms, 2006; Jonson & Geisinger, 2022; Melikyan et al., 2019; Sackett et al., 2008; Thorndike, 1971; Zieky, 2006, 2013). As described in Fletcher et al. (2021), there are three primary ways of operationalizing fairness:
- Equal outcomes: the selection rate is the same across groups.
- Equal opportunity: the sensitivity (true positive rate; 1 \(-\) false negative rate) is the same across groups.
- Equal odds: the sensitivity is the same across groups and the specificity (true negative rate; 1 \(-\) false positive rate) is the same across groups.
For example, the job selection procedure shows equal outcomes if the proportion of men selected is equal to the proportion of women selected. The job selection procedure shows equal opportunity if, among those who show strong job performance, the proportion of classification errors (false negatives) is the same for men and women. Receiver operating characteristic (ROC) curves are depicted for two groups in Figure 16.11. A cutoff that represents equal opportunity is depicted with a horizontal line (i.e., the same sensitivity) in Figure 16.11. The job selection procedure shows equal odds if (a), among those who show strong job performance, the proportion of classification errors (false negatives) is the same for men and women, and (b), among those who show poor job performance, the proportion of classification errors (false positives) is the same for men and women. A cutoff that represents equal odds is depicted where the ROC curve for Group A intersects with the ROC curve from Group B in Figure 16.11. The equal odds approach to fairness is consistent with a National Academy of Sciences committee on fairness (Committee on the General Aptitude Test Battery et al., 1989; L. S. Gottfredson, 1994). Approaches to operationalizing fairness in the context of prediction models are described by Paulus & Kent (2020).
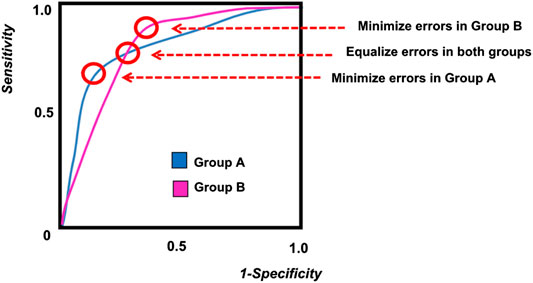
Figure 16.11: Receiver Operating Characteristic (ROC) Curves for Two Groups. (Figure reprinted from Fletcher et al. (2021), Figure 2, p. 3. Fletcher, R. R., Nakeshimana, A., & Olubeko, O. (2021). Addressing fairness, bias, and appropriate use of artificial intelligence and machine learning in global health. Frontiers in Artificial Intelligence, 3(116). https://doi.org/10.3389/frai.2020.561802)
It is not possible to meet all three types of fairness simultaneously (i.e., equal selection rates, sensitivity, and specificity across groups) unless the base rates are the same across groups or the selection is perfectly accurate (Fletcher et al., 2021). In the medical context, equal odds is the most common approach to fairness. However, using the cutoff associated with equal odds typically reduces overall classification accuracy. And, changing the cutoff for specific groups can lead to negative consequences. In the case that equal odds results in a classification accuracy that is too low, it may be worth considering using separate assessment procedures/tests for each group. In general, it is best to follow one of these approaches to fairness. It is difficult to get right, so try to minimize negative impact. Many fairness supporters argue for simpler rules. In the 1991 Civil Rights Act, score adjustments based on race, gender, and ethnicity (e.g., within-race norming or race-conscious score adjustments) were made illegal in personnel selection (L. S. Gottfredson, 1994).
Another perspective to fairness is that selection procedures should predict job performance and if they are correlated with any group membership (e.g., race, socioeconomic status, or gender), the test should not be used (Helms, 2006). That is, according to Helms, we should not use any test that assesses anything other than the construct of interest (job performance). Unfortunately, however, no measures like this exist. Every measure assesses multiple things, and factors such as poverty can have long-lasting impacts across many domains.
Another perspective to fairness is to make the selection procedures equal the number of successes within each group (Thorndike, 1971). According to this perspective, if you want to do selection, you should hire all people, then look at job performance. If among successful employees, 60% are White and 40% are Black, then set this selection rate for each group (i.e., hiring 80% White individuals and 20% Black individuals is not okay). According to this perspective, a selection system is only fair if the majority–minority differences on the selection device used are equal in magnitude to majority–minority differences in job performance. Selection criteria should be made based on prior distributions of success rates. However, you likely will not ever really know the true base rate in these situations. No one uses this approach because you would have a period where you have to accept everyone to find the percent that works. Also, this would only work in a narrow window of time because the selection pool changes over time.
There are lots of groups and subgroups. Ensuring fairness is very complex, and there is no way to accomplish the goal of being equally fair to all people. Therefore, do the best you can and try to minimize negative impact.
16.5 Correcting For Bias
16.5.1 What to Do When Detecting Bias
When examining item bias (using differential item functioning/DIF or measurement non-invariance) with many items (or measures) across many groups, there can be many tests, which will make it likely that DIF/non-invariance will be detected, especially with a large sample. Some detected DIF may be artificial or trivial, but other DIF may be real and important to address. It is important to consider how you will proceed when detecting DIF/non-invariance. Considerations of effect size and theory can be important for evaluating the DIF/non-invariance and whether it is negligible or important to address.
When detecting bias, there are several steps to take. First, consider what the bias indicates. Does the bias present adverse impact for a minority group? For what reasons might the bias exist? Second, examine the effect size of the bias. If the effects are small, if the bias does not present adverse impact for a minority group, and if there is no compelling theoretical reason for the bias, the bias might not be sufficient to scrap the instrument for the population. Some detected bias may be artificial, but other bias may be real. Gender and cultural differences have shown a number of statistically significant effects for a number of different assessment purposes, but many of the observed effects are quite small and likely trivial, and they do not present compelling reasons to change the assessment (Youngstrom & Van Meter, 2016).
However, if you find bias, correct for it! There are a number of score adjustment and non-score adjustment approaches to correct for bias, as described in Sections 16.5.2 and 16.5.3. If the bias occurs at the item level (e.g., test structure bias), it is generally recommended to remove or resolve items that show non-negligible bias. There are three primary options: (1) drop the item for both groups, (2) drop the item for one group but keep it for the other group, or (3) freely estimate the parameters for the item across groups. Addressing items that show larger bias can also reduce artificial bias in other items (Hagquist & Andrich, 2017). Thus, researchers are encouraged to handle item bias sequentially from high to low in magnitude. If the bias occurs at the test score level (e.g., predictive bias), score adjustments may be considered.
If you do not correct for bias, consider the impact of the test, procedure, and selection procedure when interpreting scores. Interpret scores with caution and provide necessary caveats in resulting papers or reports regarding the interpretations in question. In sum, it is important to examine the possibility of bias—it is important to consider how much “erroneous junk” you are introducing into your research.
16.5.2 Score Adjustment to Correct for Bias
Score adjustment involves adjusting scores for a particular group or groups.
16.5.2.1 Why Adjust Scores?
There may be several reasons to adjust scores for various groups in a given situation. First, there may be social goals to adjust scores. For example, we may want our selection device to yield personnel that better represent the nation or region, including diversity of genders, races, majors, social classes, etc. Score adjustments are typically discussed with respect to racial minority differences due to historical and systemic inequities. Our society aims to provide equal opportunity, including the opportunity to gain a fair share (i.e., proportional representation) of jobs. A diversity of perspectives in a job is a strength; a diversity of perspectives can lead to greater creativity and improved problem-solving. A second potential reason that we may want to apply score adjustment is to correct for bias. A third potential reason that we may want to apply score adjustment is to improve the fairness of a test.
16.5.2.2 Types of Score Adjustment
There are a number of potential techniques that have been used in attempts to correct for bias, i.e., to reduce negative impact of the test on an under-represented group. What is considered an under-represented group may depend on the context. For instance, men are under-represented compared to women as nurses, preschool teachers, and college students. However, men may not face the same systemic challenges compared to women, so even though men may show under-representation in some domains, it is arguable whether scores should be adjusted to increase their representation. Techniques for score adjustment include:
- Bonus points
- Within-group norming
- Separate cutoffs
- Top-down selection from different lists
- Banding
- Banding with bonus points
- Sliding band
- Separate tests
- Item elimination based on group differences
16.5.2.2.1 Bonus Points
Providing bonus points involves adding a constant number of points to the scores of all individuals who are members of a particular group with the goal of eliminating or reducing group differences. Bonus points is used to correct for predictive bias differences in intercepts between groups. An example of bonus points is military veterans in placement for civil service jobs—points are added to the initial score for all veterans (e.g., add 5 points to test scores of all veterans). An example of using bonus points as a score adjustment is depicted in Figure 16.12.
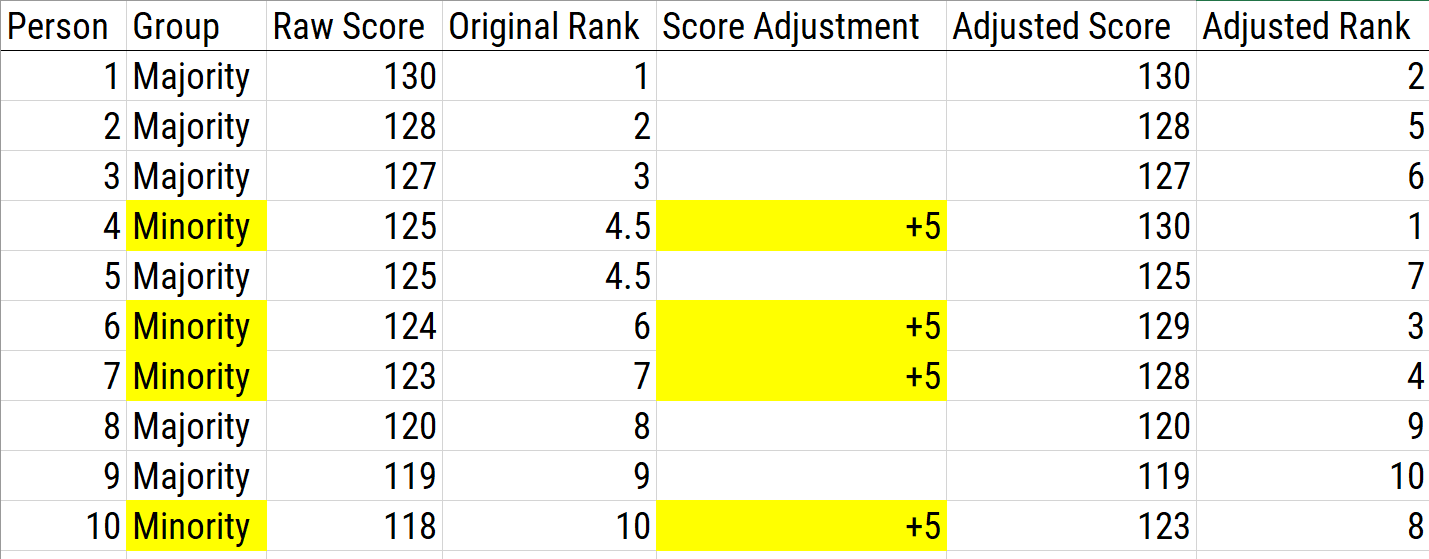
Figure 16.12: Using Bonus Points as a Scoring Adjustment.
There are several pros of bonus points. If the distribution of each group is the same, this will effectively reduce group differences. Moreover, it is a simple way of impacting test selection and procedure without changing the test, which is therefore a great advantage. There are several cons of bonus points. If there are differences in group standard deviations, adding bonus points may not actually correct for bias. The use of bonus points also obscures what is actually being done to scores, so other methods like using separate cutoffs may be more explicit. In addition, the simplicity of bonus points is also a great disadvantage because it is easily understood and often not viewed as “fair” because some people are getting extra points that others do not.
16.5.2.2.2 Within-Group Norming
A norm is the standard of performance that a person’s performance can be compared to. Within-group norming treats the person’s group in the sample as the norm. Within-group norming converts an individual’s score to standardized scores (e.g., T scores) or percentiles within one’s own group. Then, the people are selected based on the highest standard scores across groups. Withing-group norming is used to correct for predictive bias differences in slopes between groups. An example of using within-group norming as a score adjustment is depicted in Figure 16.13.
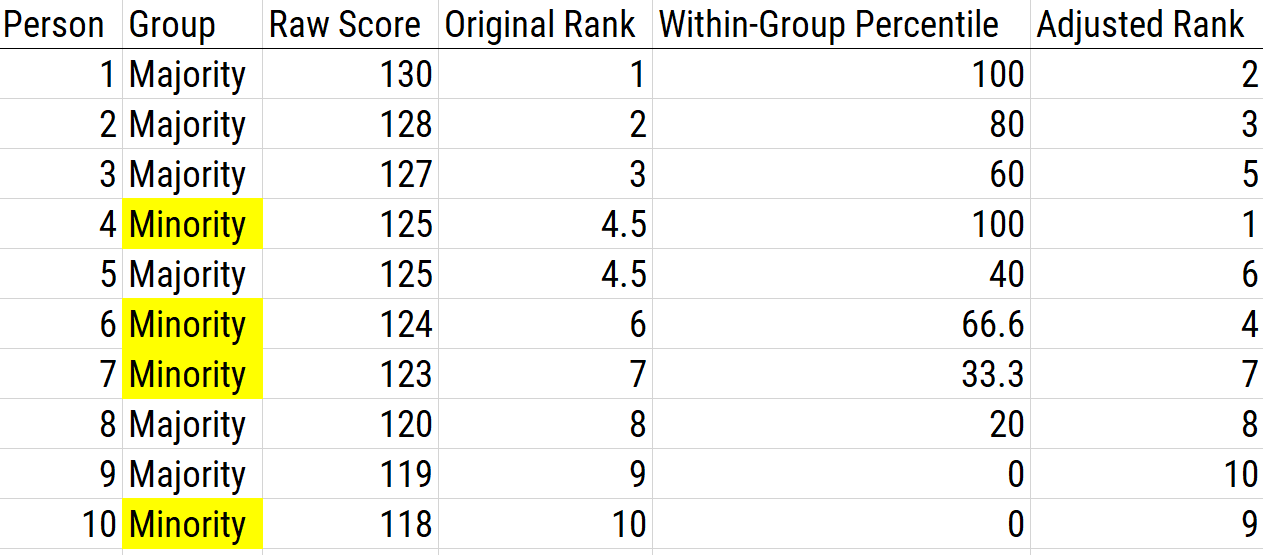
Figure 16.13: Using Within-Group Norming as a Scoring Adjustment.
There are several pros of within-group norming. First, it accounts for differences in group standard deviations and means, so it does not have the same problem as bonus points and is generally more effective at eliminating adverse impact compared to bonus points. Second, some general (non-group-specific) norms are clearly irrelevant for characterizing a person’s functioning. Group-specific norms aim to describe a person’s performance relative to people with a similar background, thus potentially reducing cultural bias. Third, group-specific norms may better reflect cultural, educational, socioeconomic, and other factors that may influence a person’s score (Burlew et al., 2019). Fourth, group-specific norms may increase specificity, and reduce over-pathologizing by preventing giving a diagnosis to people who might not show a condition (Manly & Echemendia, 2007).
There are several cons of within-group norming. First, group differences could be maintained if one decides to norm based on a reference sample or, when scores are skewed, a local sample, especially when using standardized scores. However, percentile scores will consistently eliminate adverse impact. Second, using group-specific norms may obscure background variables that explain underlying reasons for group-related differences in test performance (Manly, 2005; Manly & Echemendia, 2007). Third, group-specific norms do not address the problem if the measure shows test bias (Burlew et al., 2019). Fourth, group-specific norms may reduce sensitivity to detect conditions (Manly & Echemendia, 2007). For instance, they may prevent people from getting treatment who would benefit. It is worth noting that within-group norming on the basis of sex, gender, and ethnicity is illegal for the basis of personnel selection according to the 1991 Civil Rights Act.
As an example of within-group norming, the National Football League used to use race-norming for identification of concussions. The effect of race-norming, however, was that it lowered Black players’ concussion risk scores, which prevented many Black players from being identified as having sustained a concussion and from receiving needed treatment. Race-norming compared the Black football players cognitive test scores to group-specific norms: the cognitive test scores of Black people in the general population (not to common norms). Using Black-specific norms assumed that Black football players showed lower cognitive ability than other groups, so a low cognitive ability score for a Black player was less likely to be flagged as concerning. Thus, the race-specific norms led to lower identified rates of concussions among Black football players compared to White football players. Due to the adverse impact, Black players sued the National Football League, and the league stopped the controversial practice of race-norming for identification of concussion (https://www.washingtonpost.com/sports/2021/06/03/nfl-concussion-settlement-race-norming/; archived at https://perma.cc/KN3L-5Z7R).
A common question is whether to use group-specific norms or common norms. Group-specific norms are a controversial practice, and the answer depends. If you are interested in a person’s absolute functioning (e.g., for determining whether someone is concussed or whether they are suitable to drive), recommendations are to use common norms, not group-specific norms (Barrash et al., 2010; Silverberg & Millis, 2009). If, by contrast, you are interested in a person’s relative functioning compared to a specific group, within-group norming could make sense if there is an appropriate reference group. The question about which norms to use are complex, and psychologists should evaluate the cost and benefit of each norm, and use the norm with the greatest benefit and the least cost for the client (Manly & Echemendia, 2007).
16.5.2.2.3 Separate Cutoffs
Using separate cutoffs involves using a separate cutoff score per group and selecting the top number from each group. That is, using separate cutoffs involves using different criteria for each group. Using separate cutoffs functions the same as adding bonus points, but it has greater transparency—i.e., you are lowering the standard for one group compared to another group. An example of using separate cutoffs as a score adjustment is depicted in Figure 16.14.
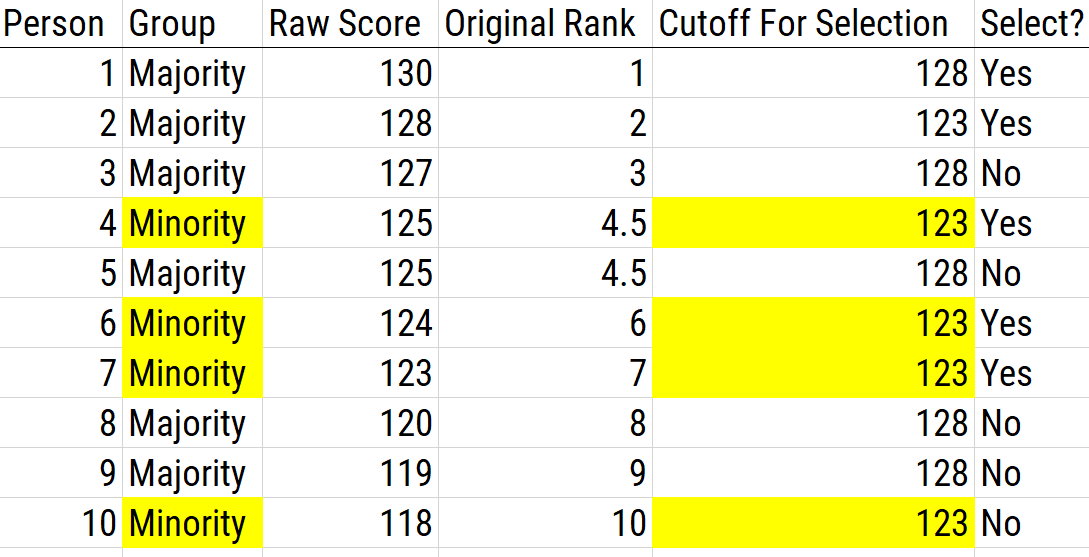
Figure 16.14: Using Separate Cutoffs as a Scoring Adjustment. In this example, the cutoff for the majority group is 128; the cutoff for the minority group is 123.
16.5.2.2.4 Top-Down Selection from Different Lists
Top-down selection from different lists involves taking the best from two different lists according to a preset rule as to how many to select from each group. Top-down selection from different lists functions the same as within-group norming. An example of using top-down selection from different lists as a score adjustment is depicted in Figure 16.15.
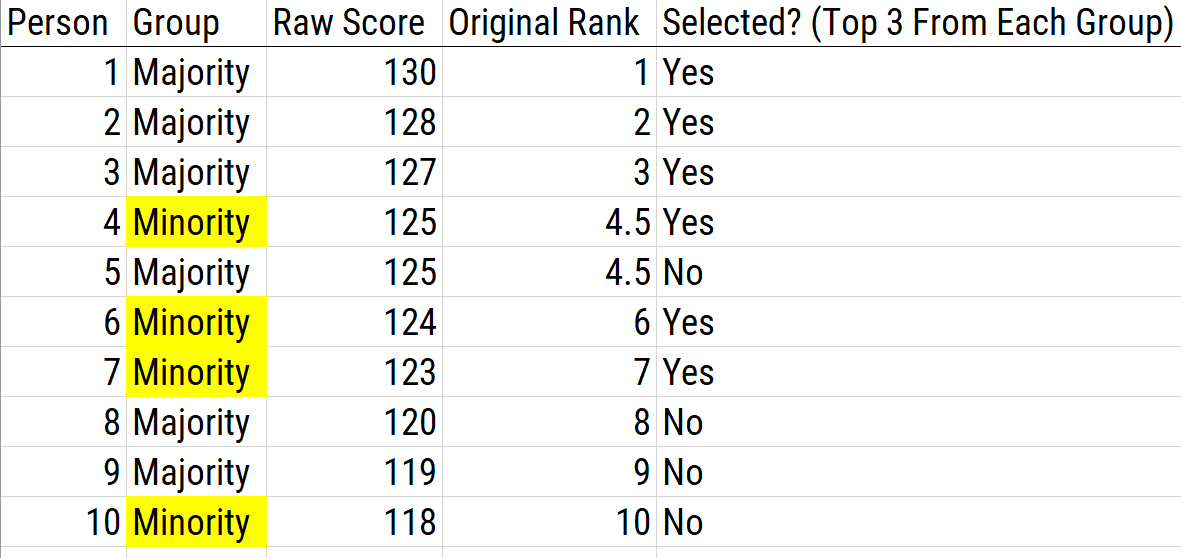
Figure 16.15: Using Top-Down Selection From Different Lists as a Scoring Adjustment. In this example, the top three candidates are selected from each group.
16.5.2.2.5 Banding
Banding uses a tier system that is based on the assumption that individuals within a specific score range are regarded as having equivalent scores. So that we do not over-estimate small score differences, scores within the same band are seen as equivalent—and the order of selection within the band can be modified depending on selection goals. The standard error of measurement (SEM) is used to estimate the precision (reliability) of the test scores, and it is used as the width of the band.
Consider an example: if a person received a score with confidence interval of 18–22, then scores between 18 to 22 are not necessarily different due to random fluctuation (measurement error). Therefore, scores in that range are considered the same, and we take a band of scores. However, banding by itself may not result in increased selection of lower scoring groups. The band provides a subsample of applicants so that we can use other criteria (other than the test) to select a candidate. Giving “minority preference” involves selecting members of minority group in a given band before selecting members of the majority group. An example of using banding as a score adjustment is depicted in Figure 16.16.
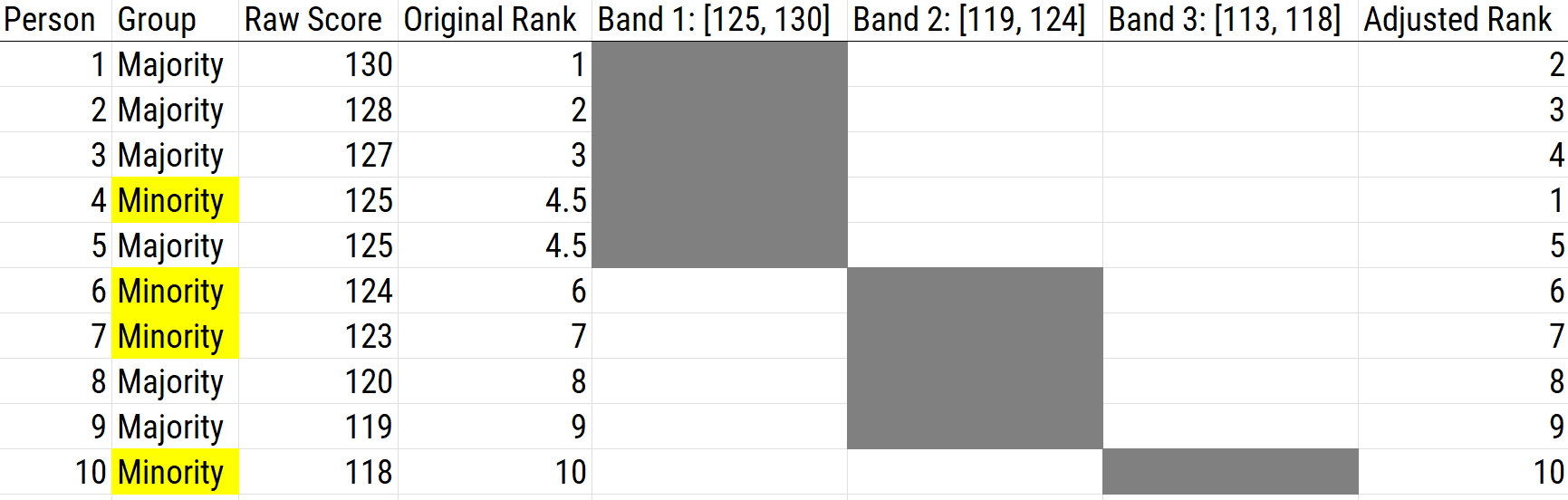
Figure 16.16: Using Banding as a Scoring Adjustment.
The problem with banding is that bands are set by the standard error of measurement: you can select the first group from the first band, but then whom do you select after the first band? There is no rationale where to “stop” the band because there are indistinguishable scores on the edges of each band to the next band. That is, 17 is indistinguishable from 18 (in terms of its confidence interval), 16 is indistinguishable from 17, and so on. Therefore, banding works okay for the top scores, but if you are going to hire a lot of candidates, it is a problem. A solution to this problem with banding is to use a sliding band, as described later.
16.5.2.2.6 Banding with Bonus Points
Banding is often used with bonus points to reduce the negative impact for minority groups. An example of using banding with bonus points as a score adjustment is depicted in Figure 16.17.
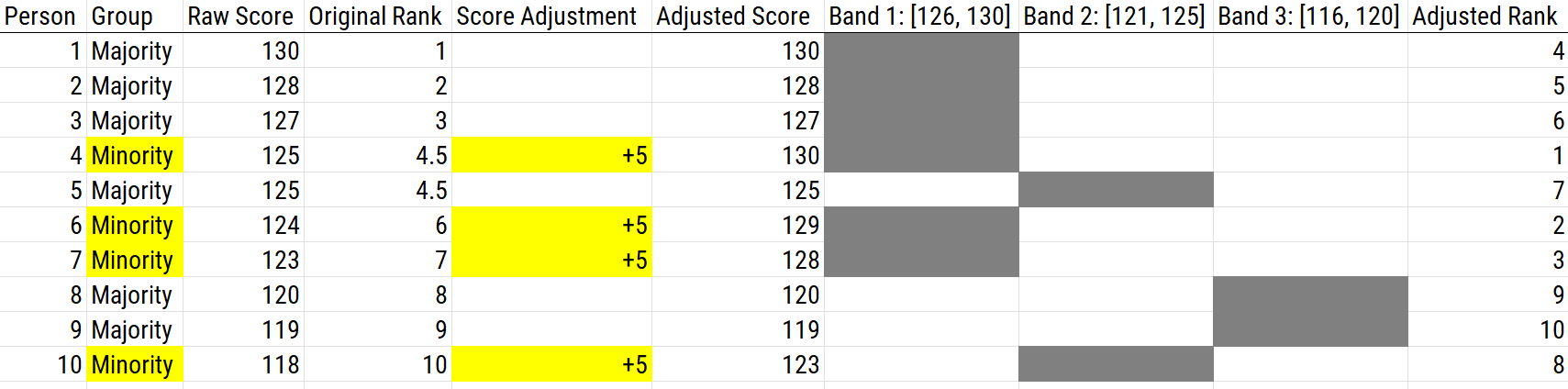
Figure 16.17: Using Banding With Bonus Points as a Scoring Adjustment.
16.5.2.2.7 Sliding Band
Using a sliding band is a solution to the problem of which bands to use when using banding. Using a sliding band can help increase the number of minorities selected. Using the top band, you select all members of a minority group in the top band, then select members of the majority group with the top score of the band, then slide the band down (based on SEM), and repeat. You work your way down with bands though groups that are indistinguishable based on SEM, until getting a cell needed to select a relevant candidate.
For instance, if the top score is 22 and the SEM is 4 points, the first band would be: [18, 22]. Here is how you would proceed:
- Select the minority group members who have a score between 18 to 22.
- Select the majority group members who have a score of 22.
- Slide the band down based on the SEM to the next highest score: [17, 21].
- Select the minority group members who have a score between 17 to 21.
- Select the majority group members who have a score of 21.
- Slide the band down based on the SEM to the next highest score: [16, 20].
- …
- And so on
An example of using a sliding band as a score adjustment is depicted in Figure 16.18.
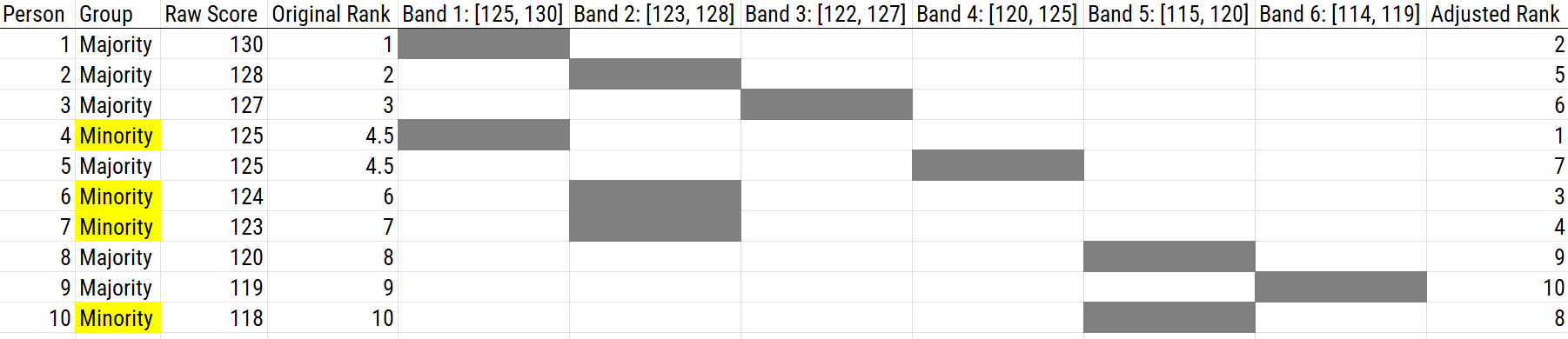
Figure 16.18: Using a Sliding Band as a Scoring Adjustment.
In sum, using a sliding band, scores that are not significantly lower than the highest remaining score should not be treated as different. Using a sliding band has the same effects on decisions as bonus points that are the width of the band. For example, if the SEM is 3, it has the same decisions as bonus points of 3; therefore, any scores within 3 of the highest score are now considered equal.
A sliding band is popular because of its scientific and statistical rationale. Also, it is more confusing and, therefore, preferred by some because it may be less likely to be sued. However, a sliding band may not always eliminate adverse impact. A sliding band has never been overturned in court (or at least, not yet).
16.5.2.2.8 Separate Tests
Using separate tests for each group is another option to reduce bias. For instance, you might use one test for the majority group and a different test for the minority group, making sure that each test is valid for the relevant group. Using separate tests is an extreme version of top-down selection and within-group norming. Using separate tests would be an option if a measure shows different slopes predictive bias.
One way of developing separate tests is to use empirical keying by group: different items for each group are selected based on each item’s association with the criterion in each group. Empirical keying is an example of dustbowl empiricism (i.e., relying on empiricism rather than theory). However, theory can also inform the item selection.
16.5.2.2.9 Item Elimination based on Group Differences
Items that show large group differences in scores can be eliminated from the test. If you remove enough items showing differences between groups, you can get similar scores between groups and can get equal group selection. A problem of item elimination based on group differences is that if you get rid of predictive items, then two goals, equal selection and predictive power, are not met. If you use this method, you often have to be willing for the measure to show decreases in predictive power.
16.5.2.3 Use of Score Adjustment
Score adjustment can be used in a number of different domains, including tests of aptitude and intelligence. Score adjustment also comes up in other areas. For example, the number of drinks it takes to be considered binge drinking differs between men (five) and women (four). Although the list of score adjustment options is long, they all really reduce to two ways:
Bonus points and within-group norming are the techniques that are most often used in the real world. These techniques differ in their degree of obscurity—i.e., confusion that is caused not for scientific reasons, but for social, political, and dissemination and implementation reasons. Often procedures that are hard to understand are preferred because it is hard to argue against, critique, or game the system. Basically, you have two options for score adjustment. One option is to adjust scores by raising scores in one group or lowering the criterion in one group. The second primary option is to renorm or change the scores. In sum, you can change the scores, or you can change the decisions you make based on the scores.
16.5.3 Other Ways to Correct for Bias
Because score adjustment is controversial, it is also important to consider other potential ways to correct for bias that do not involve score adjustment. Strategies other than score adjustment to correct for bias are described by Sackett et al. (2001).
16.5.3.1 Use Multiple Predictors
In general, high-stakes decisions should not be made based on the results from one test. So, for instance, do not make hiring decisions based just on aptitude assessments. For example, college admissions decisions are not made just based on SAT scores, but also one’s grades, personal statement, extracurricular activities, letters of recommendation, etc. Using multiple predictors works best when the predictors are not correlated with the assessment that has adverse impact, which is difficult to achieve.
There are larger majority–minority subgroup differences in verbal and cognitive ability tests than in noncognitive skills (e.g., motivation, personality, and interpersonal skills). So, it is important to include assessment of relevant noncognitive skills. Include as many relevant aspects of the construct as possible for content validity. For a job, consider as many factors as possible that are relevant for success, e.g., cognitive and noncognitive abilities.
16.5.3.2 Change the Criterion
Another option is to change the criterion so that the predictive validity of tests is less skewed. It may be that the selection instrument is not biased but the way in which we are thinking about selection procedures is biased. For example, for judging the quality of universities, there are many different criteria we could use. It could be valuable to examine the various criteria, and you might find what is driving adverse effects.
16.5.3.3 Remove Biased Items
Using item response theory or confirmatory factor analysis, you can identify items that function differently across groups (i.e., differential item functioning/DIF or measurement non-invariance). For instance, you can identify items that show different discrimination/factor loadings or difficulty/intercepts by group. You do not just want to remove items that show mean-level differences in scores (or different rates of endorsement) for one group than another, because there may be true group differences in their level on particular items. If an item is clearly invalid in one group but valid in another group, another option is to keep the item in one group, and to remove it in another group.
Be careful when removing items because removing items can lead to poorer content validity—i.e., items may no longer be a representative set of the content of the construct. Removing items also reduces a measure’s reliability and ability to detect individual differences (Hagquist, 2019; Hagquist & Andrich, 2017). DIF effects tend to be small and inconsistent; removing items showing DIF may not have a big impact.
16.5.3.4 Resolve Biased Items
Another option, for items identified that show differential item functioning using IRT or measurement non-invariance using CFA, is to resolve instead of remove items. Resolving items involves allowing an item to have a different discrimination/factor loading and/or difficulty/intercept parameter for each group. Allowing item parameters to differ across groups has a very small effect on reliability and person separation, so it can be preferable to removing items (Hagquist, 2019; Hagquist & Andrich, 2017).
16.5.3.5 Use Alternative Modes of Testing
Another option is to use alternative modes of testing. For example, you could use audio or video to present test items, rather than requiring a person to read the items, or write answers. Typical testing and computerized exams are oriented toward the upper-middle class, which is therefore a procedure problem! McClelland’s (1973) argument is that we need more real-life testing. Real-life testing could help address stereotype threats and the effects of learning disabilities. However, testing in different modalities could change the construct(s) being assessed.
16.5.3.6 Use Work Records
Using work records is based on McClelland’s (1973) argument to use more realistic and authentic assessments of job-relevant abilities. Evidence on the value of work records for personnel selection is mixed. In some cases, use of work records can actually increase adverse impact on under-represented groups because the primary group typically already has an idea of how to get into the relevant job or is already in the relevant job; therefore, they have a leg up. It would be acceptable to use work records if you trained people first and then tested, but no one spends the time to do this.
16.5.3.7 Increase Time Limit
Another option is to allot people more testing time, as long as doing so does not change the construct. Time limits often lead to greater measurement error because scores conflate pace and quality of work. Increasing time limits requires convincing stakeholders that job performance is typically not “how fast you do things” but “how well you do them”—i.e., that time does not correlate with outcome of interest. The utility of increasing time limits depends on the domain. In some domains, efficiency is crucial (e.g., medicine, pilot). Increasing time limits is not that effective in reducing group differences, and it may actually increase group differences.
16.5.3.8 Use Motivation Sets
Using motivation sets involves finding ways to increase testing motivation for minority groups. It is probably an error to think that a test assesses just aptitude; therefore, we should also consider an individual’s motivation to test. Thus, part of the score has to do with ability and some of the score has to do with motivation. You should try to maximize each examinee’s motivation, so that the person’s score on the measure better captures their true ability score. Motivation sets could include, for example, using more realistic test stimuli that are clearly applicable to the school or job requirements (i.e., that have face validity) to motivate all test takers.
16.5.3.9 Use Instructional Sets
Using instructional sets involves coaching and training. For instance, you could inform examinees about the test content, provide study materials, and recommend test-taking strategies. This could narrow the gap between groups because there is an implicit assumption that the primary group already has “light” training. Using instructional sets aims to reduce error variance due to test anxiety, unfamiliar test format, and poor test-taking skills.
Giving minority groups better access to test preparation is based on the assumption that group differences emerge because of different access to test preparation materials. This could theoretically help to systematically reduce test score differences across groups. Standardized tests like the SAT/GRE/LSAT/GMAT/MCAT, etc. embrace coaching/training. For instance, the organization ETS gives training materials for free. After training, scores on standardized tests show some but minimal improvement. In general, training yields some improvement on quantitative subscales but minimal change on verbal subscales. However, the improvements tend to apply across groups, and they do not seem to lessen group differences in scores.
16.6 Getting Started
16.6.1 Load Libraries
Code
library("petersenlab") #to install: install.packages("remotes"); remotes::install_github("DevPsyLab/petersenlab")
library("lavaan")
library("semTools")
library("semPlot")
library("mirt")
library("dmacs") #to install: install.packages("remotes"); remotes::install_github("ddueber/dmacs")
library("strucchange")
library("MOTE")
library("tidyverse")
library("here")
library("tinytex")
16.6.2 Prepare Data
16.6.2.1 Load Data
cnlsy
is a subset of a data set from the Children of the National Longitudinal Survey of Youth Survey (CNLSY).
The CNLSY is a publicly available longitudinal data set provided by the Bureau of Labor Statistics (https://perma.cc/EH38-HDRN).
The CNLSY data file for these examples is located on the book’s page of the Open Science Framework (https://osf.io/3pwza).
16.6.2.2 Simulate Data
For reproducibility, I set the seed below. Using the same seed will yield the same answer every time. There is nothing special about this particular seed.
Code
sampleSize <- 4000
set.seed(52242)
mydataBias <- data.frame(
ID = 1:sampleSize,
group = factor(c("male","female"),
levels = c("male","female")),
unbiasedPredictor1 = NA,
unbiasedPredictor2 = NA,
unbiasedPredictor3 = NA,
unbiasedCriterion1 = NA,
unbiasedCriterion2 = NA,
unbiasedCriterion3 = NA,
predictor = rnorm(sampleSize, mean = 100, sd = 15),
criterion1 = NA,
criterion2 = NA,
criterion3 = NA,
criterion4 = NA,
criterion5 = NA)
mydataBias$unbiasedPredictor1 <- rnorm(sampleSize, mean = 100, sd = 15)
mydataBias$unbiasedPredictor2[which(mydataBias$group == "male")] <-
rnorm(length(which(mydataBias$group == "male")), mean = 70, sd = 15)
mydataBias$unbiasedPredictor2[which(mydataBias$group == "female")] <-
rnorm(length(which(mydataBias$group == "female")), mean = 130, sd = 15)
mydataBias$unbiasedPredictor3[which(mydataBias$group == "male")] <-
rnorm(length(which(mydataBias$group == "male")), mean = 130, sd = 15)
mydataBias$unbiasedPredictor3[which(mydataBias$group == "female")] <-
rnorm(length(which(mydataBias$group == "female")), mean = 70, sd = 15)
mydataBias$unbiasedCriterion1 <- 1 * mydataBias$unbiasedPredictor1 +
rnorm(sampleSize, mean = 0, sd = 15)
mydataBias$unbiasedCriterion2 <- 1 * mydataBias$unbiasedPredictor2 +
rnorm(sampleSize, mean = 0, sd = 15)
mydataBias$unbiasedCriterion3 <- 1 * mydataBias$unbiasedPredictor3 +
rnorm(sampleSize, mean = 0, sd = 15)
mydataBias$criterion1[which(mydataBias$group == "male")] <-
.7 * mydataBias$predictor[which(mydataBias$group == "male")] +
rnorm(length(which(mydataBias$group == "male")), mean = 0, sd = 5)
mydataBias$criterion1[which(mydataBias$group == "female")] <-
.7 * mydataBias$predictor[which(mydataBias$group == "female")] +
rnorm(length(which(mydataBias$group == "female")), mean = 0, sd = 5)
mydataBias$criterion2[which(mydataBias$group == "male")] <-
.7 * mydataBias$predictor[which(mydataBias$group == "male")] +
rnorm(length(which(mydataBias$group == "male")), mean = 10, sd = 5)
mydataBias$criterion2[which(mydataBias$group == "female")] <-
.7 * mydataBias$predictor[which(mydataBias$group == "female")] +
rnorm(length(which(mydataBias$group == "female")), mean = 0, sd = 5)
mydataBias$criterion3[which(mydataBias$group == "male")] <-
.7 * mydataBias$predictor[which(mydataBias$group == "male")] +
rnorm(length(which(mydataBias$group == "male")), mean = 0, sd = 5)
mydataBias$criterion3[which(mydataBias$group == "female")] <-
.3 * mydataBias$predictor[which(mydataBias$group == "female")] +
rnorm(length(which(mydataBias$group == "female")), mean = 0, sd = 5)
mydataBias$criterion4[which(mydataBias$group == "male")] <-
.7 * mydataBias$predictor[which(mydataBias$group == "male")] +
rnorm(length(which(mydataBias$group == "male")), mean = 0, sd = 5)
mydataBias$criterion4[which(mydataBias$group == "female")] <-
.3 * mydataBias$predictor[which(mydataBias$group == "female")] +
rnorm(length(which(mydataBias$group == "female")), mean = 30, sd = 5)
mydataBias$criterion5[which(mydataBias$group == "male")] <-
.7 * mydataBias$predictor[which(mydataBias$group == "male")] +
rnorm(length(which(mydataBias$group == "male")), mean = 0, sd = 30)
mydataBias$criterion5[which(mydataBias$group == "female")] <-
.7 * mydataBias$predictor[which(mydataBias$group == "female")] +
rnorm(length(which(mydataBias$group == "female")), mean = 0, sd = 5)
16.6.2.3 Add Missing Data
Adding missing data to dataframes helps make examples more realistic to real-life data and helps you get in the habit of programming to account for missing data.
HolzingerSwineford1939
is a data set from the lavaan
package (Rosseel et al., 2022) that contains mental ability test scores (x1
–x9
) for seventh- and eighth-grade children.
Code
varNames <- names(mydataBias)
dimensionsDf <- dim(mydataBias[,-c(1,2)])
unlistedDf <- unlist(mydataBias[,-c(1,2)])
unlistedDf[sample(
1:length(unlistedDf),
size = .01 * length(unlistedDf))] <- NA
mydataBias <- cbind(
mydataBias[,c("ID","group")],
as.data.frame(
matrix(
unlistedDf,
ncol = dimensionsDf[2])))
names(mydataBias) <- varNames
data("HolzingerSwineford1939")
varNames <- names(HolzingerSwineford1939)
dimensionsDf <- dim(HolzingerSwineford1939[,paste("x", 1:9, sep = "")])
unlistedDf <- unlist(HolzingerSwineford1939[,paste("x", 1:9, sep = "")])
unlistedDf[sample(
1:length(unlistedDf),
size = .01 * length(unlistedDf))] <- NA
HolzingerSwineford1939 <- cbind(
HolzingerSwineford1939[,1:6],
as.data.frame(matrix(
unlistedDf,
ncol = dimensionsDf[2])))
names(HolzingerSwineford1939) <- varNames
16.7 Examples of Unbiased Tests (in Terms of Predictive Bias)
16.7.1 Unbiased test where males and females have equal means on predictor and criterion
Figure 16.19 depicts an example of an unbiased test where males and females have equal means on the predictor and criterion.
The test is unbiased because there are no significant differences in the regression lines (of predictor
predicting criterion
) between males and females.
Code
Call:
lm(formula = unbiasedCriterion1 ~ unbiasedPredictor1 + group +
unbiasedPredictor1:group, data = mydataBias)
Residuals:
Min 1Q Median 3Q Max
-54.623 -10.050 -0.025 10.373 66.811
Coefficients:
Estimate Std. Error t value Pr(>|t|)
(Intercept) 0.930697 2.295058 0.406 0.685
unbiasedPredictor1 0.996976 0.022624 44.066 <2e-16 ***
groupfemale -1.165200 3.250481 -0.358 0.720
unbiasedPredictor1:groupfemale -0.004397 0.032115 -0.137 0.891
---
Signif. codes: 0 '***' 0.001 '**' 0.01 '*' 0.05 '.' 0.1 ' ' 1
Residual standard error: 15.13 on 3913 degrees of freedom
(83 observations deleted due to missingness)
Multiple R-squared: 0.4963, Adjusted R-squared: 0.496
F-statistic: 1285 on 3 and 3913 DF, p-value: < 2.2e-16
Code
plot(
unbiasedCriterion1 ~ unbiasedPredictor1,
data = mydataBias,
xlim = c(
0,
max(c(
mydataBias$unbiasedCriterion1,
mydataBias$unbiasedPredictor1),
na.rm = TRUE)),
ylim = c(
0,
max(c(
mydataBias$criterion1,
mydataBias$predictor),
na.rm = TRUE)),
type = "n",
xlab = "predictor",
ylab = "criterion")
points(
mydataBias$unbiasedPredictor1[which(mydataBias$group == "male")],
mydataBias$unbiasedCriterion1[which(mydataBias$group == "male")],
pch = 20,
col = "blue")
points(mydataBias$unbiasedPredictor1[which(mydataBias$group == "female")],
mydataBias$unbiasedCriterion1[which(mydataBias$group == "female")],
pch = 1,
col = "red")
abline(lm(
unbiasedCriterion1 ~ unbiasedPredictor1,
data = mydataBias[which(mydataBias$group == "male"),]),
lty = 1,
col = "blue")
abline(lm(
unbiasedCriterion1 ~ unbiasedPredictor1,
data = mydataBias[which(mydataBias$group == "female"),]),
lty = 2,
col = "red")
legend(
"bottomright",
c("Male","Female"),
lty = c(1,2),
pch = c(20,1),
col = c("blue","red"))
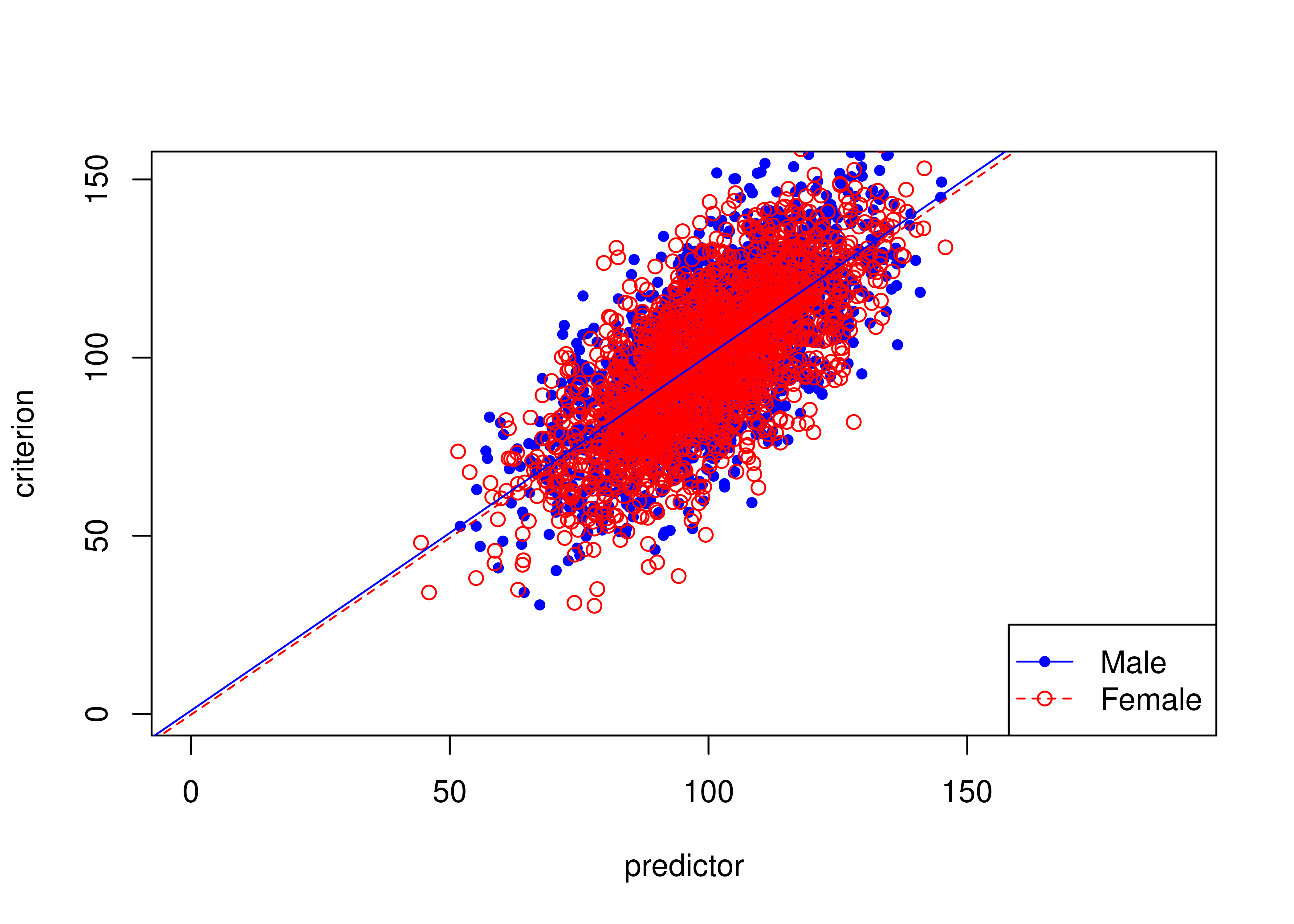
Figure 16.19: Unbiased Test Where Males and Females Have Equal Means on Predictor and Criterion.
16.7.2 Unbiased test where females have higher means than males on predictor and criterion
Figure 16.20 depicts an example of an unbiased test where females have higher means than males on the predictor and criterion.
The test is unbiased because there are no differences in the regression lines (of predictor
predicting criterion
) between males and females.
Code
Call:
lm(formula = unbiasedCriterion2 ~ unbiasedPredictor2 + group +
unbiasedPredictor2:group, data = mydataBias)
Residuals:
Min 1Q Median 3Q Max
-47.302 -9.989 0.010 9.860 53.791
Coefficients:
Estimate Std. Error t value Pr(>|t|)
(Intercept) -1.29332 1.57773 -0.820 0.412
unbiasedPredictor2 1.02006 0.02218 46.000 <2e-16 ***
groupfemale 1.21294 3.28319 0.369 0.712
unbiasedPredictor2:groupfemale -0.01501 0.03125 -0.480 0.631
---
Signif. codes: 0 '***' 0.001 '**' 0.01 '*' 0.05 '.' 0.1 ' ' 1
Residual standard error: 14.81 on 3919 degrees of freedom
(77 observations deleted due to missingness)
Multiple R-squared: 0.8412, Adjusted R-squared: 0.8411
F-statistic: 6921 on 3 and 3919 DF, p-value: < 2.2e-16
Code
plot(
unbiasedCriterion2 ~ unbiasedPredictor2,
data = mydataBias,
xlim = c(
0,
max(c(
mydataBias$unbiasedCriterion2,
mydataBias$unbiasedPredictor2),
na.rm = TRUE)),
ylim = c(
0,
max(c(
mydataBias$criterion1,
mydataBias$predictor),
na.rm = TRUE)),
type = "n",
xlab = "predictor",
ylab = "criterion")
points(
mydataBias$unbiasedPredictor2[which(mydataBias$group == "male")],
mydataBias$unbiasedCriterion2[which(mydataBias$group == "male")],
pch = 20,
col = "blue")
points(
mydataBias$unbiasedPredictor2[which(mydataBias$group == "female")],
mydataBias$unbiasedCriterion2[which(mydataBias$group == "female")],
pch = 1,
col = "red")
abline(lm(
unbiasedCriterion2 ~ unbiasedPredictor2,
data = mydataBias[which(mydataBias$group == "male"),]),
lty = 1,
col = "blue")
abline(lm(
unbiasedCriterion2 ~ unbiasedPredictor2,
data = mydataBias[which(mydataBias$group == "female"),]),
lty = 2,
col = "red")
legend(
"bottomright",
c("Male","Female"),
lty = c(1,2),
pch = c(20,1),
col = c("blue","red"))
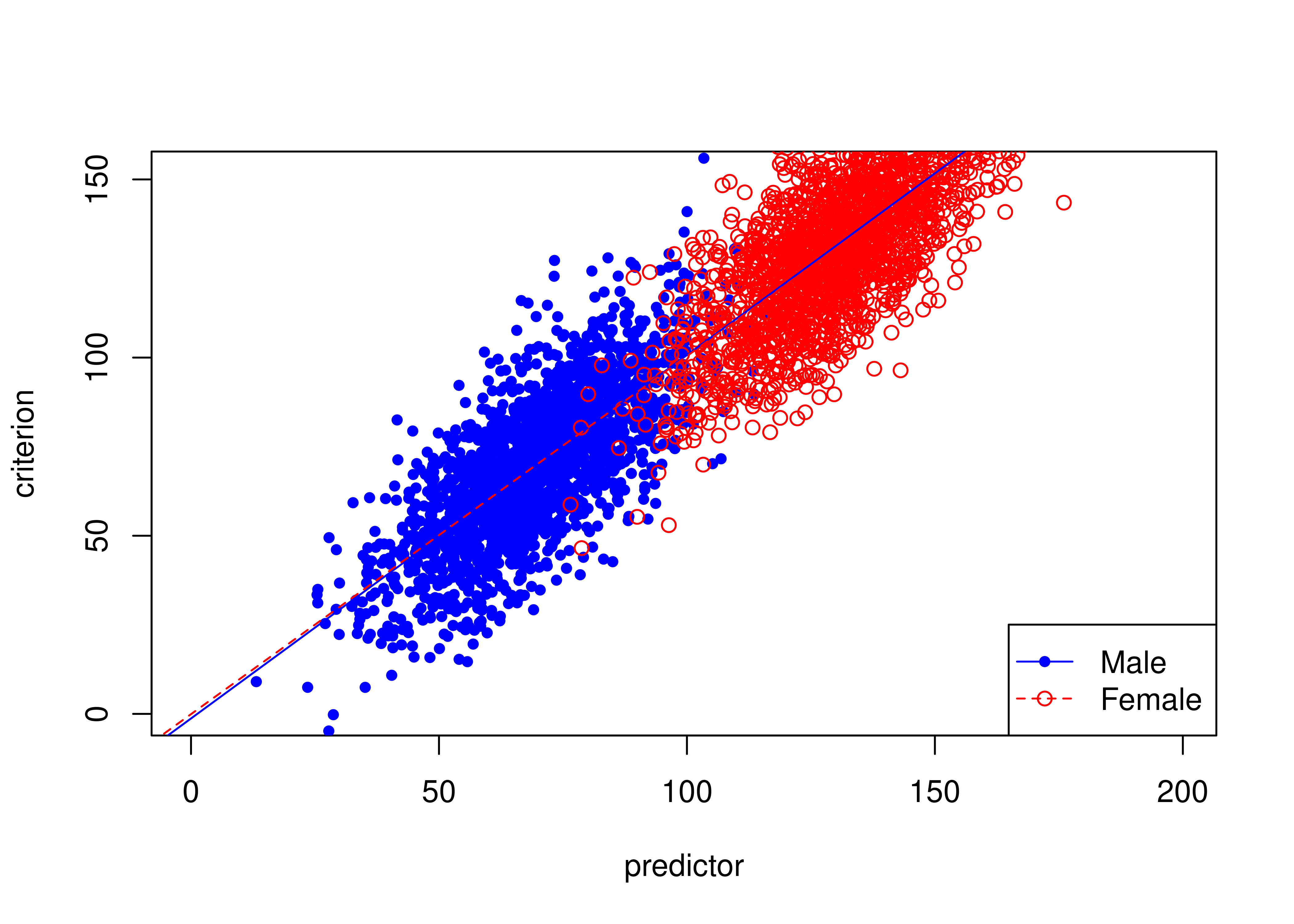
Figure 16.20: Unbiased Test Where Females Have Higher Means Than Males on Predictor and Criterion.
16.7.3 Unbiased test where males have higher means than females on predictor and criterion
Figure 16.21 depicts an example of an unbiased test where males have higher means than females on the predictor and criterion.
The test is unbiased because there are no differences in the regression lines (of predictor
predicting criterion
) between males and females.
Code
Call:
lm(formula = unbiasedCriterion3 ~ unbiasedPredictor3 + group +
unbiasedPredictor3:group, data = mydataBias)
Residuals:
Min 1Q Median 3Q Max
-48.613 -10.115 -0.068 9.598 57.126
Coefficients:
Estimate Std. Error t value Pr(>|t|)
(Intercept) -1.68352 2.84985 -0.591 0.555
unbiasedPredictor3 1.01072 0.02179 46.375 <2e-16 ***
groupfemale 1.42842 3.26227 0.438 0.662
unbiasedPredictor3:groupfemale -0.01187 0.03109 -0.382 0.703
---
Signif. codes: 0 '***' 0.001 '**' 0.01 '*' 0.05 '.' 0.1 ' ' 1
Residual standard error: 14.82 on 3916 degrees of freedom
(80 observations deleted due to missingness)
Multiple R-squared: 0.8376, Adjusted R-squared: 0.8375
F-statistic: 6732 on 3 and 3916 DF, p-value: < 2.2e-16
Code
plot(
unbiasedCriterion3 ~ unbiasedPredictor3,
data = mydataBias,
xlim = c(
0,
max(c(
mydataBias$unbiasedCriterion3,
mydataBias$unbiasedPredictor3),
na.rm = TRUE)),
ylim = c(0, max(c(
mydataBias$criterion1,
mydataBias$predictor),
na.rm = TRUE)),
type = "n",
xlab = "predictor",
ylab = "criterion")
points(
mydataBias$unbiasedPredictor3[which(mydataBias$group == "male")],
mydataBias$unbiasedCriterion3[which(mydataBias$group == "male")],
pch = 20,
col = "blue")
points(
mydataBias$unbiasedPredictor3[which(mydataBias$group == "female")],
mydataBias$unbiasedCriterion3[which(mydataBias$group == "female")],
pch = 1,
col = "red")
abline(lm(
unbiasedCriterion3 ~ unbiasedPredictor3,
data = mydataBias[which(mydataBias$group == "male"),]),
lty = 1,
col = "blue")
abline(lm(
unbiasedCriterion3 ~ unbiasedPredictor3,
data = mydataBias[which(mydataBias$group == "female"),]),
lty = 2,
col = "red")
legend(
"bottomright",
c("Male","Female"),
lty = c(1,2),
pch = c(20,1),
col = c("blue","red"))
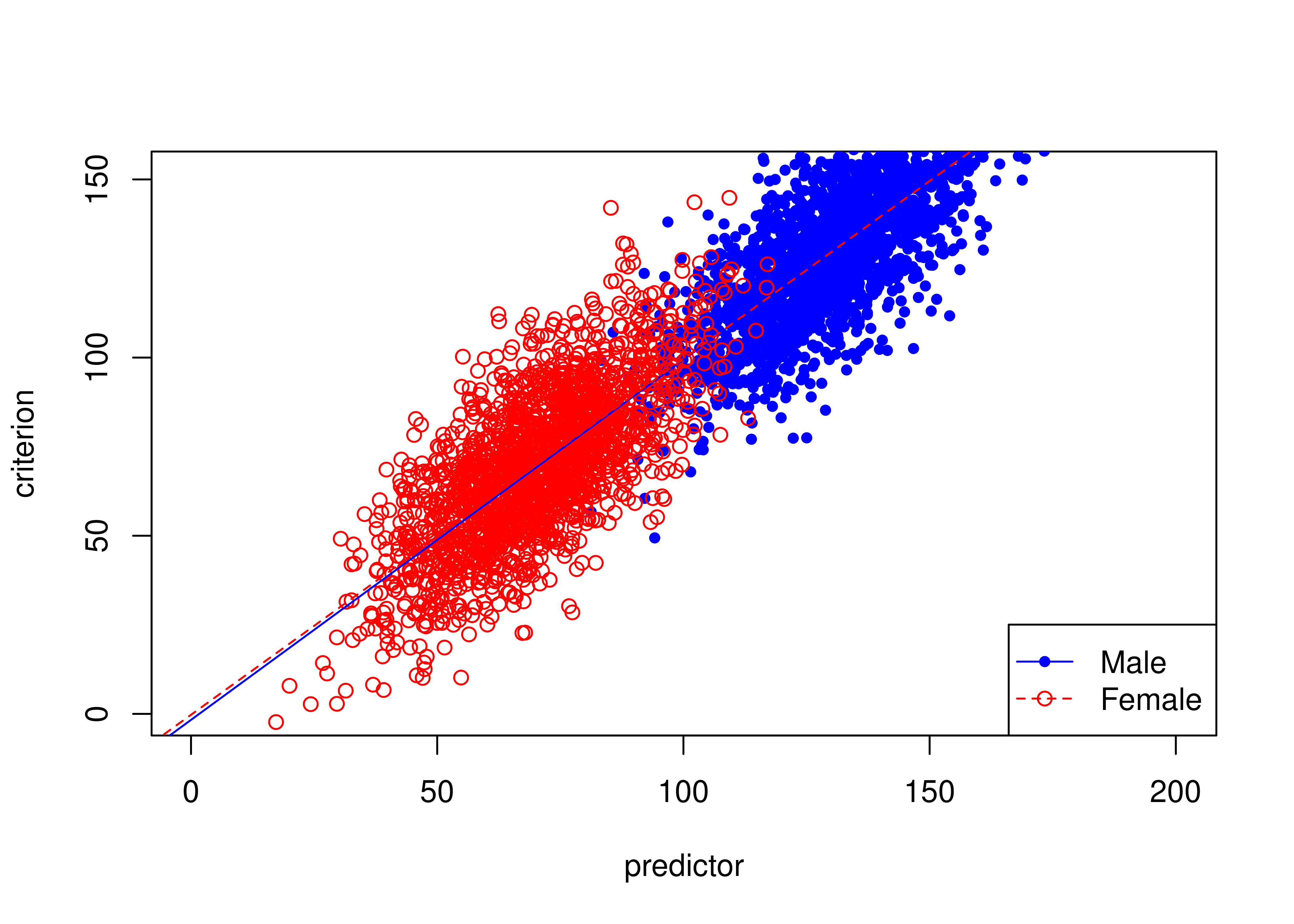
Figure 16.21: Unbiased Test Where Males Have Higher Means Than Females on Predictor and Criterion.
16.8 Predictive Bias: Different Regression Lines
16.8.1 Example of unbiased prediction (no differences in intercepts or slopes)
Figure 16.22 depicts an example of an unbiased test where males and females have equal means on the predictor and criterion.
The test is unbiased because there are no differences in the regression lines (of predictor
predicting criterion1
) between males and females.
Call:
lm(formula = criterion1 ~ predictor + group + predictor:group,
data = mydataBias)
Residuals:
Min 1Q Median 3Q Max
-18.831 -3.338 0.004 3.330 19.335
Coefficients:
Estimate Std. Error t value Pr(>|t|)
(Intercept) -0.264357 0.747269 -0.354 0.724
predictor 0.701726 0.007370 95.207 <2e-16 ***
groupfemale -0.515860 1.059267 -0.487 0.626
predictor:groupfemale 0.006002 0.010476 0.573 0.567
---
Signif. codes: 0 '***' 0.001 '**' 0.01 '*' 0.05 '.' 0.1 ' ' 1
Residual standard error: 4.952 on 3908 degrees of freedom
(88 observations deleted due to missingness)
Multiple R-squared: 0.8225, Adjusted R-squared: 0.8223
F-statistic: 6035 on 3 and 3908 DF, p-value: < 2.2e-16
Code
plot(
criterion1 ~ predictor,
data = mydataBias,
xlim = c(
0,
max(c(
mydataBias$criterion1,
mydataBias$predictor),
na.rm = TRUE)),
ylim = c(
0,
max(c(
mydataBias$criterion1,
mydataBias$predictor),
na.rm = TRUE)),
type = "n",
xlab = "predictor",
ylab = "criterion")
points(
mydataBias$predictor[which(mydataBias$group == "male")],
mydataBias$criterion1[which(mydataBias$group == "male")],
pch = 20,
col = "blue")
points(
mydataBias$predictor[which(mydataBias$group == "female")],
mydataBias$criterion1[which(mydataBias$group == "female")],
pch = 1,
col = "red")
abline(lm(
criterion1 ~ predictor,
data = mydataBias[which(mydataBias$group == "male"),]),
lty = 1,
col = "blue")
abline(lm(
criterion1 ~ predictor,
data = mydataBias[which(mydataBias$group == "female"),]),
lty = 2,
col = "red")
legend(
"bottomright",
c("Male","Female"),
lty = c(1,2),
pch = c(20,1),
col = c("blue","red"))
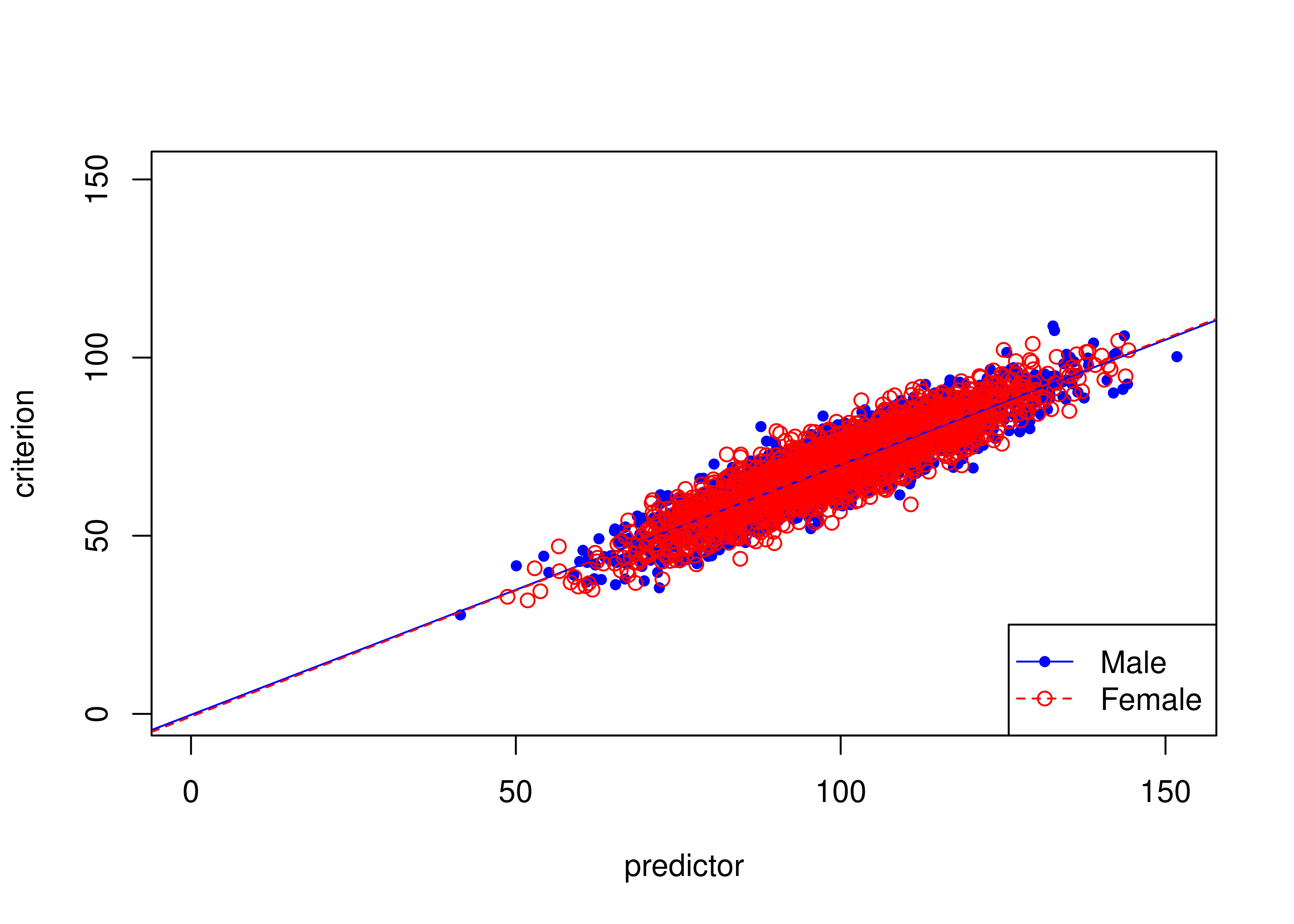
Figure 16.22: Example of Unbiased Prediction (No Differences in Intercepts or Slopes Between Males and Females).
16.8.2 Example of intercept bias
Figure 16.23 depicts an example of a biased test due to intercept bias.
There are differences in the intercepts of the regression lines (of predictor
predicting criterion2
) between males and females: males have a higher intercept than females.
That is, the same score on the predictor results in higher predictions for males than females.
Call:
lm(formula = criterion2 ~ predictor + group + predictor:group,
data = mydataBias)
Residuals:
Min 1Q Median 3Q Max
-18.6853 -3.4153 -0.0385 3.3979 18.0864
Coefficients:
Estimate Std. Error t value Pr(>|t|)
(Intercept) 10.054048 0.760084 13.228 <2e-16 ***
predictor 0.697987 0.007499 93.075 <2e-16 ***
groupfemale -10.288893 1.074168 -9.578 <2e-16 ***
predictor:groupfemale 0.004748 0.010625 0.447 0.655
---
Signif. codes: 0 '***' 0.001 '**' 0.01 '*' 0.05 '.' 0.1 ' ' 1
Residual standard error: 5.037 on 3913 degrees of freedom
(83 observations deleted due to missingness)
Multiple R-squared: 0.8452, Adjusted R-squared: 0.8451
F-statistic: 7124 on 3 and 3913 DF, p-value: < 2.2e-16
Code
plot(
criterion2 ~ predictor,
data = mydataBias,
xlim = c(
0,
max(c(
mydataBias$criterion2,
mydataBias$predictor), na.rm = TRUE)),
ylim = c(
0,
max(c(
mydataBias$criterion2,
mydataBias$predictor),
na.rm = TRUE)),
type = "n",
xlab = "predictor",
ylab = "criterion")
points(
mydataBias$predictor[which(mydataBias$group == "male")],
mydataBias$criterion2[which(mydataBias$group == "male")],
pch = 20,
col = "blue")
points(
mydataBias$predictor[which(mydataBias$group == "female")],
mydataBias$criterion2[which(mydataBias$group == "female")],
pch = 1,
col = "red")
abline(lm(
criterion2 ~ predictor,
data = mydataBias[which(mydataBias$group == "male"),]),
lty = 1,
col = "blue")
abline(lm(
criterion2 ~ predictor,
data = mydataBias[which(mydataBias$group == "female"),]),
lty = 2,
col = "red")
legend(
"bottomright",
c("Male","Female"),
lty = c(1,2),
pch = c(20,1),
col = c("blue","red"))
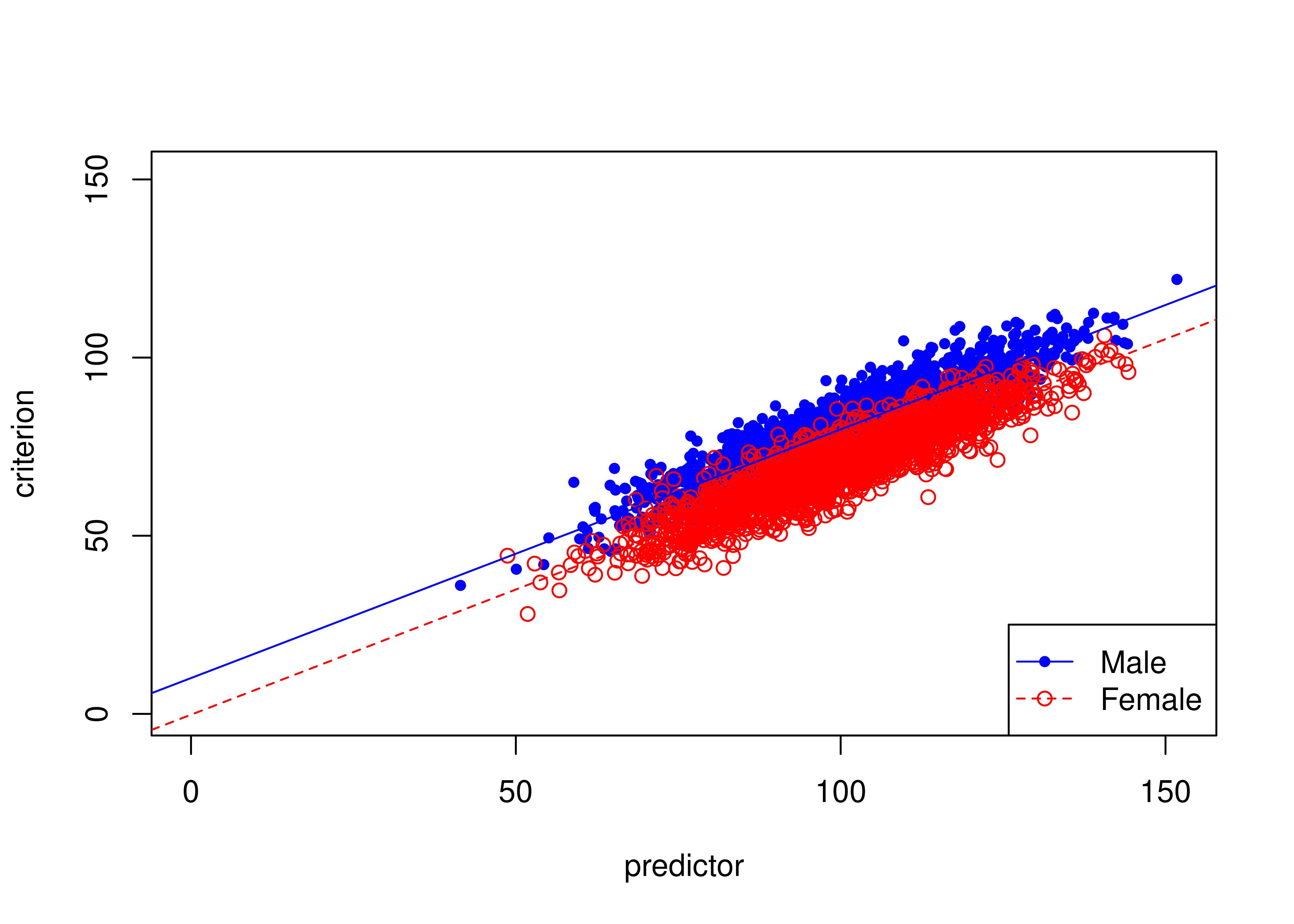
Figure 16.23: Example of Intercept Bias in Prediction (Different Intercepts Between Males and Females).
16.8.3 Example of slope bias
Figure 16.24 depicts an example of a biased test due to slope bias.
There are differences in the slopes of the regression lines (of predictor
predicting criterion3
) between males and females: males have a higher slope than females.
That is, scores have stronger predictive validity for males than females.
Call:
lm(formula = criterion3 ~ predictor + group + predictor:group,
data = mydataBias)
Residuals:
Min 1Q Median 3Q Max
-18.3183 -3.3715 0.0256 3.3844 19.3484
Coefficients:
Estimate Std. Error t value Pr(>|t|)
(Intercept) -1.041940 0.757914 -1.375 0.169
predictor 0.708670 0.007475 94.804 <2e-16 ***
groupfemale 1.478534 1.074064 1.377 0.169
predictor:groupfemale -0.413233 0.010621 -38.907 <2e-16 ***
---
Signif. codes: 0 '***' 0.001 '**' 0.01 '*' 0.05 '.' 0.1 ' ' 1
Residual standard error: 5.031 on 3927 degrees of freedom
(69 observations deleted due to missingness)
Multiple R-squared: 0.9489, Adjusted R-squared: 0.9489
F-statistic: 2.432e+04 on 3 and 3927 DF, p-value: < 2.2e-16
Code
plot(
criterion3 ~ predictor,
data = mydataBias,
xlim = c(
0,
max(c(
mydataBias$criterion3,
mydataBias$predictor),
na.rm = TRUE)),
ylim = c(
0,
max(c(
mydataBias$criterion3,
mydataBias$predictor),
na.rm = TRUE)),
type = "n",
xlab = "predictor",
ylab = "criterion")
points(
mydataBias$predictor[which(mydataBias$group == "male")],
mydataBias$criterion3[which(mydataBias$group == "male")],
pch = 20,
col = "blue")
points(
mydataBias$predictor[which(mydataBias$group == "female")],
mydataBias$criterion3[which(mydataBias$group == "female")],
pch = 1,
col = "red")
abline(lm(
criterion3 ~ predictor,
data = mydataBias[which(mydataBias$group == "male"),]),
lty = 1,
col = "blue")
abline(lm(
criterion3 ~ predictor,
data = mydataBias[which(mydataBias$group == "female"),]),
lty = 2,
col = "red")
legend(
"bottomright",
c("Male","Female"),
lty = c(1,2),
pch = c(20,1),
col = c("blue","red"))
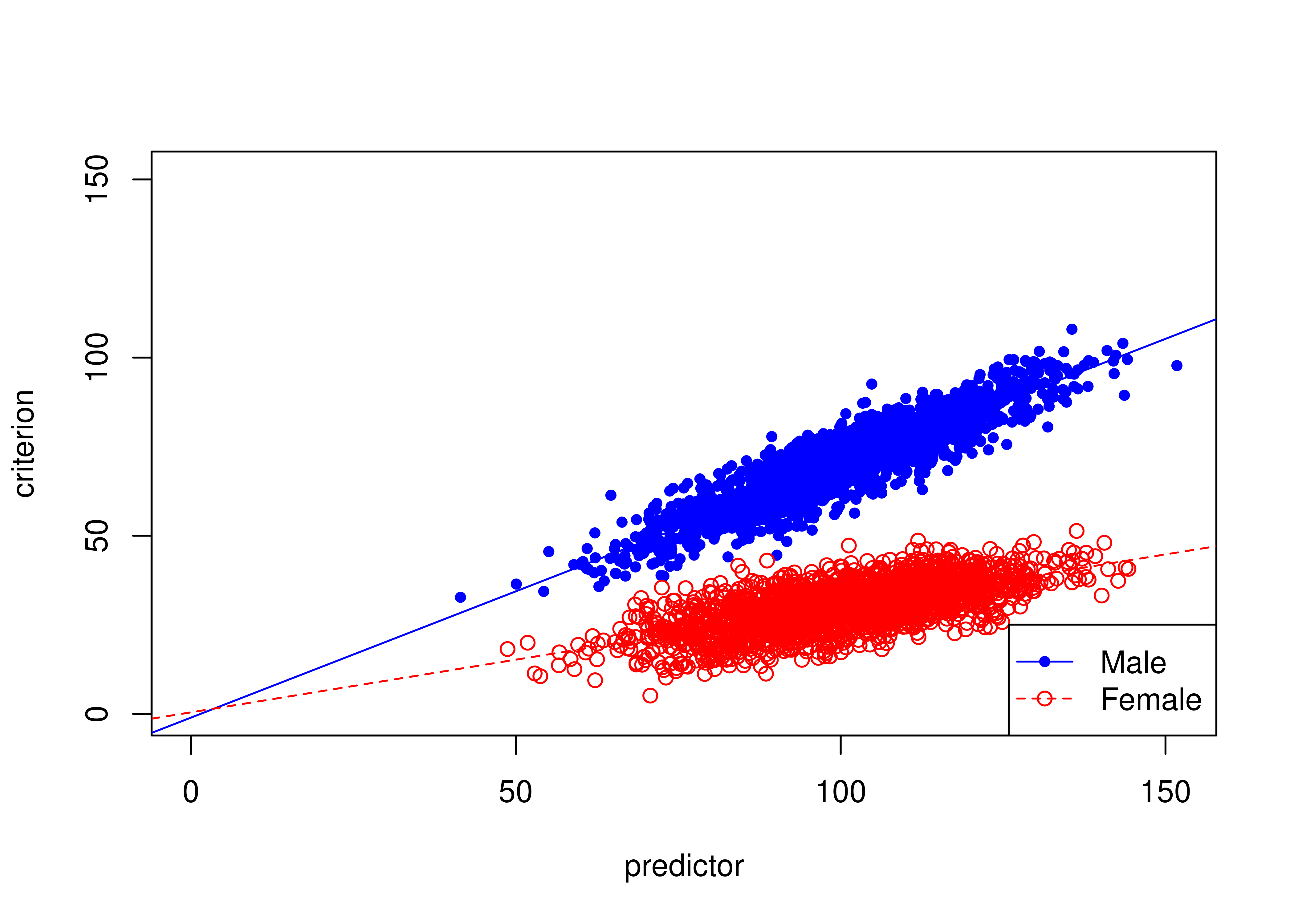
Figure 16.24: Example of Slope Bias in Prediction (Different Slopes Between Males And Females).
16.8.4 Example of intercept and slope bias
Figure 16.25 depicts an example of a biased test due to intercept and slope bias.
There are differences in the intercepts and slopes of the regression lines (of predictor
predicting criterion4
) between males and females: males have a higher slope than females.
That is, scores have stronger predictive validity for males than females.
Females have a higher intercept than males.
That is, at lower scores, the same score on the predictor results in higher predictions for females than males; at higher scores on the predictor, the same score results in higher predictions for males than females.
Call:
lm(formula = criterion4 ~ predictor + group + predictor:group,
data = mydataBias)
Residuals:
Min 1Q Median 3Q Max
-18.2805 -3.4400 0.0269 3.3199 17.8824
Coefficients:
Estimate Std. Error t value Pr(>|t|)
(Intercept) -0.276228 0.767080 -0.36 0.719
predictor 0.702178 0.007565 92.81 <2e-16 ***
groupfemale 29.156167 1.086254 26.84 <2e-16 ***
predictor:groupfemale -0.392016 0.010743 -36.49 <2e-16 ***
---
Signif. codes: 0 '***' 0.001 '**' 0.01 '*' 0.05 '.' 0.1 ' ' 1
Residual standard error: 5.099 on 3930 degrees of freedom
(66 observations deleted due to missingness)
Multiple R-squared: 0.7843, Adjusted R-squared: 0.7842
F-statistic: 4764 on 3 and 3930 DF, p-value: < 2.2e-16
Code
plot(
criterion4 ~ predictor,
data = mydataBias,
xlim = c(
0,
max(c(
mydataBias$criterion4,
mydataBias$predictor),
na.rm = TRUE)),
ylim = c(
0,
max(c(
mydataBias$criterion4,
mydataBias$predictor),
na.rm = TRUE)),
type = "n",
xlab = "predictor",
ylab = "criterion")
points(
mydataBias$predictor[which(mydataBias$group == "male")],
mydataBias$criterion4[which(mydataBias$group == "male")],
pch = 20,
col = "blue")
points(
mydataBias$predictor[which(mydataBias$group == "female")],
mydataBias$criterion4[which(mydataBias$group == "female")],
pch = 1,
col = "red")
abline(lm(
criterion4 ~ predictor,
data = mydataBias[which(mydataBias$group == "male"),]),
lty = 1,
col = "blue")
abline(lm(
criterion4 ~ predictor,
data = mydataBias[which(mydataBias$group == "female"),]),
lty = 2,
col = "red")
legend(
"bottomright",
c("Male","Female"),
lty = c(1,2),
pch = c(20,1),
col = c("blue","red"))
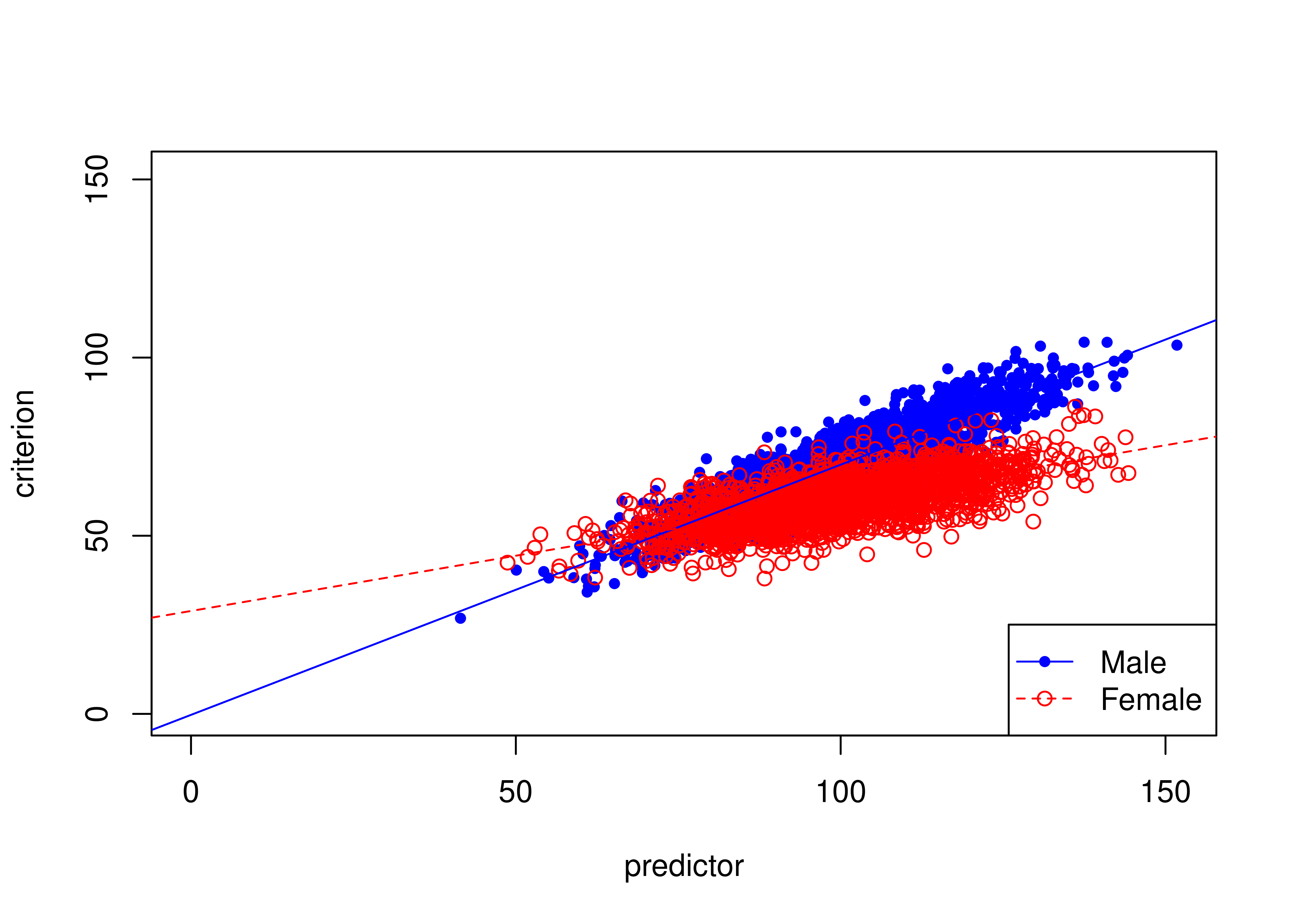
Figure 16.25: Example of Intercept And Slope Bias in Prediction (Different Intercepts and Slopes Between Males and Females).
16.8.5 Example of different measurement reliability/error across groups
In the example depicted in Figure 16.26, there are differences in the measurement reliability/error on the criterion between males and females: males’ scores have a lower reliability (higher measurement error) on the criterion than females’ scores. That is, we are more confident about a female’s level on the criterion given a particular score on the predictor than we are about a male’s level on the criterion given a particular score on the predictor.
Call:
lm(formula = criterion5 ~ predictor + group + predictor:group,
data = mydataBias)
Residuals:
Min 1Q Median 3Q Max
-109.295 -7.065 0.077 6.836 108.428
Coefficients:
Estimate Std. Error t value Pr(>|t|)
(Intercept) -9.28386 3.32617 -2.791 0.00528 **
predictor 0.79172 0.03280 24.136 < 2e-16 ***
groupfemale 9.12452 4.70470 1.939 0.05252 .
predictor:groupfemale -0.09083 0.04654 -1.952 0.05102 .
---
Signif. codes: 0 '***' 0.001 '**' 0.01 '*' 0.05 '.' 0.1 ' ' 1
Residual standard error: 22.03 on 3920 degrees of freedom
(76 observations deleted due to missingness)
Multiple R-squared: 0.2087, Adjusted R-squared: 0.2081
F-statistic: 344.6 on 3 and 3920 DF, p-value: < 2.2e-16
Code
plot(
criterion5 ~ predictor,
data = mydataBias,
xlim = c(
0,
max(c(mydataBias$criterion5,
mydataBias$predictor),
na.rm = TRUE)),
ylim = c(
0,
max(c(
mydataBias$criterion5,
mydataBias$predictor),
na.rm = TRUE)),
type = "n",
xlab = "predictor",
ylab = "criterion")
points(
mydataBias$predictor[which(mydataBias$group == "male")],
mydataBias$criterion5[which(mydataBias$group == "male")],
pch = 20,
col = "blue")
points(mydataBias$predictor[which(mydataBias$group == "female")],
mydataBias$criterion5[which(mydataBias$group == "female")],
pch = 1,
col = "red")
abline(lm(criterion5 ~ predictor,
data = mydataBias[which(mydataBias$group == "male"),]),
lty = 1,
col = "blue")
abline(lm(criterion5 ~ predictor,
data = mydataBias[which(mydataBias$group == "female"),]),
lty = 2,
col = "red")
legend(
"bottomright",
c("Male","Female"),
lty = c(1,2),
pch = c(20,1),
col = c("blue","red"))
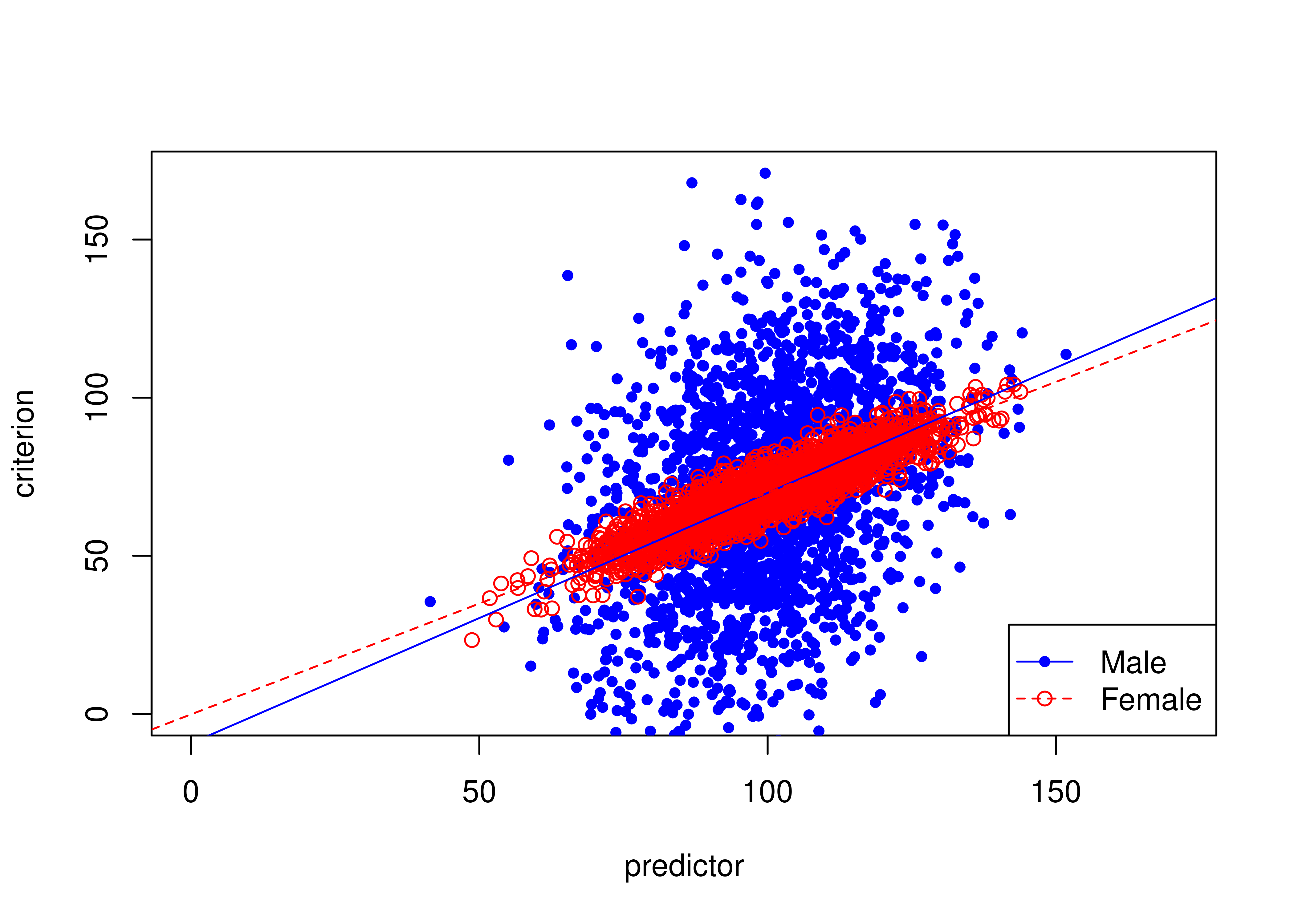
Figure 16.26: Example of Different Measurement Reliability/Error Across Groups.
16.9 Differential Item Functioning (DIF)
Differential item functioning (DIF) indicates that one or more items functions differently across groups. That is, one or more of the item parameters for an item differs across groups. For instance, the severity or discrimination of an item could be higher in one group compared to another group. If an item functions differently across groups, it can lead to biased scores for particular groups. Thus, when observing group differences in level on the measure or group differences in the measure’s association with other measures, it is unclear whether the observed group differences reflect true group differences or differences in the functioning of the measure across groups.
For instance, consider an item such as “disobedience to authority”, which is thought to reflect externalizing behavior in childhood. However, in adulthood, disobedience to authority could reflect prosocial functions, such as protesting against societally unjust actions, and may show weaker construct validity with respect to externalizing problems, as operationalized by a weaker discrimination coefficient in adulthood than in childhood. Tests of differential item functioning are equivalent to tests of measurement invariance.
Approaches to addressing DIF are described in Section 16.5.1. If we identify that an item shows non-negligible DIF, we have three primary options (described above): (1) drop the item for both groups, (2) drop the item for one group but keep it for the other group, or (3) freely estimate the parameters (discrimination and difficulty) for the item across groups.
Tests of DIF were conducted using the mirt
package (Chalmers, 2020).
16.9.1 Item Descriptive Statistics
Code
$female
$female$overall
N.complete N mean_total.score sd_total.score ave.r sd.r alpha SEM.alpha
1620 5641 2.554 2.132 0.255 0.083 0.681 1.203
$female$itemstats
N mean sd total.r total.r_if_rm alpha_if_rm
bpi_antisocialT1_1 1928 0.538 0.587 0.662 0.456 0.626
bpi_antisocialT1_2 1925 0.278 0.510 0.648 0.471 0.624
bpi_antisocialT1_3 1925 0.456 0.654 0.625 0.376 0.657
bpi_antisocialT1_4 1933 0.113 0.355 0.523 0.384 0.654
bpi_antisocialT1_5 1649 0.186 0.431 0.594 0.438 0.637
bpi_antisocialT1_6 1658 0.103 0.345 0.572 0.446 0.643
bpi_antisocialT1_7 1944 0.834 0.639 0.553 0.292 0.683
$female$proportions
0 1 2 NA
bpi_antisocialT1_1 0.174 0.151 0.016 0.658
bpi_antisocialT1_2 0.257 0.075 0.010 0.659
bpi_antisocialT1_3 0.216 0.094 0.031 0.659
bpi_antisocialT1_4 0.308 0.030 0.004 0.657
bpi_antisocialT1_5 0.243 0.044 0.005 0.708
bpi_antisocialT1_6 0.268 0.023 0.004 0.706
bpi_antisocialT1_7 0.104 0.194 0.046 0.655
$female$total.score_frequency
0 1 2 3 4 5 6 7 8 9 10 11 12 13
Freq 217 364 349 283 166 91 67 38 12 10 11 4 4 4
$female$total.score_means
0 1 2
bpi_antisocialT1_1 1.354919 3.339237 7.216867
bpi_antisocialT1_2 1.846343 4.173789 8.192308
bpi_antisocialT1_3 1.593417 3.846682 5.406667
bpi_antisocialT1_4 2.204952 5.090278 9.045455
bpi_antisocialT1_5 2.010386 4.946721 7.892857
bpi_antisocialT1_6 2.189959 5.701613 9.227273
bpi_antisocialT1_7 1.004357 2.778970 4.746725
$female$total.score_sds
0 1 2
bpi_antisocialT1_1 1.262270 1.668474 2.763209
bpi_antisocialT1_2 1.480206 1.809814 2.664498
bpi_antisocialT1_3 1.329902 1.837884 2.783211
bpi_antisocialT1_4 1.743521 2.280698 2.802673
bpi_antisocialT1_5 1.566126 2.196431 3.258485
bpi_antisocialT1_6 1.684322 2.215906 2.844072
bpi_antisocialT1_7 1.232733 1.798814 2.475591
$male
$male$overall
N.complete N mean_total.score sd_total.score ave.r sd.r alpha SEM.alpha
1645 5891 3.16 2.385 0.266 0.081 0.703 1.3
$male$itemstats
N mean sd total.r total.r_if_rm alpha_if_rm
bpi_antisocialT1_1 1948 0.615 0.585 0.634 0.449 0.660
bpi_antisocialT1_2 1947 0.372 0.559 0.655 0.485 0.650
bpi_antisocialT1_3 1949 0.520 0.668 0.582 0.346 0.692
bpi_antisocialT1_4 1948 0.233 0.486 0.596 0.441 0.666
bpi_antisocialT1_5 1676 0.367 0.547 0.627 0.454 0.662
bpi_antisocialT1_6 1688 0.177 0.446 0.556 0.406 0.675
bpi_antisocialT1_7 1962 0.834 0.646 0.582 0.357 0.687
$male$proportions
0 1 2 NA
bpi_antisocialT1_1 0.145 0.168 0.017 0.669
bpi_antisocialT1_2 0.220 0.097 0.013 0.669
bpi_antisocialT1_3 0.191 0.107 0.033 0.669
bpi_antisocialT1_4 0.263 0.058 0.010 0.669
bpi_antisocialT1_5 0.190 0.085 0.010 0.715
bpi_antisocialT1_6 0.243 0.035 0.008 0.713
bpi_antisocialT1_7 0.102 0.185 0.046 0.667
$male$total.score_frequency
0 1 2 3 4 5 6 7 8 9 10 11 12 13 14
Freq 163 294 310 259 208 144 104 74 40 25 9 7 5 1 2
$male$total.score_means
0 1 2
bpi_antisocialT1_1 1.622478 3.944056 7.397849
bpi_antisocialT1_2 2.134191 4.767821 8.106061
bpi_antisocialT1_3 2.016754 4.400763 5.819277
bpi_antisocialT1_4 2.484091 5.539286 8.177778
bpi_antisocialT1_5 2.174111 4.817073 7.910714
bpi_antisocialT1_6 2.616762 5.810680 8.093023
bpi_antisocialT1_7 1.412500 3.375679 5.782787
$male$total.score_sds
0 1 2
bpi_antisocialT1_1 1.515237 1.982218 2.454542
bpi_antisocialT1_2 1.678482 1.955386 2.437709
bpi_antisocialT1_3 1.654589 2.069463 2.774973
bpi_antisocialT1_4 1.845772 2.119687 2.534210
bpi_antisocialT1_5 1.709715 2.047072 2.725481
bpi_antisocialT1_6 1.911288 2.181537 2.982600
bpi_antisocialT1_7 1.522500 1.906515 2.652344
16.9.2 Unconstrained Model
16.9.2.2 Model Summary
Items that appear to differ:
- items 5 and 6 are more discriminating (\(a\) parameter) among females than males
- item 4 has higher difficulty/severity (\(b_1\) and \(b_2\) parameters) among males than females
----------
GROUP: female
F1 h2
bpi_antisocialT1_1 0.698 0.487
bpi_antisocialT1_2 0.706 0.499
bpi_antisocialT1_3 0.532 0.283
bpi_antisocialT1_4 0.683 0.466
bpi_antisocialT1_5 0.763 0.582
bpi_antisocialT1_6 0.838 0.702
bpi_antisocialT1_7 0.429 0.184
SS loadings: 3.202
Proportion Var: 0.457
Factor correlations:
F1
F1 1
----------
GROUP: male
F1 h2
bpi_antisocialT1_1 0.663 0.440
bpi_antisocialT1_2 0.736 0.542
bpi_antisocialT1_3 0.531 0.282
bpi_antisocialT1_4 0.713 0.508
bpi_antisocialT1_5 0.691 0.477
bpi_antisocialT1_6 0.702 0.492
bpi_antisocialT1_7 0.507 0.257
SS loadings: 2.999
Proportion Var: 0.428
Factor correlations:
F1
F1 1
$female
$items
a b1 b2
bpi_antisocialT1_1 1.657 0.029 2.451
bpi_antisocialT1_2 1.697 0.953 2.763
bpi_antisocialT1_3 1.069 0.650 2.585
bpi_antisocialT1_4 1.590 1.895 3.465
bpi_antisocialT1_5 2.008 1.281 2.936
bpi_antisocialT1_6 2.614 1.625 2.770
bpi_antisocialT1_7 0.807 -1.184 2.583
$means
F1
0
$cov
F1
F1 1
$male
$items
a b1 b2
bpi_antisocialT1_1 1.507 -0.238 2.505
bpi_antisocialT1_2 1.853 0.582 2.455
bpi_antisocialT1_3 1.067 0.384 2.480
bpi_antisocialT1_4 1.729 1.165 2.765
bpi_antisocialT1_5 1.625 0.635 2.757
bpi_antisocialT1_6 1.677 1.494 2.873
bpi_antisocialT1_7 1.002 -0.986 2.143
$means
F1
0
$cov
F1
F1 1
16.9.3 Constrained Model
Constrain item parameters to be equal across groups (to use as baseline model for identifying DIF).
16.9.3.1 Fit Model
Code
constrainedModel <- multipleGroup(
data = cnlsy[,c(
"bpi_antisocialT1_1","bpi_antisocialT1_2","bpi_antisocialT1_3",
"bpi_antisocialT1_4","bpi_antisocialT1_5","bpi_antisocialT1_6",
"bpi_antisocialT1_7")],
model = 1,
group = cnlsy$sex,
invariance = c(c(
"bpi_antisocialT1_1","bpi_antisocialT1_2","bpi_antisocialT1_3",
"bpi_antisocialT1_4","bpi_antisocialT1_5","bpi_antisocialT1_6",
"bpi_antisocialT1_7"),
"free_means", "free_var"),
SE = TRUE)
16.9.3.2 Model Summary
----------
GROUP: female
F1 h2
bpi_antisocialT1_1 0.657 0.431
bpi_antisocialT1_2 0.707 0.500
bpi_antisocialT1_3 0.512 0.262
bpi_antisocialT1_4 0.701 0.491
bpi_antisocialT1_5 0.733 0.538
bpi_antisocialT1_6 0.765 0.585
bpi_antisocialT1_7 0.440 0.193
SS loadings: 3
Proportion Var: 0.429
Factor correlations:
F1
F1 1
----------
GROUP: male
F1 h2
bpi_antisocialT1_1 0.672 0.452
bpi_antisocialT1_2 0.722 0.521
bpi_antisocialT1_3 0.527 0.278
bpi_antisocialT1_4 0.716 0.512
bpi_antisocialT1_5 0.747 0.559
bpi_antisocialT1_6 0.778 0.605
bpi_antisocialT1_7 0.455 0.207
SS loadings: 3.134
Proportion Var: 0.448
Factor correlations:
F1
F1 1
$female
$items
a b1 b2
bpi_antisocialT1_1 1.482 0.078 2.798
bpi_antisocialT1_2 1.701 0.973 2.892
bpi_antisocialT1_3 1.013 0.726 2.844
bpi_antisocialT1_4 1.673 1.688 3.278
bpi_antisocialT1_5 1.836 1.135 3.028
bpi_antisocialT1_6 2.020 1.782 3.048
bpi_antisocialT1_7 0.834 -0.967 2.697
$means
F1
0
$cov
F1
F1 1
$male
$items
a b1 b2
bpi_antisocialT1_1 1.482 0.078 2.798
bpi_antisocialT1_2 1.701 0.973 2.892
bpi_antisocialT1_3 1.013 0.726 2.844
bpi_antisocialT1_4 1.673 1.688 3.278
bpi_antisocialT1_5 1.836 1.135 3.028
bpi_antisocialT1_6 2.020 1.782 3.048
bpi_antisocialT1_7 0.834 -0.967 2.697
$means
F1
0.37
$cov
F1
F1 1.088
16.9.4 Compare model fit of constrained model to unconstrained model
The constrained model and the unconstrained model are considered “nested” models. The constrained model is nested within the unconstrained model because the unconstrained model includes all of the terms of the constrained model along with additional terms. Model fit of nested models can be compared with a chi-square difference test.
The constrained model fits significantly worse than the unconstrained model, which suggests that item parameters differ between males and females.
16.9.5 Identify DIF by iteratively removing constraints from fully constrained model
One way to identify DIF is to iteratively remove constraints from a model in which all parameters are constrained to be the same across groups. Removing constraints allows individual items to have different item parameters across groups, to identify which items yield a significant improvement in model fit when allowing their discrimination and/or severity to differ across groups. Items 1, 3, 4, 5, 6, and 7 showed DIF in discrimination and/or severity, based on a significant chi-square difference test.
16.9.5.2 Items that differ in discrimination
Items 1, 3, 4, and 5 showed DIF in discrimination, based on a significant chi-square difference test.
Code
16.9.5.3 Items that differ in severity
Items 1, 3, 4, 5, and 7 showed DIF in difficulty/severity, based on a significant chi-square difference test.
Code
16.9.6 Identify DIF by iteratively adding constraints to unconstrained model
16.9.6.1 Items that differ in discrimination and/or severity
Another way to identify DIF is to iteratively add constraints to a model in which all parameters are allowed to differ across groups. Adding constraints forces individual items to have the same item parameters across groups, to identify which items yield a significant worsening in model fit when constraining their discrimination and/or severity to be the same across groups. Items 1, 2, 3, 4, 5, and 6 showed DIF in discrimination and/or severity, based on a significant chi-square difference test. The DIF in discrimination and/or severity is depicted in Figure 16.27.
Code
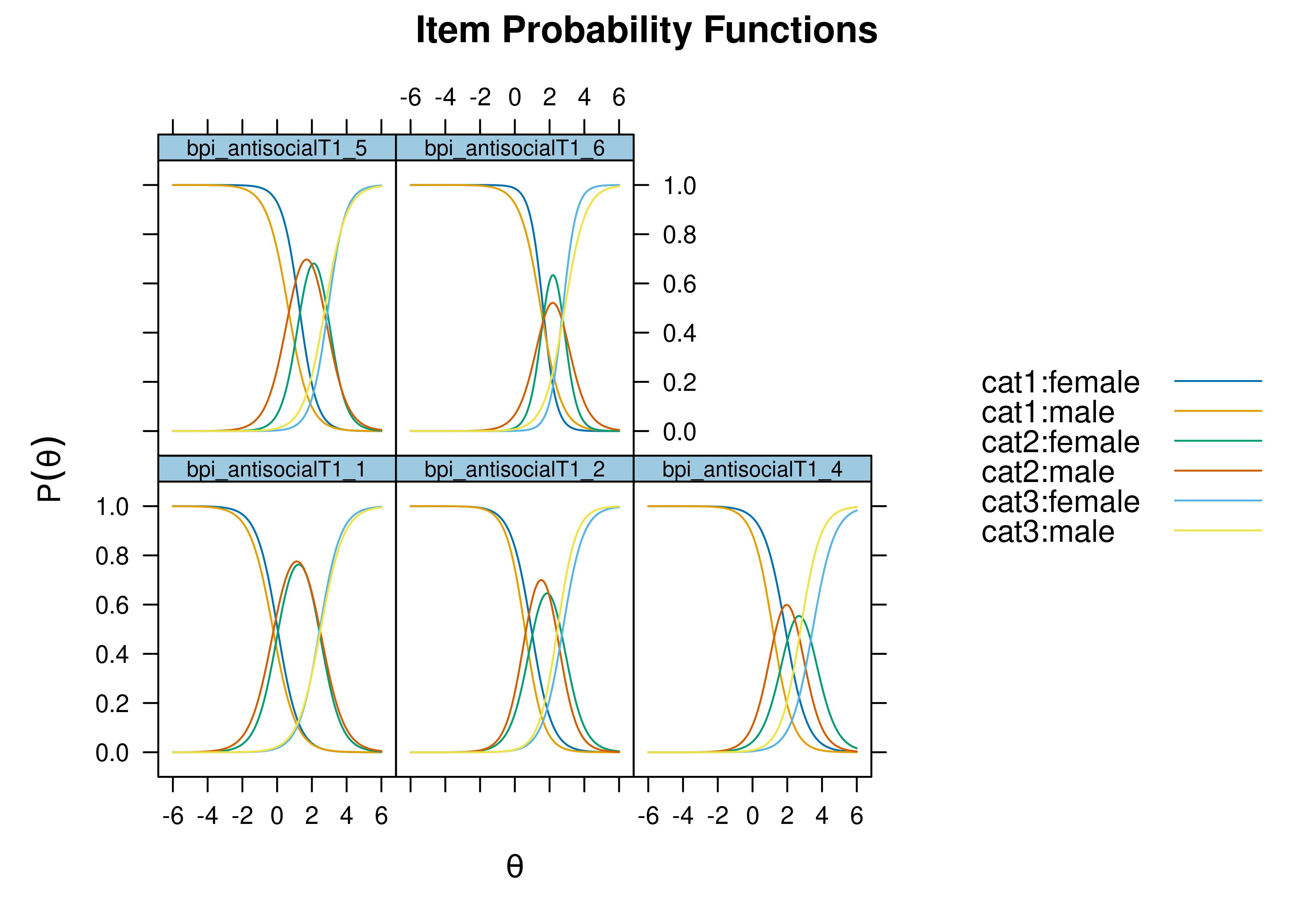
Figure 16.27: Item Probability Functions for Examining Differential Item Functioning in Terms of Discrimination and/or Severity.
16.9.6.2 Items that differ in discrimination
Items 6 and 7 showed DIF in discrimination, based on a significant chi-square difference test. The DIF in discrimination is depicted in Figure 16.28.
Code
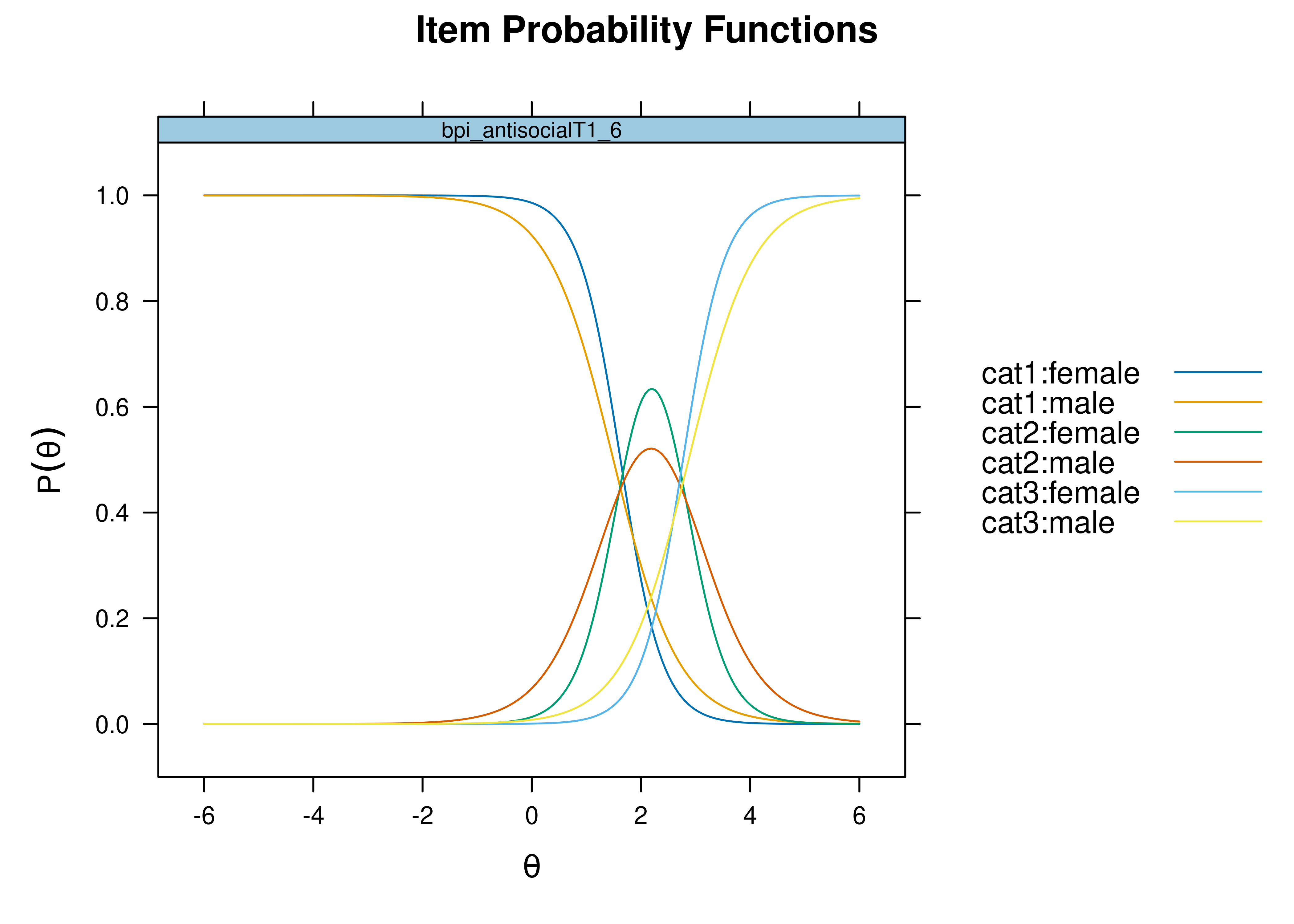
Figure 16.28: Item Probability Functions for Examining Differential Item Functioning in Terms of Discrimination.
16.9.6.3 Items that differ in severity
Items 1, 2, 3, 4, 5, and 6 showed DIF in difficulty/severity, based on a significant chi-square difference test. The DIF in difficulty/severity is depicted in Figure 16.29
Code
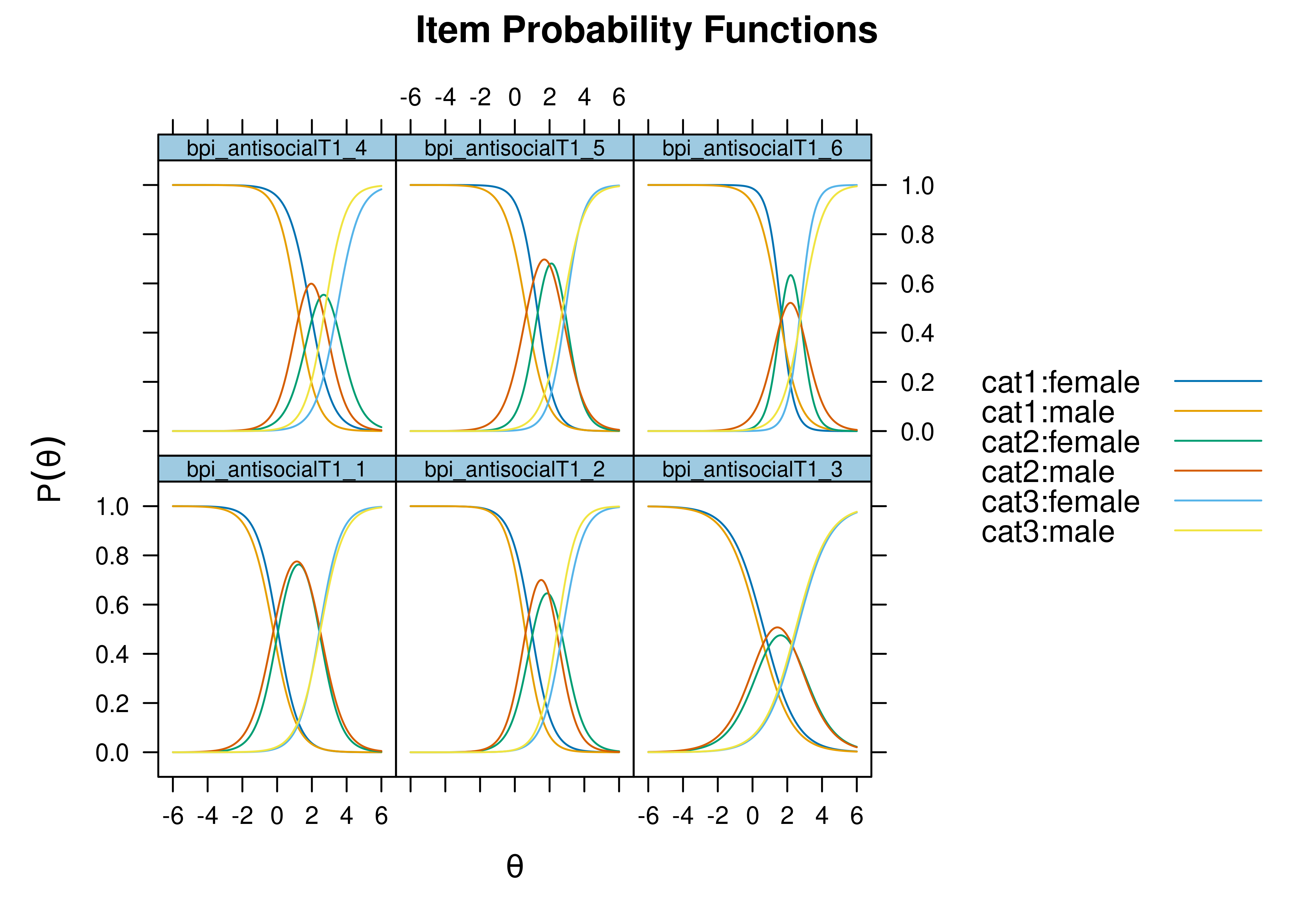
Figure 16.29: Item Probability Functions for Examining Differential Item Functioning in Terms of Severity.
16.9.7 Compute effect size of DIF
Effect size measures of DIF were computed based on expected scores (Meade, 2010) using the mirt
package (Chalmers, 2020).
Some researchers recommend using a simulation-based procedure to examine the impact of DIF on screening accuracy (Gonzalez & Pelham, 2021).
16.9.7.1 Test-level DIF
In addition to consideration of differential test functioning, we can also consider whether the test as a whole (i.e., the collection of items) differs in its functioning by group—called differential test functioning. Differential test functioning is depicted in Figure 16.30. Estimates of differential test functioning are in Table ??.
In general, the measure showed greater difficulty for females than for males. That is, at a given construct level, males were more likely than females to endorse the items. Otherwise said, it takes a higher construct level for females to obtain the same score on the measure as males.
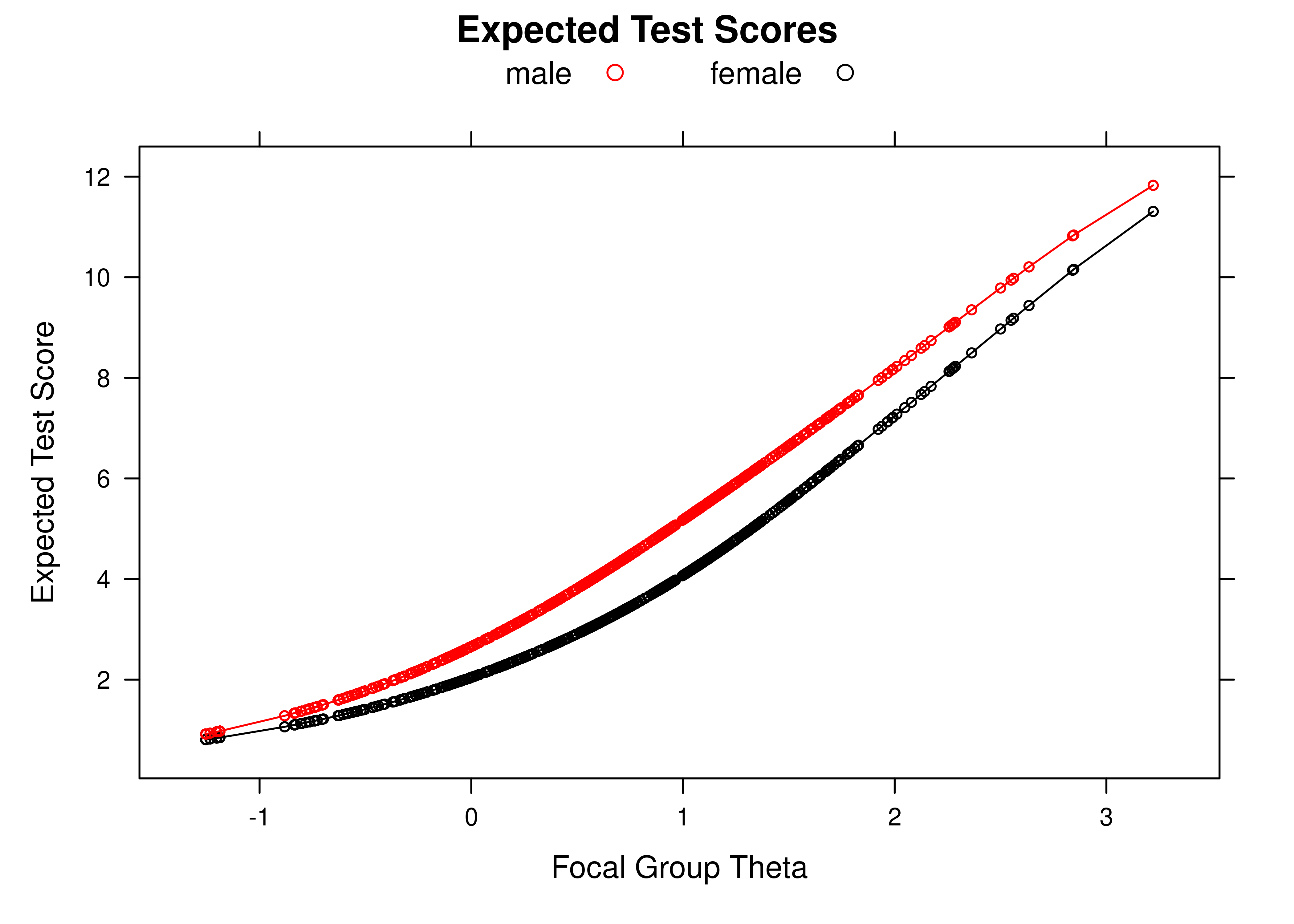
Figure 16.30: Differential Test Functioning by Sex.
16.9.7.2 Item-level DIF
Differential item functioning is depicted in Figure 16.31. Estimates of differential item functioning are in Table ??.
The measure-level differences in functioning appear to be largely driven by greater difficulty of items 4 and 5 for females than males. That is, at a given construct level, males were more likely than females to endorse items 4 and 5, in particular. Otherwise said, it takes a higher construct level for females than males to endorse items 4 and 5.
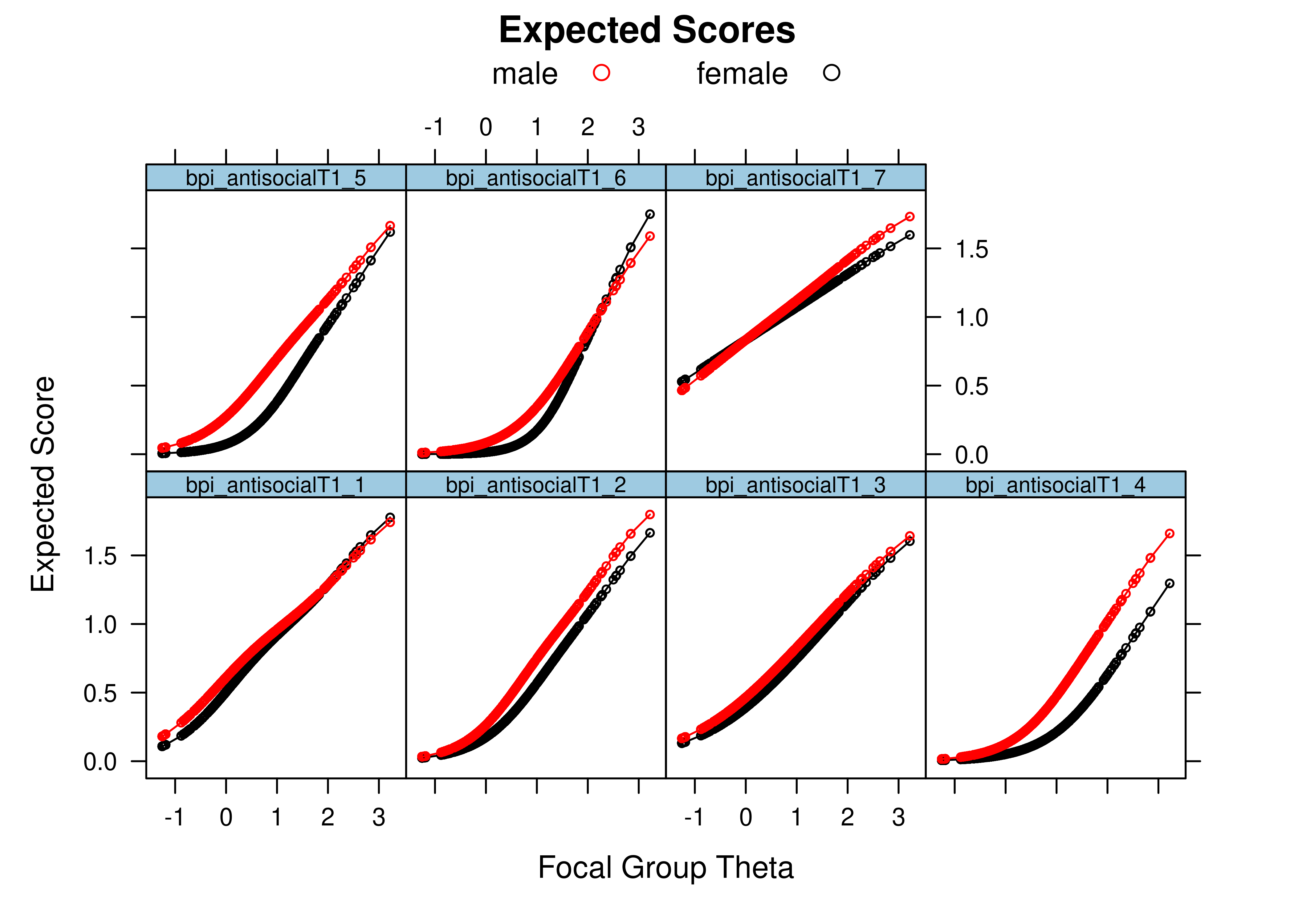
Figure 16.31: Differential Item Functioning by Sex.
16.9.8 Item plots
Plots of item response category characteristic curves by sex are in Figures 16.32–16.38 below.
Code
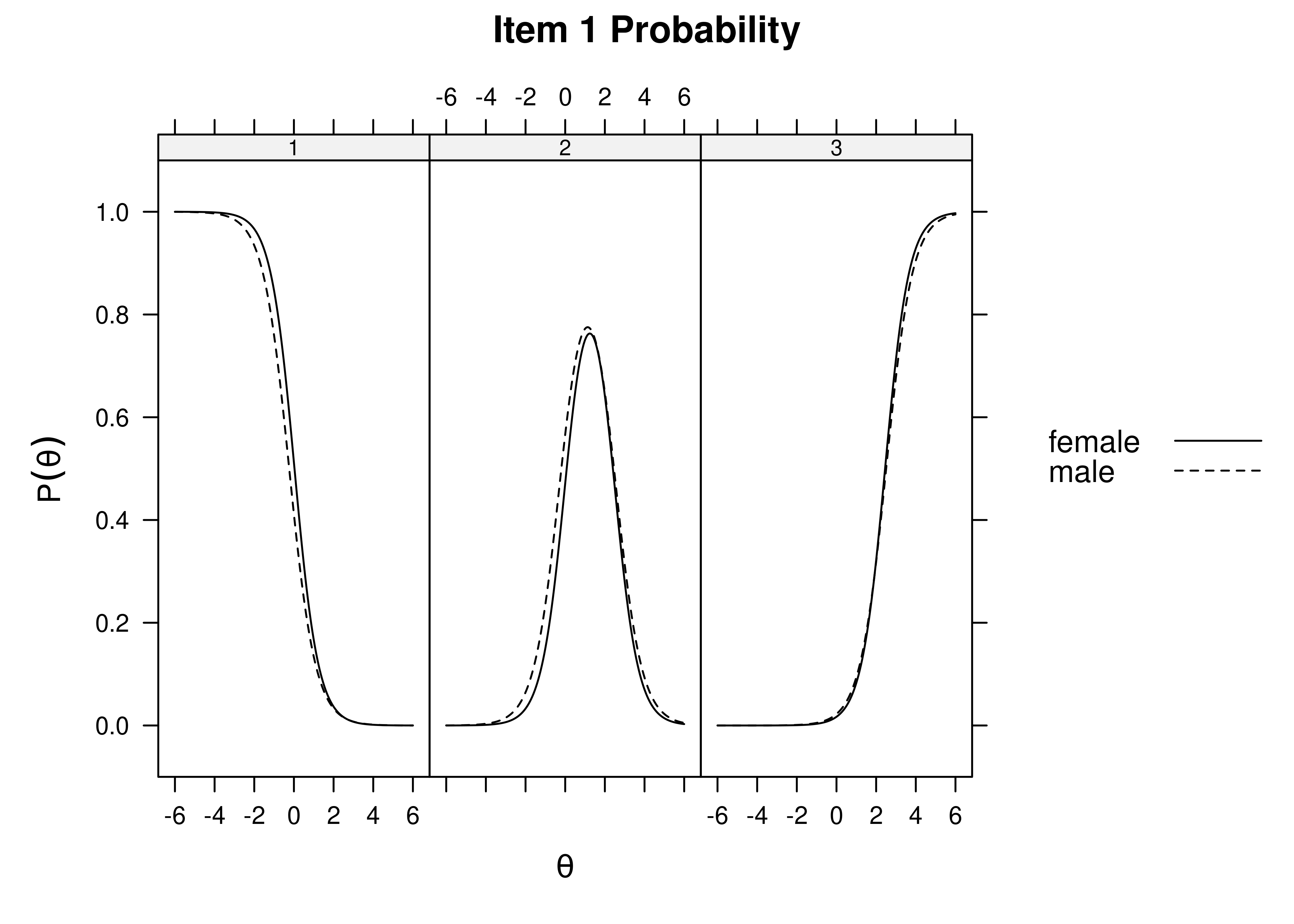
Figure 16.32: Item Response Category Characteristic Curves by Sex: Item 1.
Code
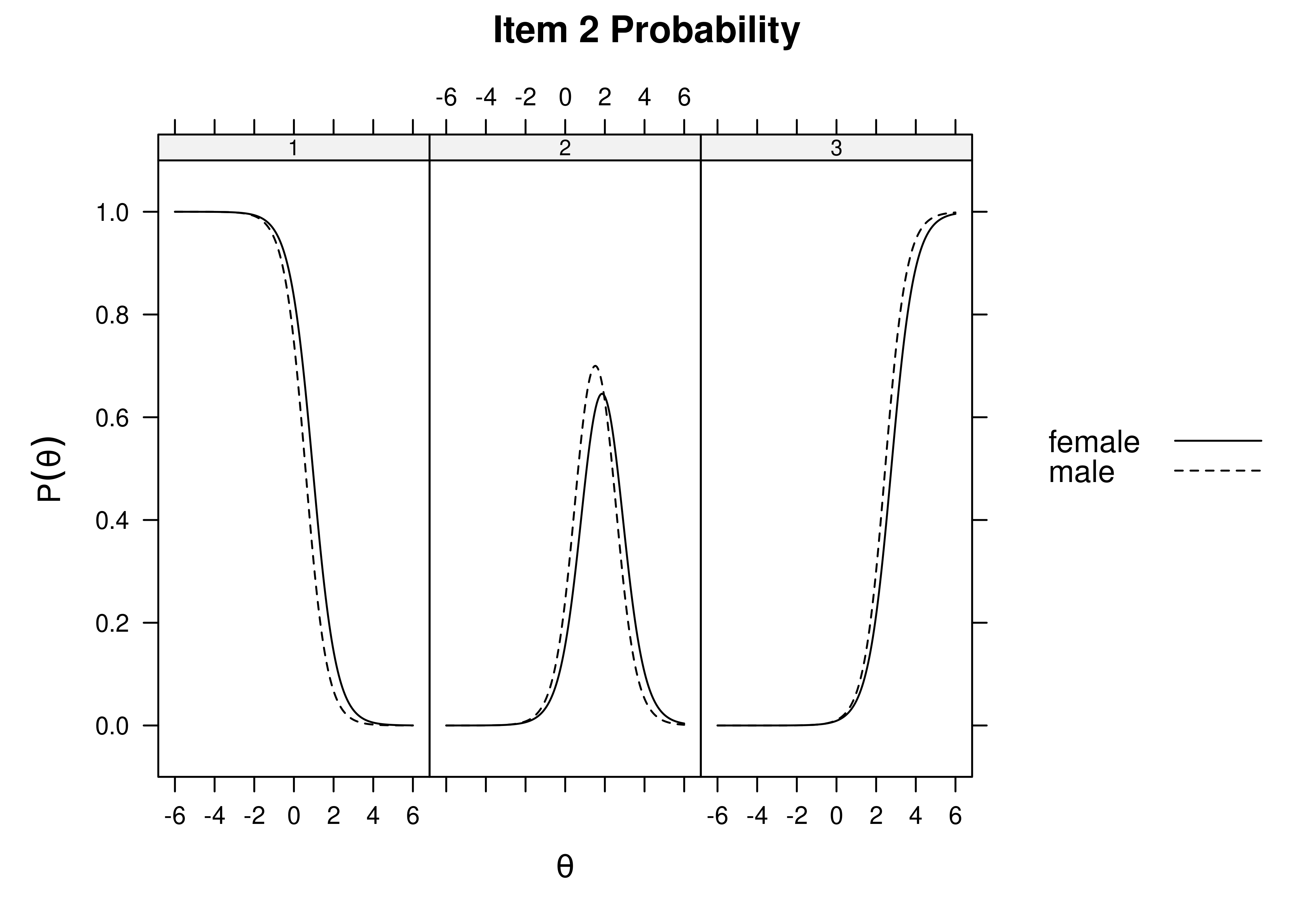
Figure 16.33: Item Response Category Characteristic Curves by Sex: Item 2.
Code
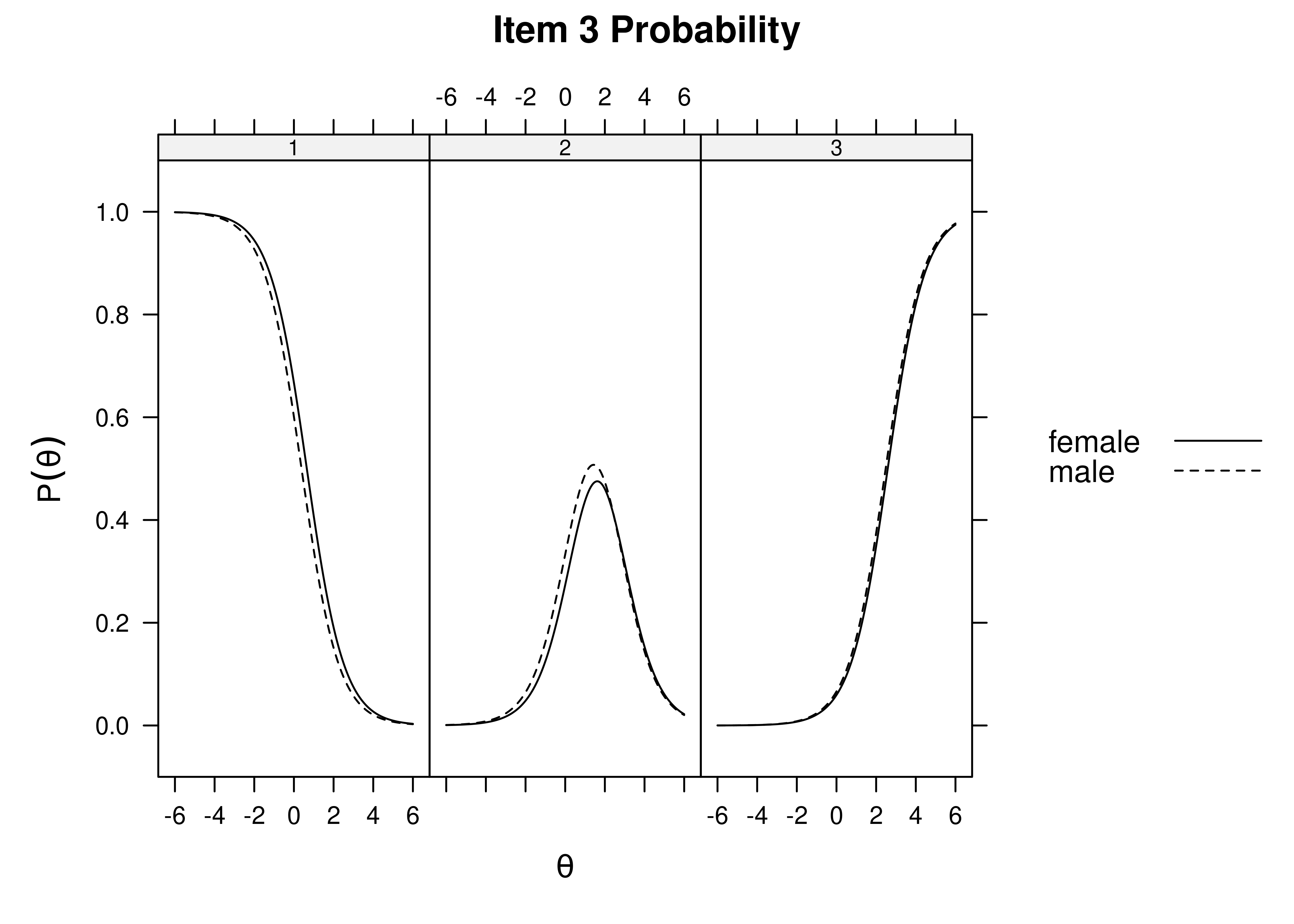
Figure 16.34: Item Response Category Characteristic Curves by Sex: Item 3.
Code
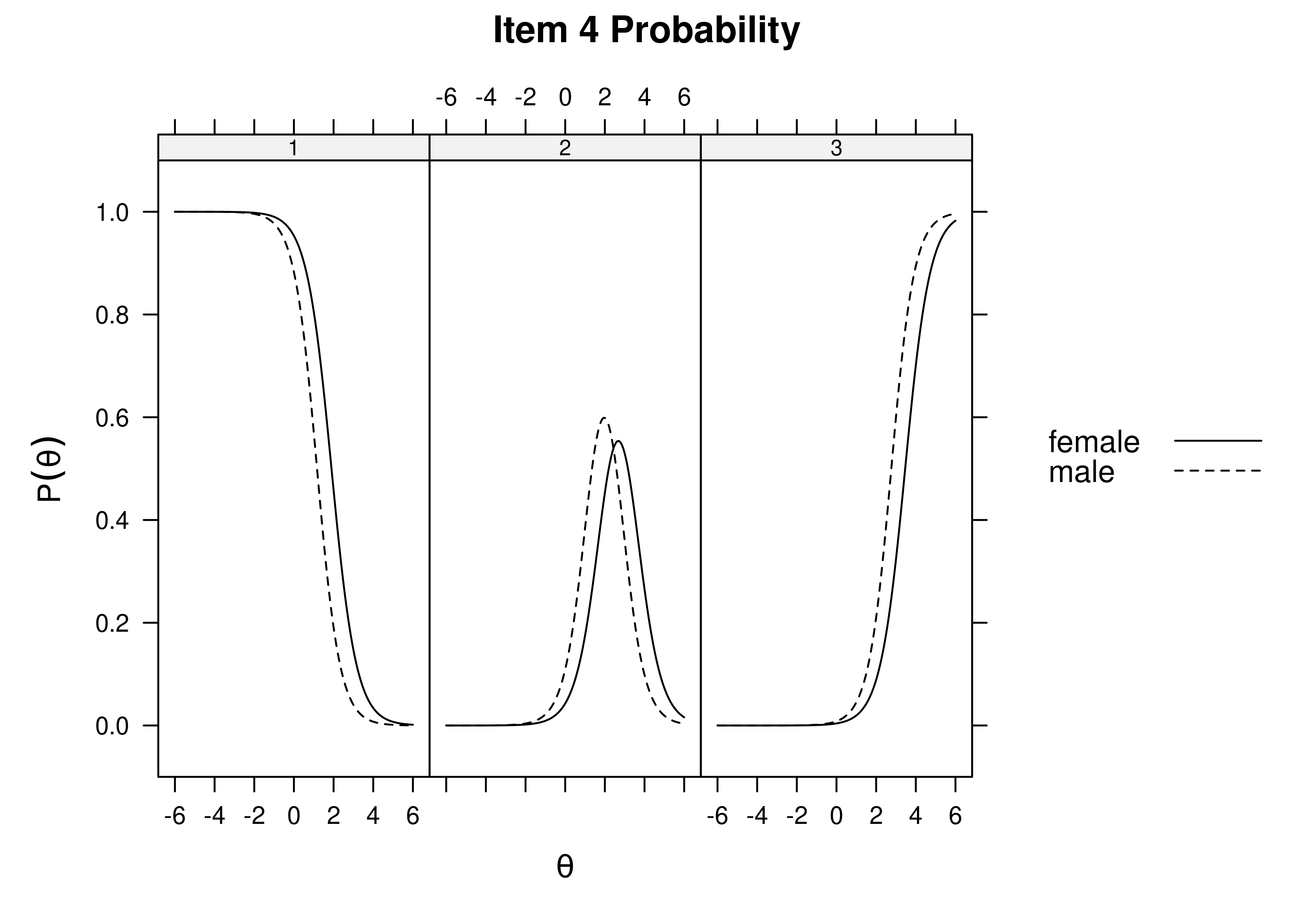
Figure 16.35: Item Response Category Characteristic Curves by Sex: Item 4.
Code
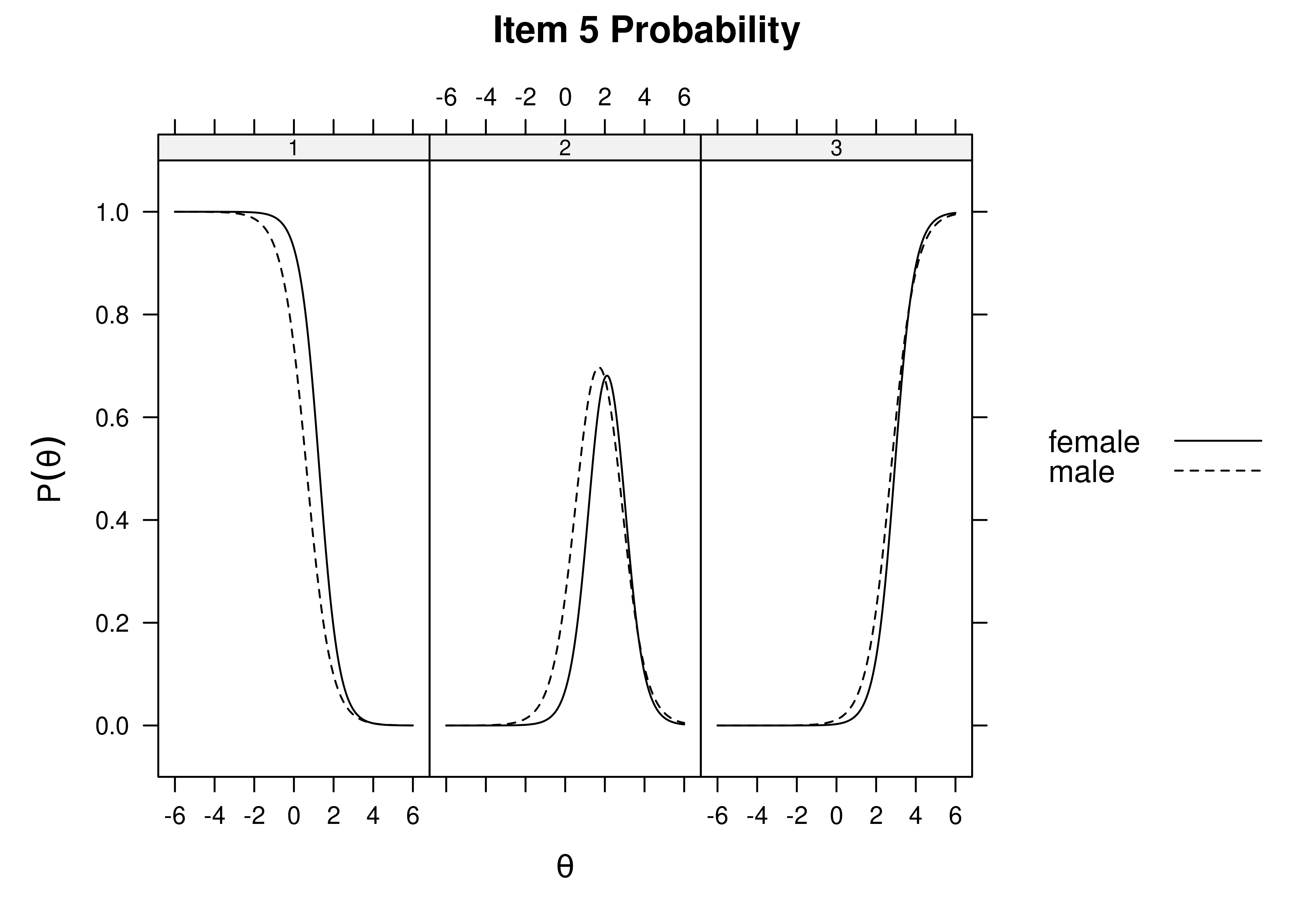
Figure 16.36: Item Response Category Characteristic Curves by Sex: Item 5.
Code
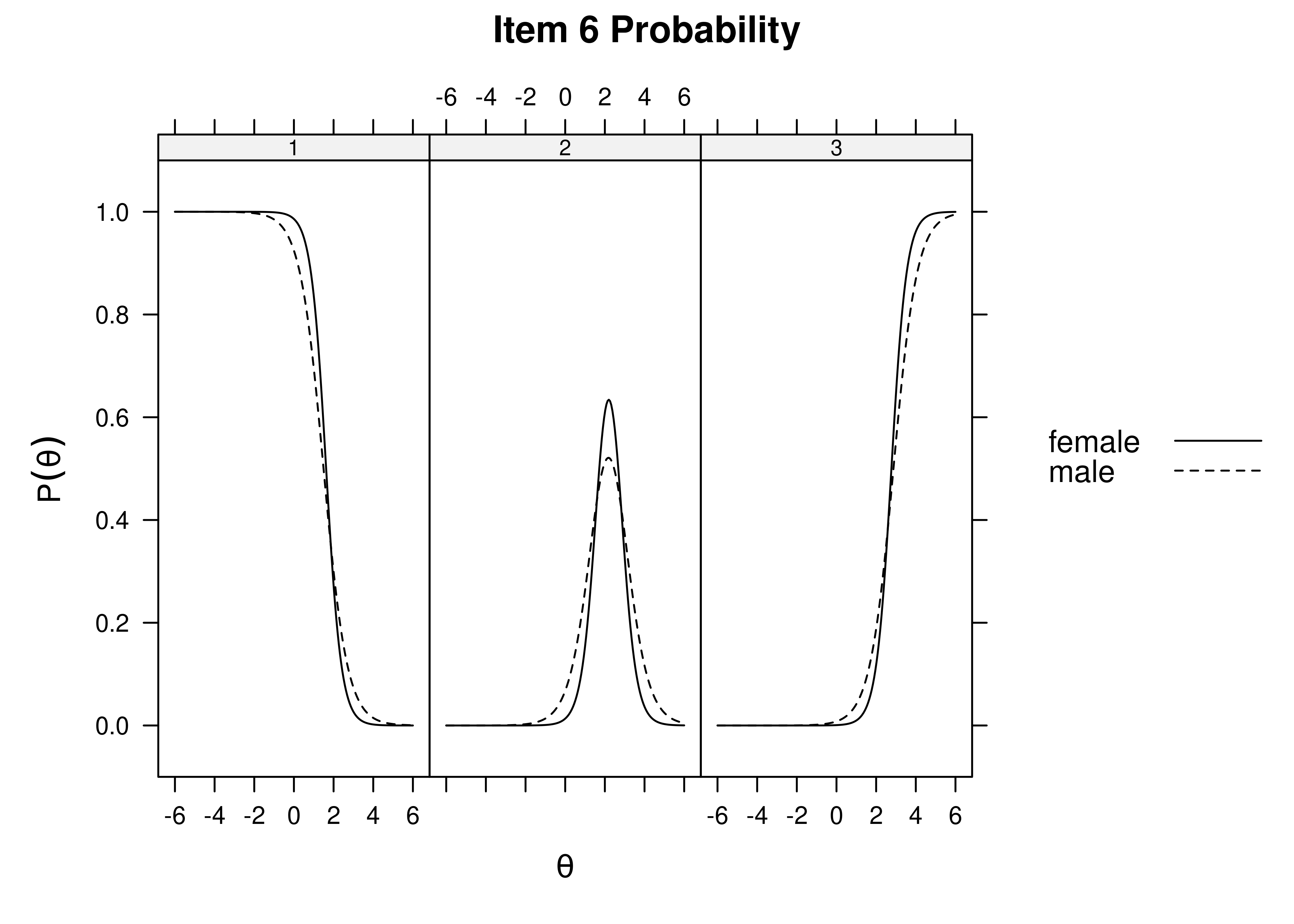
Figure 16.37: Item Response Category Characteristic Curves by Sex: Item 6.
Code
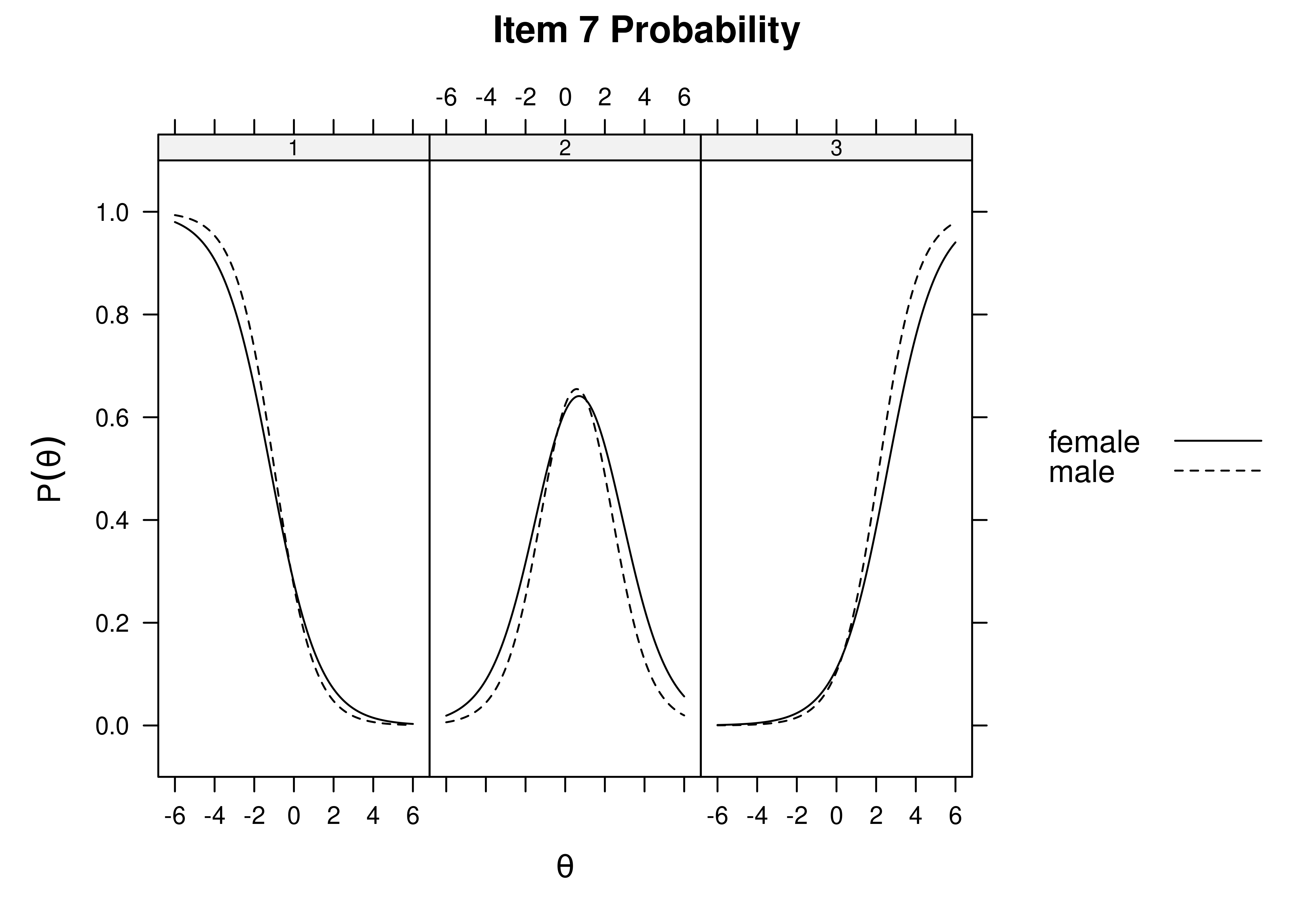
Figure 16.38: Item Response Category Characteristic Curves by Sex: Item 7.
Plots of item information functions by sex are in Figures 16.39–16.45 below. Items 1, 5, and 6 showed greater information for females than for males. Items 2, 4, and 7 showed greater information for females than for males.
Code
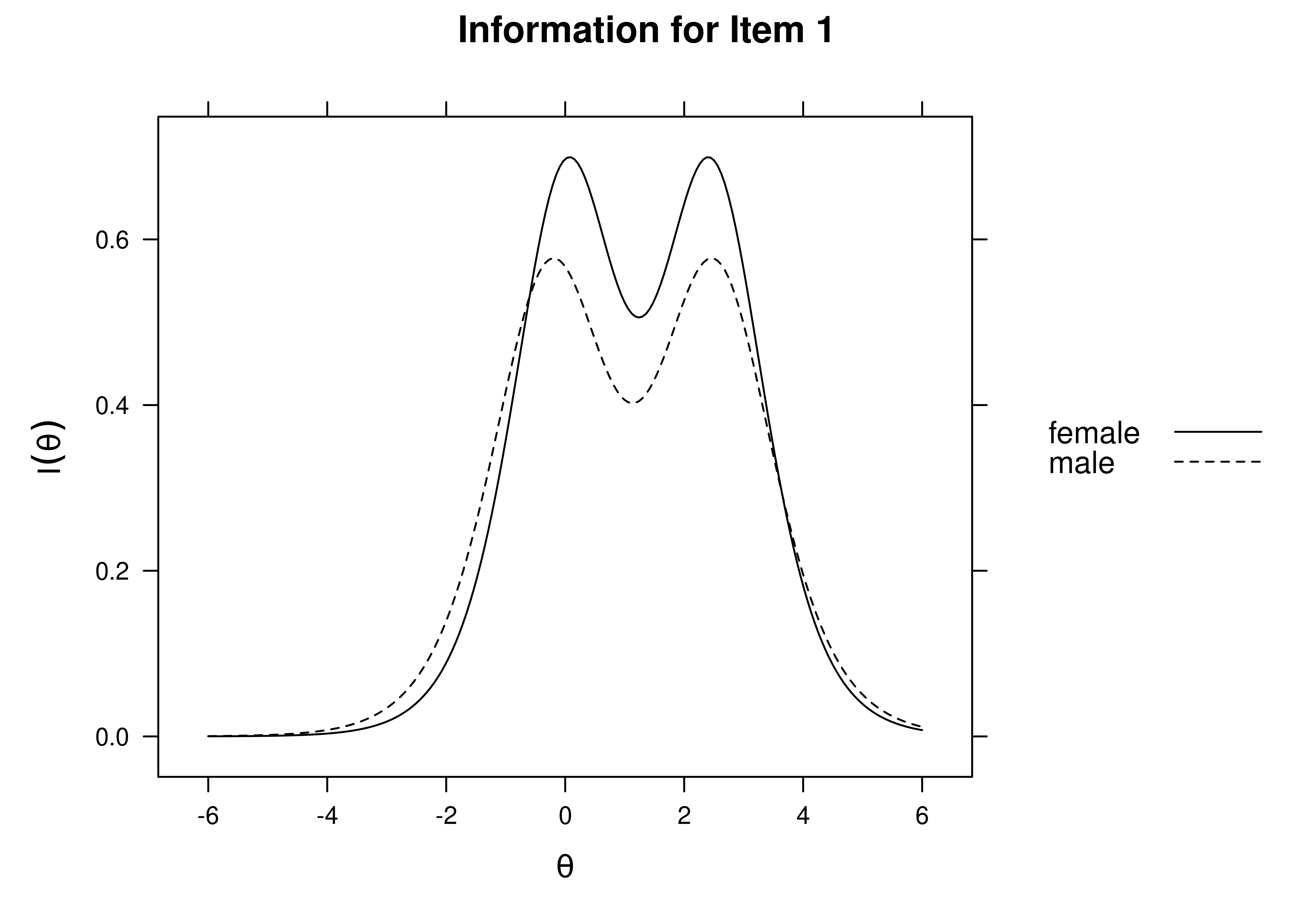
Figure 16.39: Item Information Curves by Sex: Item 1.
Code
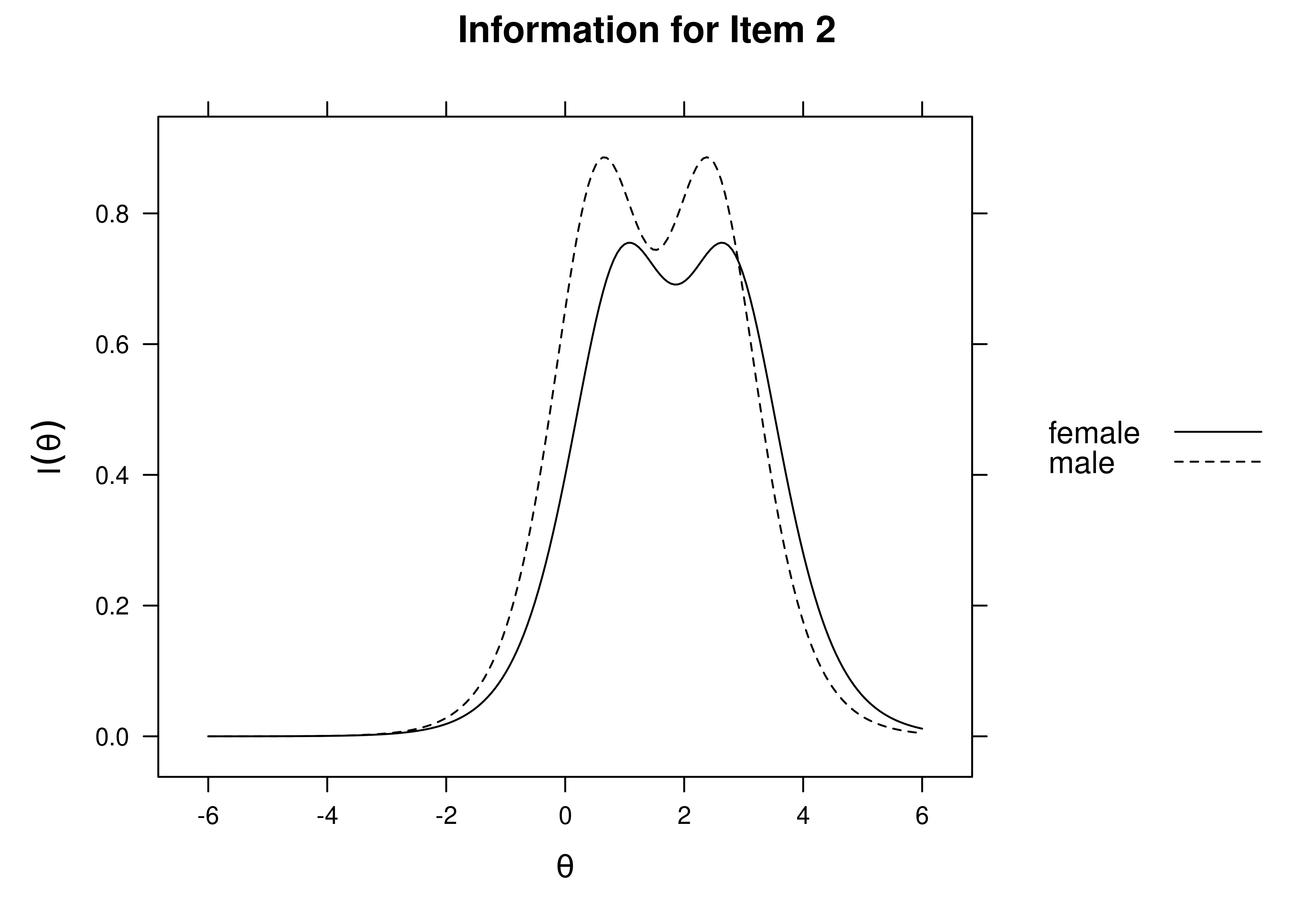
Figure 16.40: Item Information Curves by Sex: Item 2.
Code
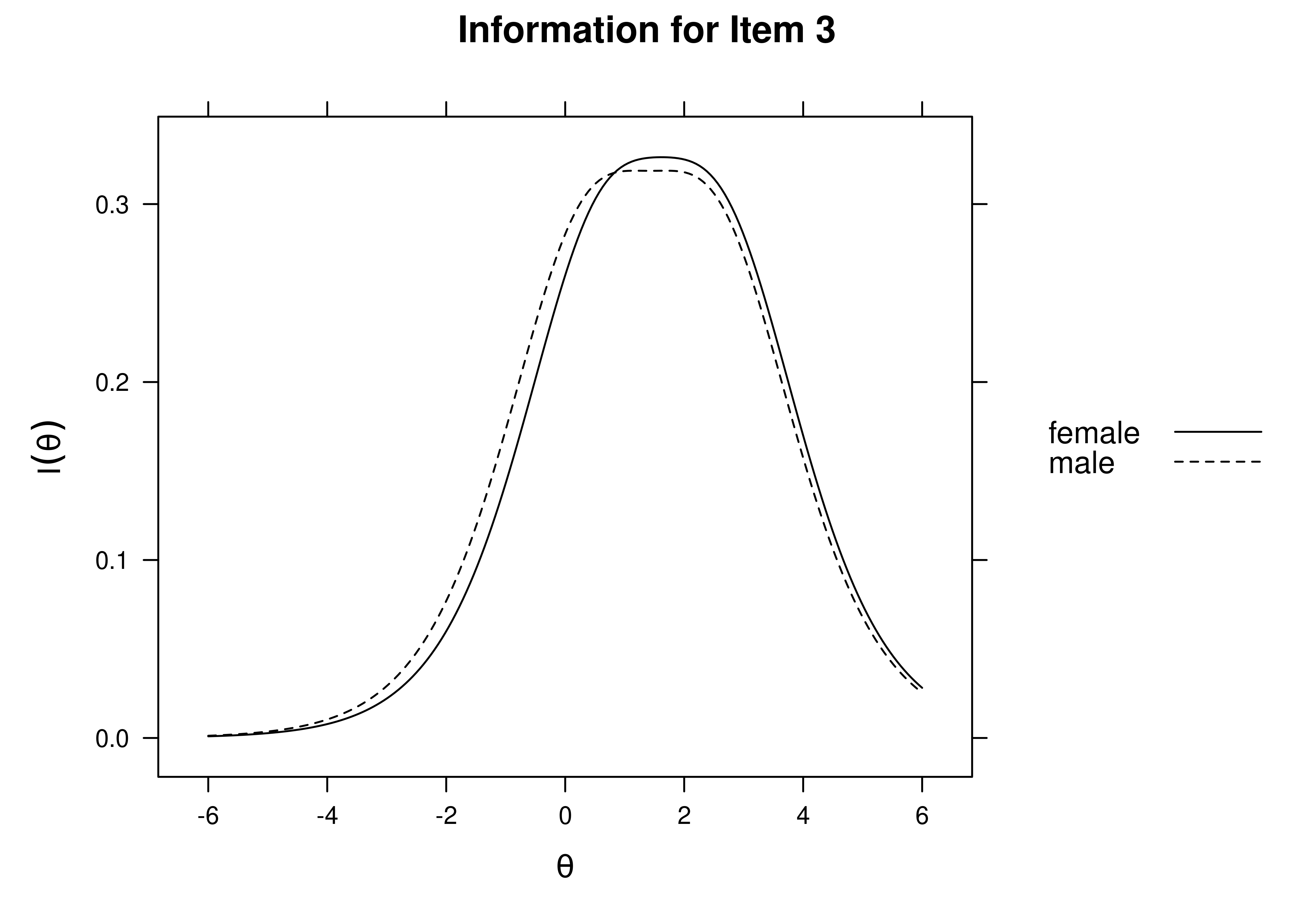
Figure 16.41: Item Information Curves by Sex: Item 3.
Code
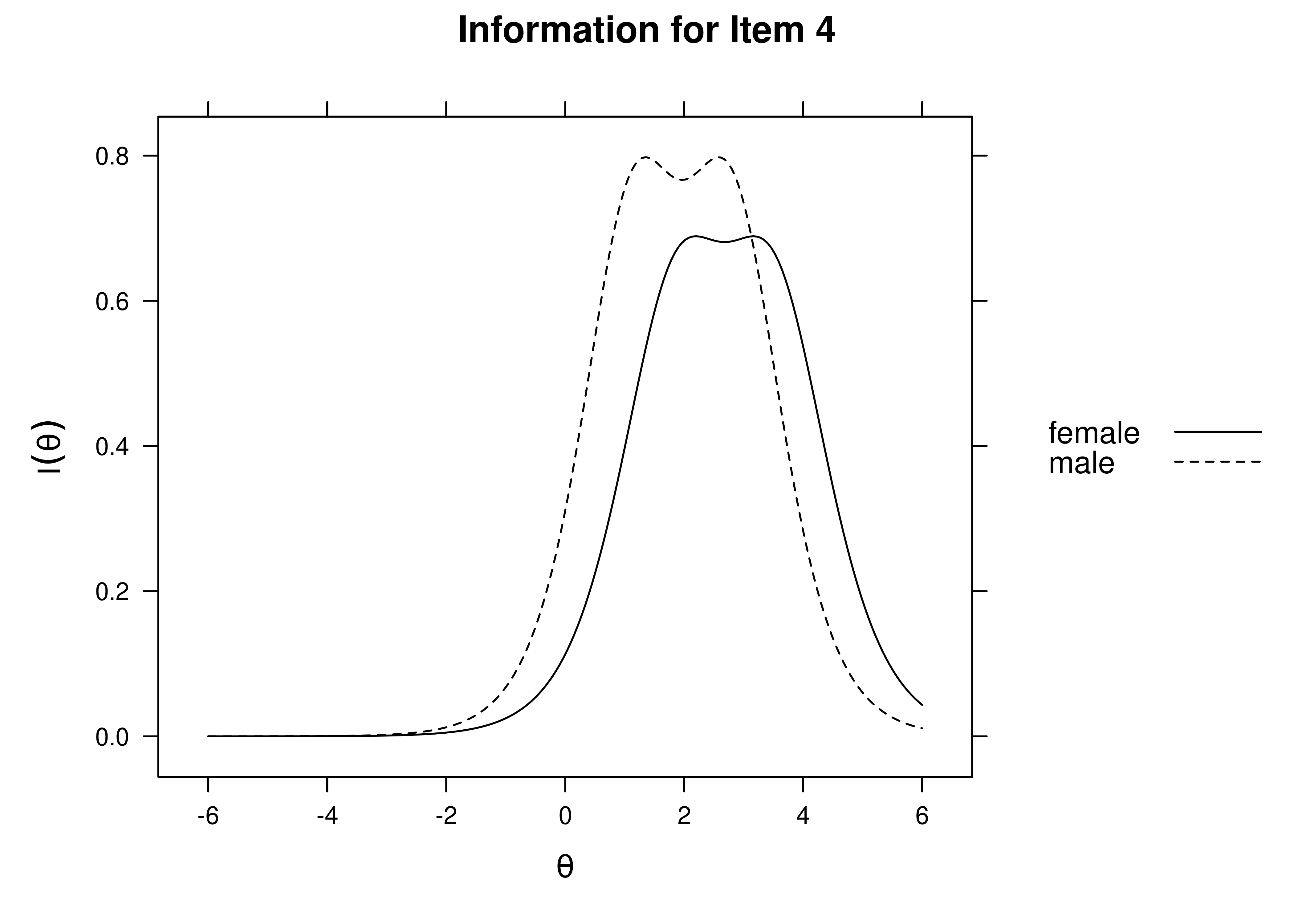
Figure 16.42: Item Information Curves by Sex: Item 4.
Code
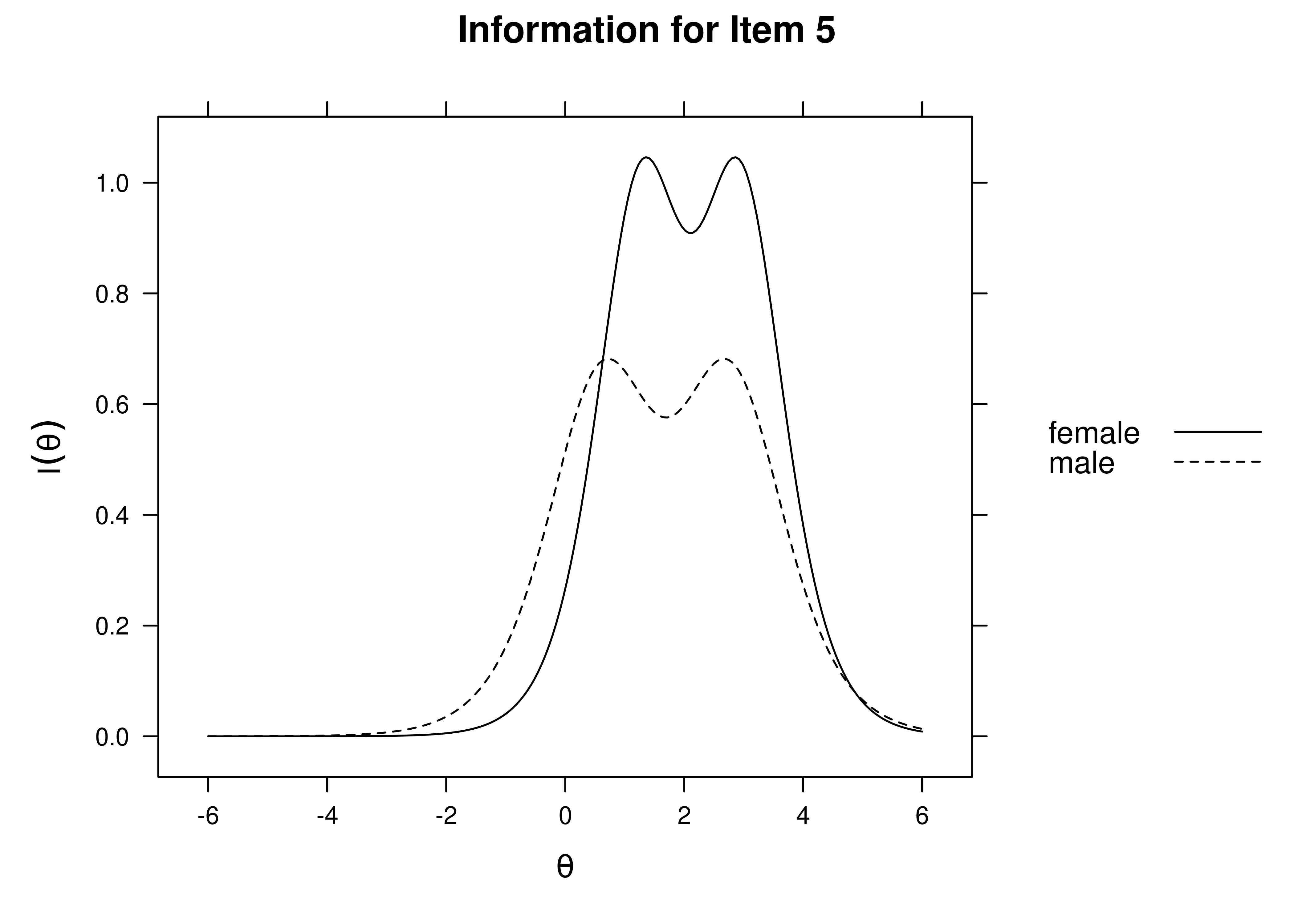
Figure 16.43: Item Information Curves by Sex: Item 5.
Code
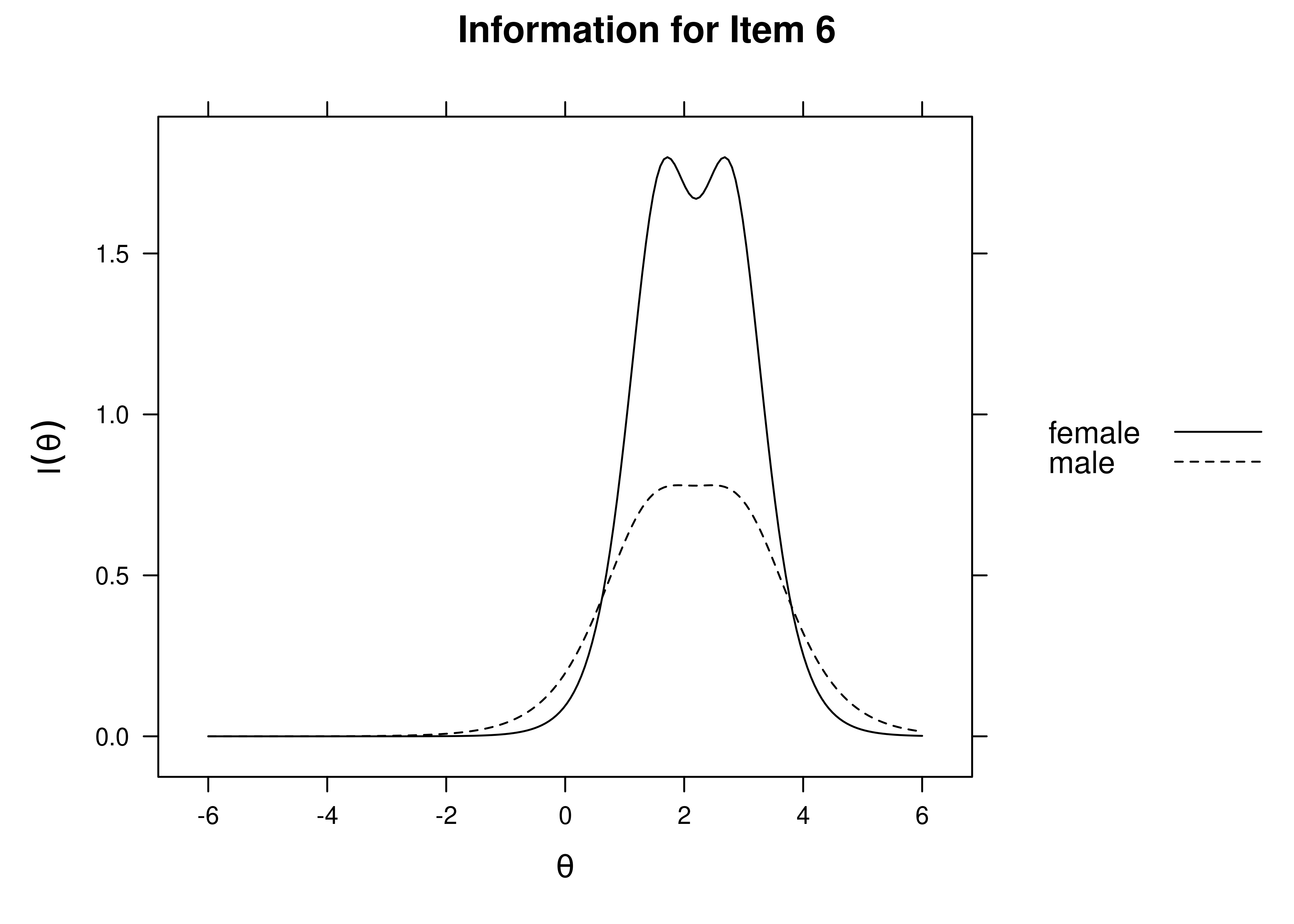
Figure 16.44: Item Information Curves by Sex: Item 6.
Code
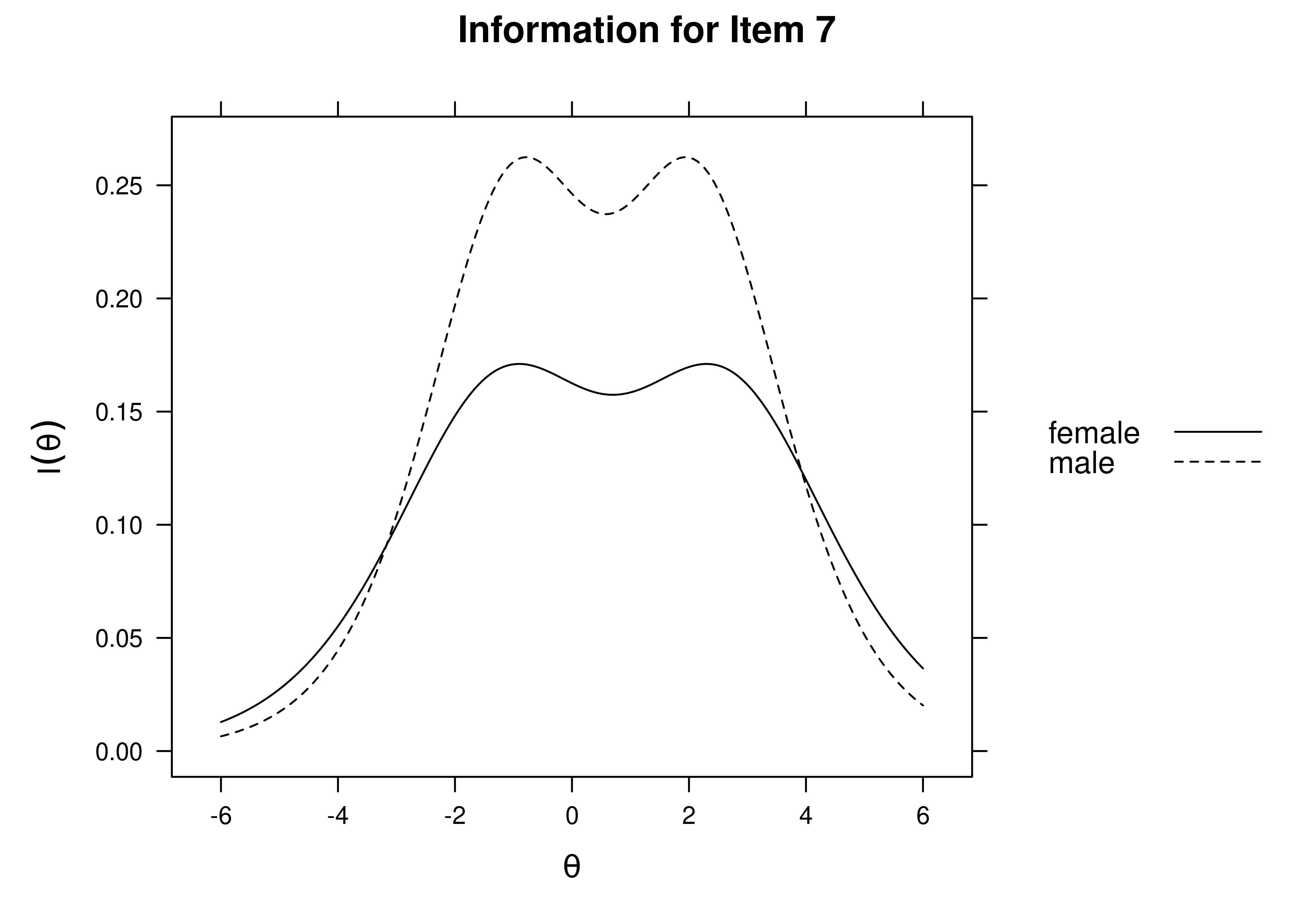
Figure 16.45: Item Information Curves by Sex: Item 7.
Plots of expected item scores by sex are are in Figures 16.46–16.52 below.
Code
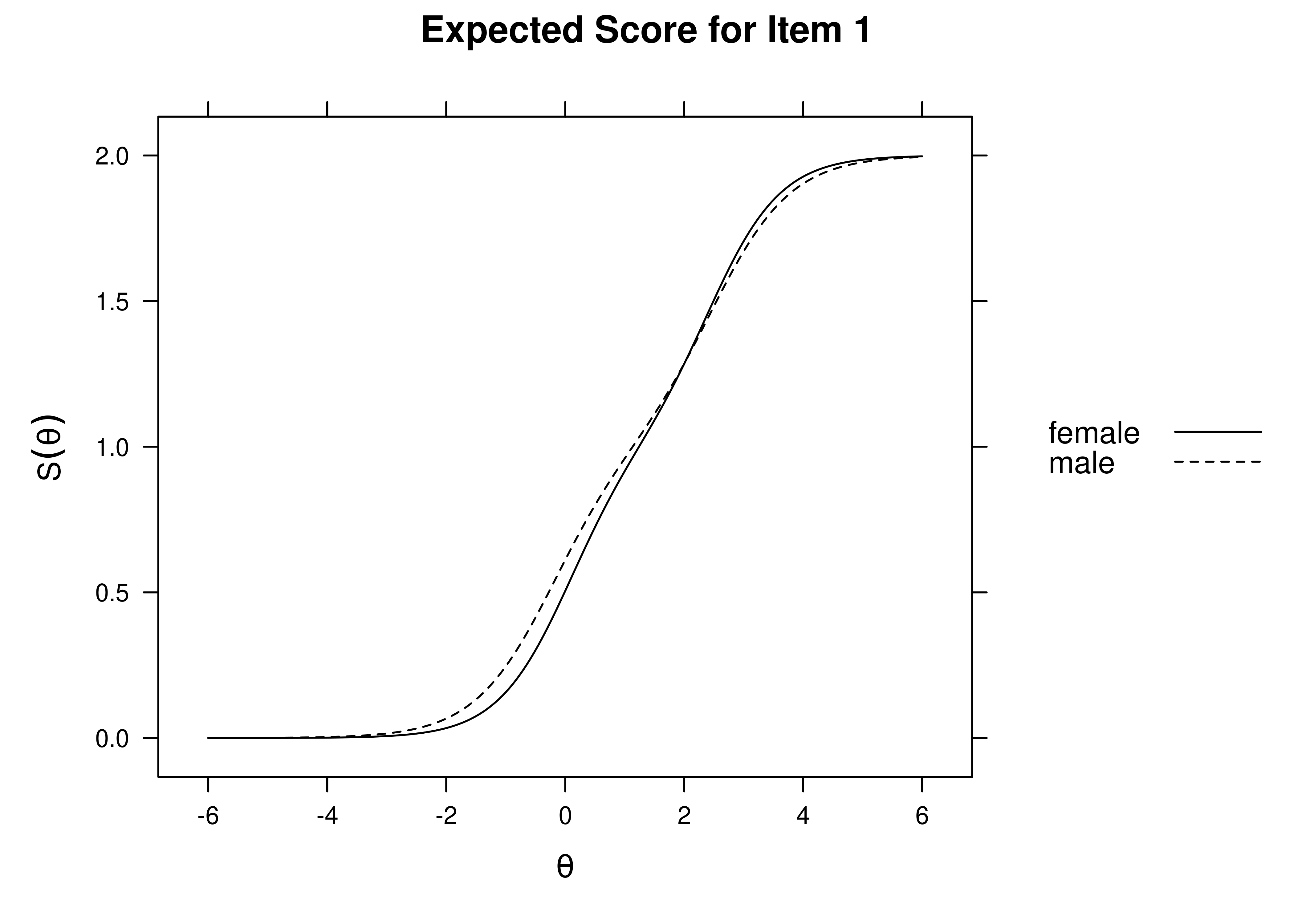
Figure 16.46: Expected Item Score by Sex: Item 1.
Code
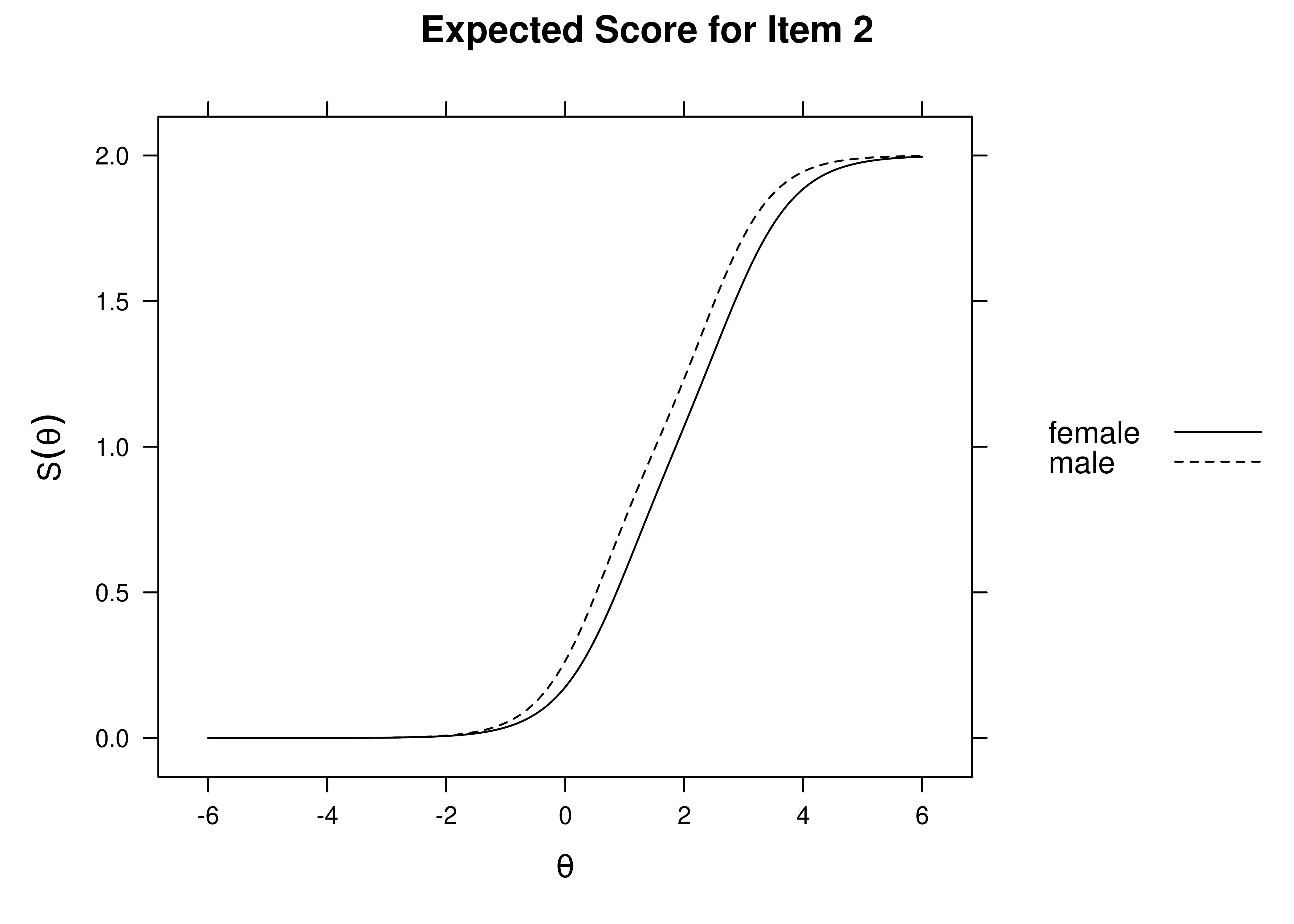
Figure 16.47: Expected Item Score by Sex: Item 2.
Code
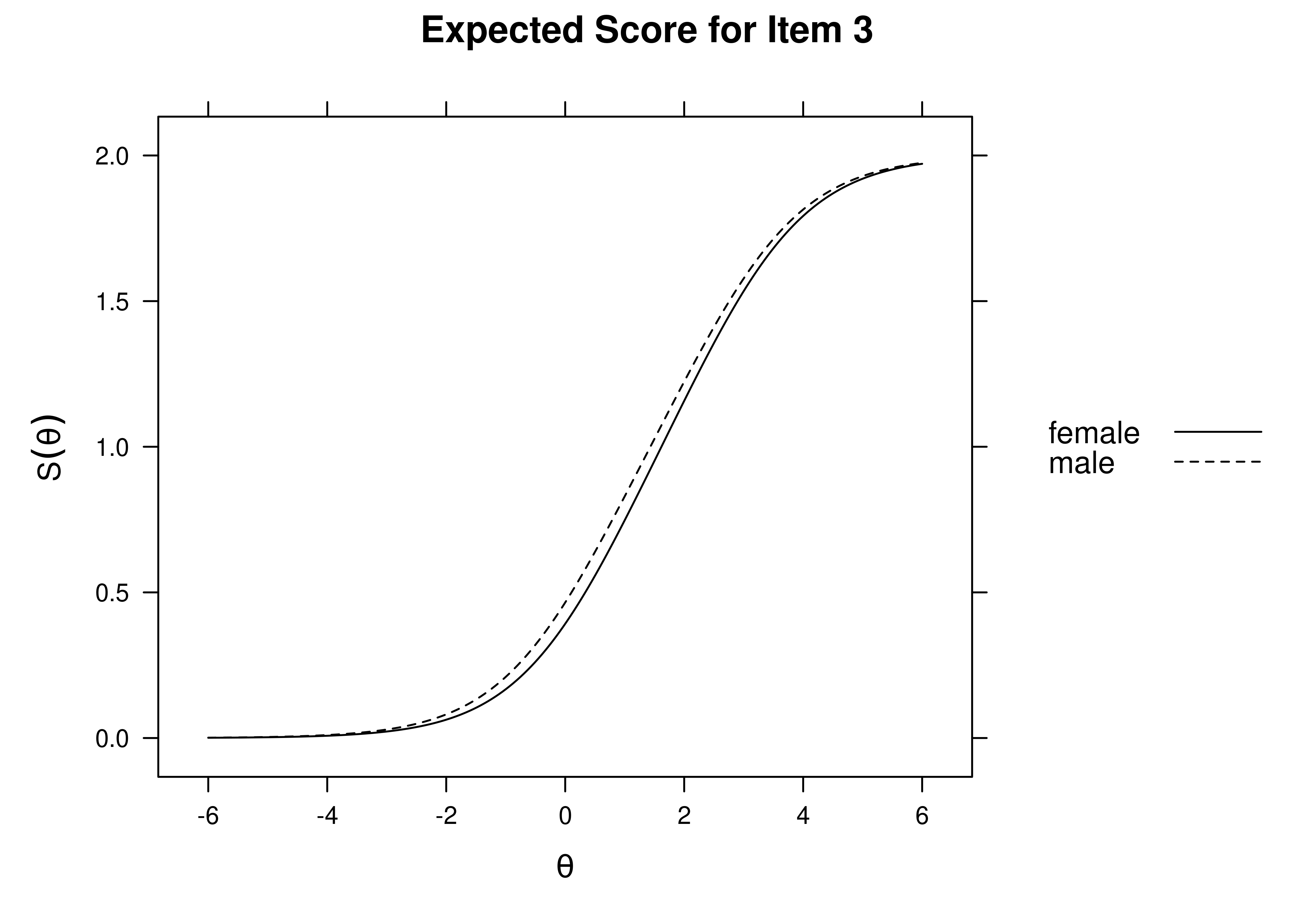
Figure 16.48: Expected Item Score by Sex: Item 3.
Code
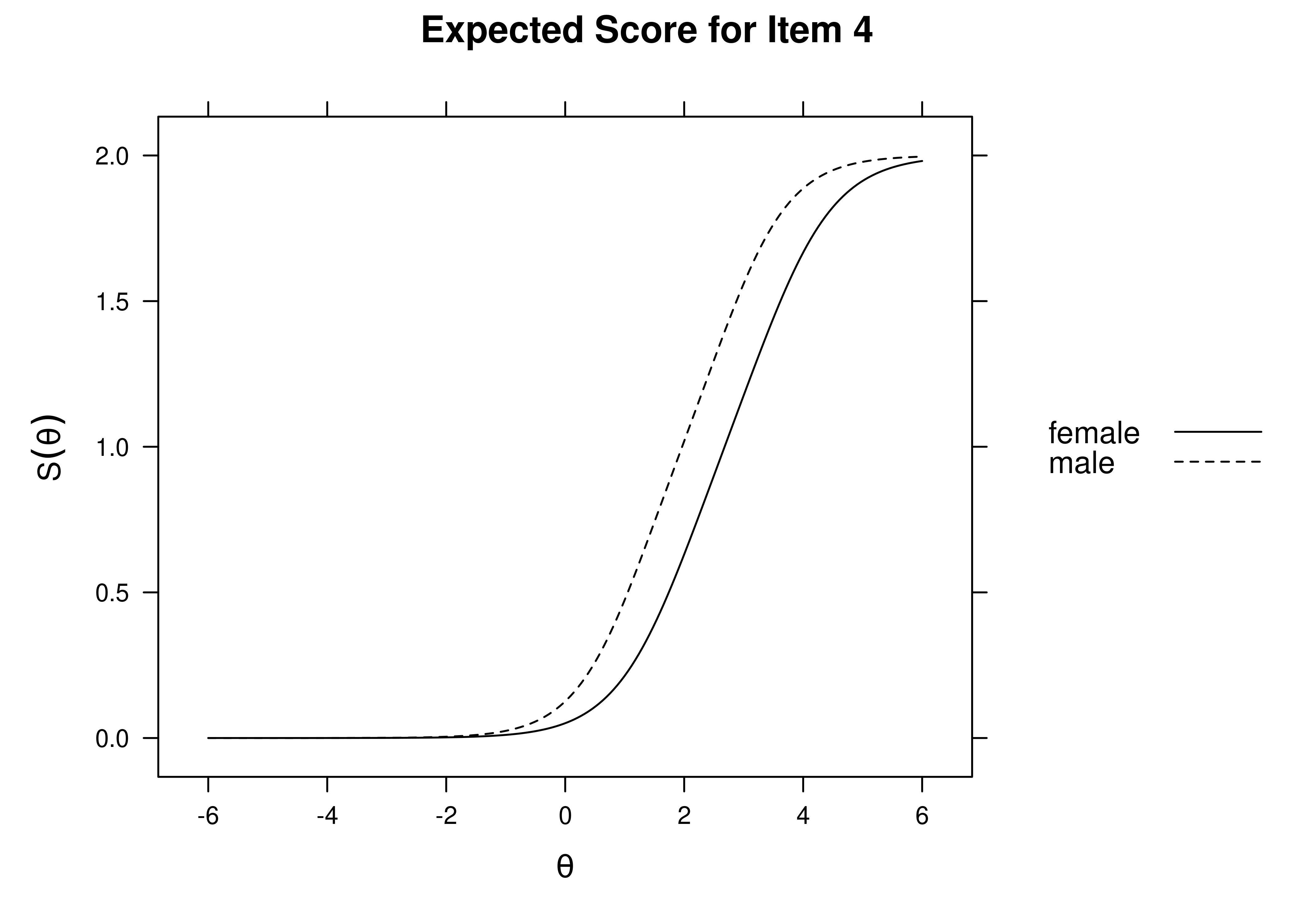
Figure 16.49: Expected Item Score by Sex: Item 4.
Code
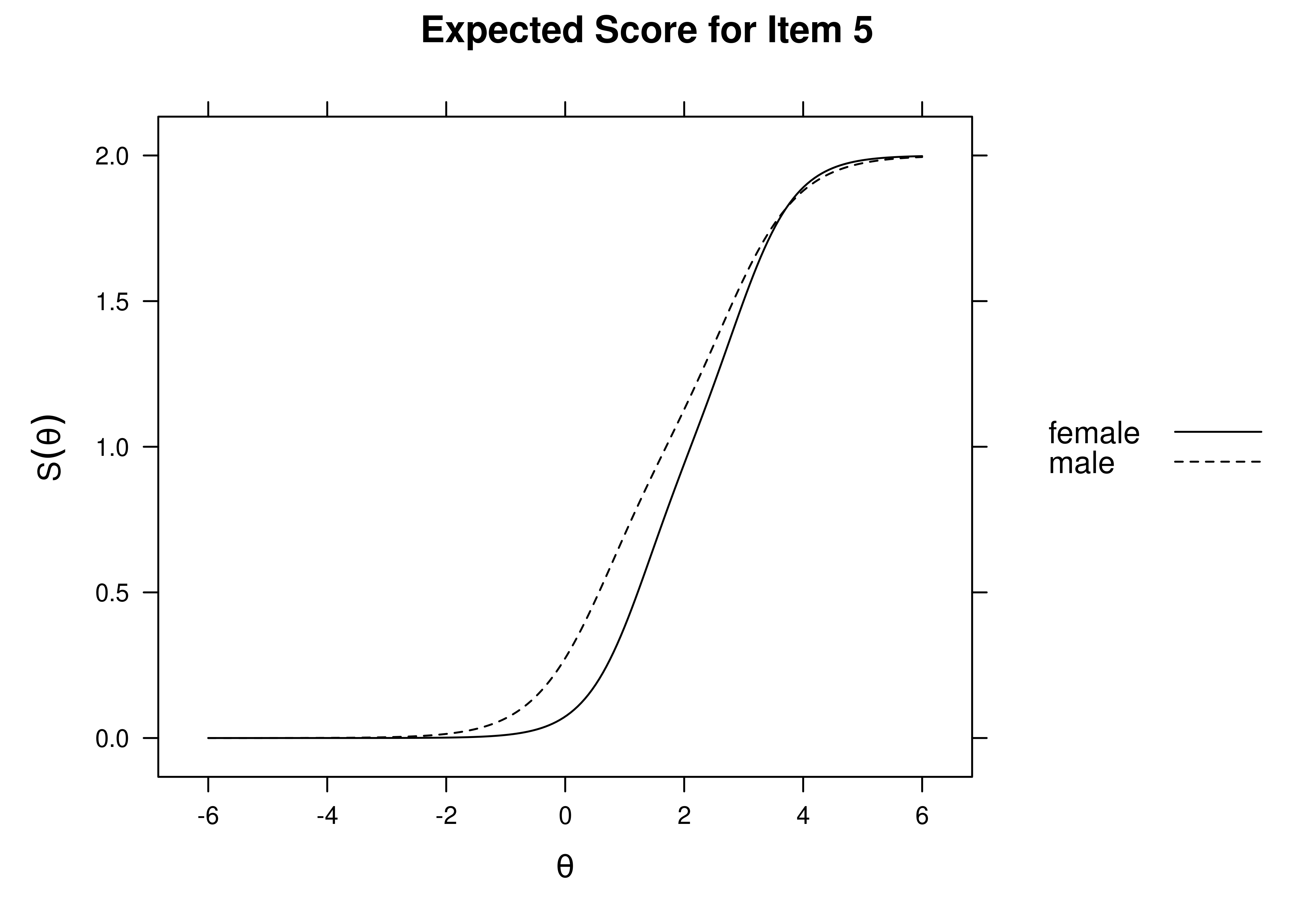
Figure 16.50: Expected Item Score by Sex: Item 5.
Code
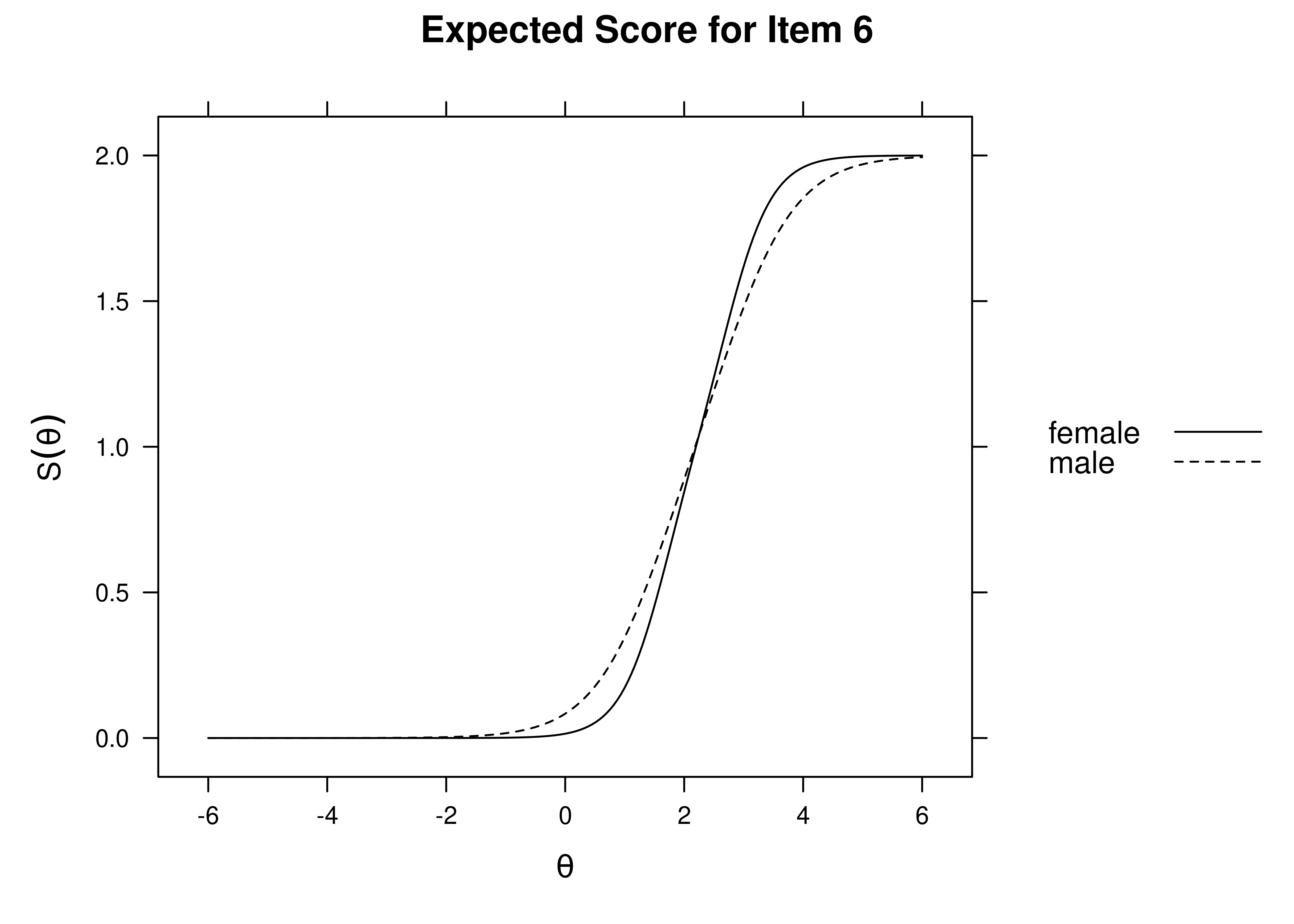
Figure 16.51: Expected Item Score by Sex: Item 6.
Code
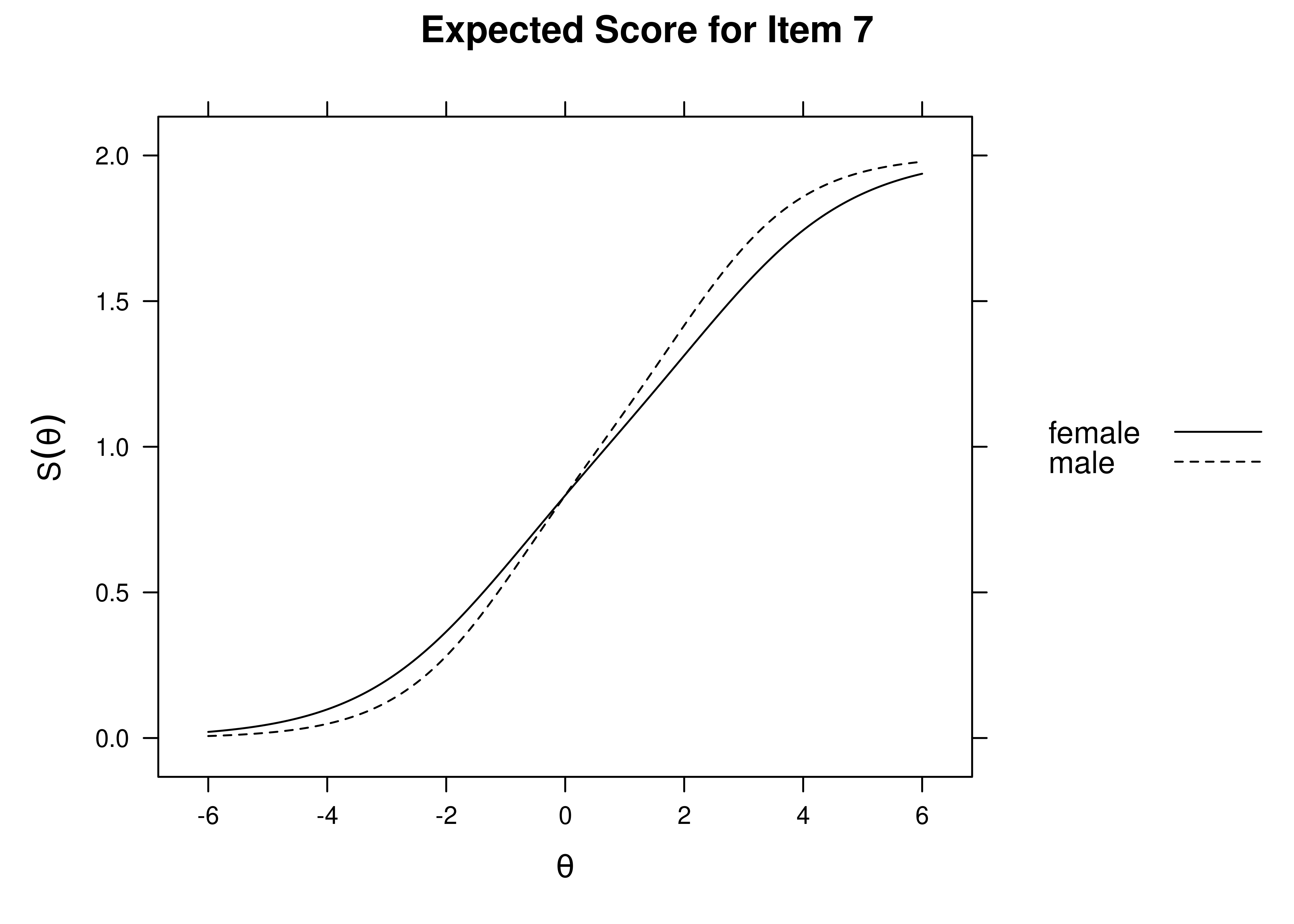
Figure 16.52: Expected Item Score by Sex: Item 7.
16.9.9 Addressing DIF
Based on the analyses above, item 5 shows the largest magnitude of DIF. Specifically, item 5 has a stronger discrimination parameter for women than men. So, we should handle item 5 first. If we deem the DIF for item 5 to be non-negligible, we have three primary options: (1) drop item 5 for both men and women, (2) drop item 5 for men but keep it for women, or (3) freely estimate the parameters (discrimination and difficulty) for item 5 across groups.
16.9.9.1 Drop item for both groups
The first option is to drop item 5 for both men and women groups. You might do this if you want to use a measure that has only those items that function equivalently across groups. However, this can lead to lower reliability and weaker ability to detect individual differences.
Code
constrainedModelDropItem5 <- multipleGroup(
data = cnlsy[,c(
"bpi_antisocialT1_1","bpi_antisocialT1_2","bpi_antisocialT1_3",
"bpi_antisocialT1_4","bpi_antisocialT1_6","bpi_antisocialT1_7")],
model = 1,
group = cnlsy$sex,
invariance = c(c(
"bpi_antisocialT1_1","bpi_antisocialT1_2","bpi_antisocialT1_3",
"bpi_antisocialT1_4","bpi_antisocialT1_6","bpi_antisocialT1_7"),
"free_means", "free_var"),
SE = TRUE)
coef(constrainedModelDropItem5, simplify = TRUE)
16.9.9.2 Drop item for one group but not another group
A second option is to drop item 5 for men but to keep it for women. You might do this if the item is invalid for men, but still valid for women. The coefficients show an item parameter for item 5 for men, but it was constrained to be equal to the parameter for women, and data were removed from the estimation of item 5 for men.
Code
dropItem5ForMen <- cnlsy
dropItem5ForMen[which(
dropItem5ForMen$sex == "male"),
"bpi_antisocialT1_5"] <- NA
constrainedModelDropItem5ForMen <- multipleGroup(
data = dropItem5ForMen[,c(
"bpi_antisocialT1_1","bpi_antisocialT1_2","bpi_antisocialT1_3",
"bpi_antisocialT1_4","bpi_antisocialT1_5","bpi_antisocialT1_6",
"bpi_antisocialT1_7")],
model = 1,
group = dropItem5ForMen$sex,
invariance = c(c(
"bpi_antisocialT1_1","bpi_antisocialT1_2","bpi_antisocialT1_3",
"bpi_antisocialT1_4","bpi_antisocialT1_5","bpi_antisocialT1_6",
"bpi_antisocialT1_7"),
"free_means", "free_var"),
SE = TRUE)
coef(constrainedModelDropItem5ForMen, simplify = TRUE)
16.9.9.3 Freely estimate item to have different parameters across groups
Alternatively, we can resolve DIF by allowing the item to have different parameters (discrimination and difficulty) across both groups. You might do this if the item is valid for both men and women, and it has importantly different item parameters nonetheless.
Code
constrainedModelResolveItem5 <- multipleGroup(
data = cnlsy[,c(
"bpi_antisocialT1_1","bpi_antisocialT1_2","bpi_antisocialT1_3",
"bpi_antisocialT1_4","bpi_antisocialT1_5","bpi_antisocialT1_6",
"bpi_antisocialT1_7")],
model = 1,
group = cnlsy$sex,
invariance = c(c(
"bpi_antisocialT1_1","bpi_antisocialT1_2","bpi_antisocialT1_3",
"bpi_antisocialT1_4","bpi_antisocialT1_6","bpi_antisocialT1_7"),
"free_means", "free_var"),
SE = TRUE)
coef(constrainedModelResolveItem5, simplify = TRUE)
16.9.9.4 Compare model fit
We can compare the model fit against the fully constrained model. In this case, all three of these approaches for handling DIF resulted in improvement in model fit based on AIC and, for the two nested models, the chi-square difference test.
16.9.9.5 Next steps
Whichever of these approaches we select, we would then identify and handle the remaining non-negligent DIF sequentially by magnitude. For instance, if we chose to resolve DIF in item 5 by allowing the item to have different parameters across both groups, we would then iteratively drop constraints to see which items continue to show non-negligible DIF.
Code
groups | converged | AIC | SABIC | HQ | BIC | X2 | df | p | |
---|---|---|---|---|---|---|---|---|---|
bpi_antisocialT1_1 | female,male | TRUE | -5.5042001 | 3.7824138 | 1.1738421 | 13.3150439 | 11.5042001 | 3 | 0.0092897 |
bpi_antisocialT1_2 | female,male | TRUE | 3.8539227 | 13.1405366 | 10.5319649 | 22.6731667 | 2.1460773 | 3 | 0.5426474 |
bpi_antisocialT1_3 | female,male | TRUE | 1.4534865 | 10.7401004 | 8.1315287 | 20.2727305 | 4.5465135 | 3 | 0.2081787 |
bpi_antisocialT1_4 | female,male | TRUE | -26.4683563 | -17.1817424 | -19.7903141 | -7.6491123 | 32.4683563 | 3 | 0.0000004 |
bpi_antisocialT1_5 | female,male | TRUE | 0.0000966 | 0.0000966 | 0.0000966 | 0.0000966 | -0.0000966 | 0 | NaN |
bpi_antisocialT1_6 | female,male | TRUE | -9.9043878 | -0.6177739 | -3.2263456 | 8.9148562 | 15.9043878 | 3 | 0.0011863 |
bpi_antisocialT1_7 | female,male | TRUE | -16.5941405 | -7.3075266 | -9.9160983 | 2.2251035 | 22.5941405 | 3 | 0.0000491 |
Estimates of DIF after resolving item 5 are in Table 16.1. Of the remaining DIF, item 4 appears to have the largest DIF. If we deem item 4 to show non-negligible DIF, we would address it using one of the three approaches above and then see which items continue to show the largest DIF, address it if necessary, then identify the remaining DIF, etc.
16.10 Measurement/Factorial Invariance
Before making comparisons across groups in terms of associations between constructs or their level on the construct, it is important to establish measurement invariance (also called factorial invariance) across the groups (Millsap, 2011). Tests of measurement invariance are equivalent to tests of differential item functioning. Measurement invariance can help provide greater confidence that the measure functions equivalently across groups, that is, the items have the same strength of association with the latent factor, and the latent factor is on the same metric (i.e., the items have the same level when accounting for the latent factor). For instance, If you observe differences between groups in level of depression without establishing measurement invariance across the groups, you do not know whether the differences observed reflect true group-related differences in depression or differences in the functioning of the measure across the groups.
Measurement invariance can be tested using many methods, including confirmatory factor analysis and IRT. CFA approaches to testing measurement invariance include multi-group CFA (MGCFA), multiple-indicator, multiple-causes (MIMIC) models, moderated nonlinear factor analysis (MNLFA), score-based tests (T. Wang et al., 2014), and the alignment method (Lai, 2021). The IRT approach to testing measurement (non-)invariance is to examine whether items show differential item functioning.
The tests of measurement invariance in confirmatory factor analysis (CFA) models were fit in the lavaan
package (Rosseel et al., 2022).
The examples were adapted from lavaan
documentation: https://lavaan.ugent.be/tutorial/groups.html (archived at https://perma.cc/2FBK-RSAH).
Procedures for testing measurement invariance are outlined in Putnick & Bornstein (2016).
In addition to nested chi-square difference tests (\(\chi^2_{\text{diff}}\)), I also demonstrate permutation procedures for testing measurement invariance, as described by Jorgensen et al. (2018).
Also, you are encouraged to read about MIMIC models (Cheng et al., 2016; W.-C. Wang et al., 2009), score-based tests (T. Wang et al., 2014), and MNLFA that allows for testing measurement invariance across continuous moderators (Bauer et al., 2020; Curran et al., 2014; N. C. Gottfredson et al., 2019).
MNLFA is implemented in the mnlfa
package (Robitzsch, 2019).
You can also generate syntax for conducting MNLFA in Mplus software (Muthén & Muthén, 2019) using the aMNLFA
package (V. Cole et al., 2018).
The alignment method allows many groups to be compared (Han et al., 2019).
Model fit of nested models can be compared with a chi-square difference test, as a way of testing measurement invariance. In this approach to testing measurement invariance, first model fit is evaluated in a configural invariance model, in which the same number of factors is specified in each group, and which indicators load on which factors are the same in each group. Then, successive constraints are made across groups, including constraining factor loadings, intercepts, and residuals across groups. The metric (“weak factorial”) invariance model is similar to the configural invariance model, but it constrains the factor loadings to be the same across groups. The scalar (“strong factorial”) invariance model keeps the constraints of the metric invariance model, but it also constrains the intercepts to be the same across groups. The residual (“strict factorial”) invariance model keeps the constraints of the scalar invariance model, but it also constrains the residuals to be the same across groups. The fit of each constrained model is compared to the previous model without such constraints. That is, the fit of the metric invariance model is compared to the fit of the configural invariance model, the fit of the scalar invariance model is compared to the fit of the metric invariance model, and the fit of the residual invariance model is compared to the fit of the scalar invariance model.
The chi-square difference test is sensitive to sample size, and trivial differences in fit can be detected with large samples (Cheung & Rensvold, 2002). As a result, researchers also recommend examining change in additional criteria, including CFI, RMSEA, and SRMR (F. F. Chen, 2007). Cheung & Rensvold (2002) recommend a cutoff of \(\Delta \text{CFI} \geq -.01\) for identifying measurement non-invariance. F. F. Chen (2007) recommends the following cutoffs for identifying measurement non-invariance:
- with a small sample size (total \(N \leq 300\)):
- testing invariance of factor loadings:
- \(\Delta \text{CFI} \geq -.005\)
- supplemented by \(\Delta \text{RMSEA} \geq .010\) or \(\Delta \text{SRMR} \geq .025\)
- testing invariance of intercepts or residuals:
- \(\Delta \text{CFI} \geq -.005\)
- supplemented by \(\Delta \text{RMSEA} \geq .010\) or \(\Delta \text{SRMR} \geq .005\)
- testing invariance of factor loadings:
- with an adequate sample size (total \(N > 300\)):
- testing invariance of factor loadings:
- \(\Delta \text{CFI} \geq -.010\)
- supplemented by \(\Delta \text{RMSEA} \geq .015\) or \(\Delta \text{SRMR} \geq .030\)
- testing invariance of intercepts or residuals:
- \(\Delta \text{CFI} \geq -.005\)
- supplemented by \(\Delta \text{RMSEA} \geq .015\) or \(\Delta \text{SRMR} \geq .010\)
- testing invariance of factor loadings:
Little et al. (2007) suggested that researchers establish at least partial invariance of factor loadings (metric invariance) to compare covariances across groups, and at least partial invariance of intercepts (scalar invariance) to compare mean levels across groups. Partial invariance refers to invariance with some but not all indicators. So, to examine associations with other variables, invariance of at least some factor loadings would be preferable. To examine differences in level or growth, invariance of at least some factor loadings and intercepts would be preferable. Residual invariance is considered overly restrictive, and it is not generally expected that one establish residual invariance (Little, 2013).
Although measurement invariance is important to test, it is also worth noting that tests of measurement invariance and DIF rest on various fundamentally untestable assumptions related to scale setting (Raykov et al., 2020). For instance, to give the latent factor units, oftentimes researchers set one item’s factor loading to be equal across time (i.e., the marker variable). Results of factorial invariance tests can depend highly on which item is used as the anchor item for setting the scale of the latent factor (Belzak & Bauer, 2020). And subsequent tests of measurement invariance then rest on the fundamentally untestable assumption that changes in the level of the latent factor precipitates the same amount of change in the item across groups. If this scaling is not proper, however, it could lead to improper conclusions. Researchers are, therefore, not conclusively able to establish measurement invariance.
Several possible approaches may help address this. First, it can be helpful to place greater focus on degree of measurement invariance and confidence (rather than presence versus absence of measurement invariance). To the extent that non-invariance is trivial in effect size, it provides the researcher with greater confidence that they can use the measure to assess a construct in a comparable way over time. A number of studies describe how to test the effect size of measurement invariance (Gunn et al., 2020; e.g., Liu et al., 2017) or differential item functioning (Gonzalez & Pelham, 2021; e.g., Meade, 2010). Second, there may be important robustness checks. I describe each in greater detail below. One important sensitivity analysis could be to see if measurement invariance holds when using different marker variables. This would provide greater evidence that their apparent measurement invariance was not specific to one marker variable (i.e., one particular set of assumptions). A second robustness check would be to use effects coding, in which the average of items’ factor loadings is equal to 1, so the metric of the latent variable is on the metric of all of the items rather than just one item (Little et al., 2006). A third robustness check would be to use regularization, which is an alternative method to select the anchor items and to identify differential item functioning based on a machine learning technique that applies penalization to remove parameters that have little impact on model fit (Bauer et al., 2020; Belzak & Bauer, 2020).
Another issue with measurement invariance is that the null hypothesis that adding constraints across groups will not worsen fit is likely always false, because there are often at least slight differences across groups in factor loadings, intercepts, or residuals that reflect sampling variability. However, we are not interested in trivial differences across groups that reflect sampling variability. Instead, we are interested in the extent to which there are substantive, meaningful differences across the groups of large enough magnitude to be practically meaningful. Thus, it can also be helpful to consider whether the measures show approximate measurement invariance (Van De Schoot et al., 2015). For details on how to test approximate measurement invariance, see Van De Schoot et al. (2013).
When detecting measurement non-invariance in a given parameter (e.g., factor loadings, intercepts, or residuals), one can identify the specific items that show measurement non-invariance in one of two primary ways: (1) starting with a model that allows the given parameter to differ across groups, a researcher can iteratively add constraints to identify the item(s) for which measurement invariance fails, or (2) starting with a model that constrains the given parameter to be the same across groups, a researcher can iteratively remove constraints to identify the item(s) for which measurement invariance becomes established (and by process of elimination, the items for which measurement invariance does not become established).
Approaches to addressing measurement non-invariance are described in Section 16.5.1. If we identify that an item shows non-negligible non-invariance, we have three primary options (described above): (1) drop the item for both groups, (2) drop the item for one group but keep it for the other group, or (3) freely estimate the parameters (factor loadings and/or intercepts) for the item across groups.
Using the traditional chi-square difference test, tests of measurement invariance compare the model fit to a model with perfect fit. As the sample size grows larger, smaller differences in model fit will be detected as significant, thus rejecting measurement invariance even if the model fits well. So, it can also be useful to compare the model fit to a null hypothesis that the fit is poor, instead of the null hypothesis that the model is a perfect fit. Chi-square equivalence tests use the poor model fit as the null hypothesis. Thus, a significant chi-square equivalence test suggests that the equality constraints are plausible, providing support for the alternative hypothesis that the model fit is acceptable (Counsell et al., 2020).
16.10.1 Specify Models
16.10.1.1 Null Model
Fix residual variances and intercepts of manifest variables to be equal across groups.
Code
nullModel <- '
#Fix residual variances of manifest variables to be equal across groups
x1 ~~ c(psi1, psi1)*x1
x2 ~~ c(psi2, psi2)*x2
x3 ~~ c(psi3, psi3)*x3
x4 ~~ c(psi4, psi4)*x4
x5 ~~ c(psi5, psi5)*x5
x6 ~~ c(psi6, psi6)*x6
x7 ~~ c(psi7, psi7)*x7
x8 ~~ c(psi8, psi8)*x8
x9 ~~ c(psi9, psi9)*x9
#Fix intercepts of manifest variables to be equal across groups
x1 ~ c(tau1, tau1)*1
x2 ~ c(tau2, tau2)*1
x3 ~ c(tau3, tau3)*1
x4 ~ c(tau4, tau4)*1
x5 ~ c(tau5, tau5)*1
x6 ~ c(tau6, tau6)*1
x7 ~ c(tau7, tau7)*1
x8 ~ c(tau8, tau8)*1
x9 ~ c(tau9, tau9)*1
'
16.10.1.3 Configural Invariance
Specify the same number of factors in each group, and which indicators load on which factors are the same in each group.
Code
cfaModel_configuralInvariance <- '
#Factor loadings (free the factor loading of the first indicator)
visual =~ NA*x1 + x2 + x3
textual =~ NA*x4 + x5 + x6
speed =~ NA*x7 + x8 + x9
#Fix latent means to zero
visual ~ 0
textual ~ 0
speed ~ 0
#Fix latent variances to one
visual ~~ 1*visual
textual ~~ 1*textual
speed ~~ 1*speed
#Estimate covariances among latent variables
visual ~~ textual
visual ~~ speed
textual ~~ speed
#Estimate residual variances of manifest variables
x1 ~~ x1
x2 ~~ x2
x3 ~~ x3
x4 ~~ x4
x5 ~~ x5
x6 ~~ x6
x7 ~~ x7
x8 ~~ x8
x9 ~~ x9
#Free intercepts of manifest variables
x1 ~ NA*1
x2 ~ NA*1
x3 ~ NA*1
x4 ~ NA*1
x5 ~ NA*1
x6 ~ NA*1
x7 ~ NA*1
x8 ~ NA*1
x9 ~ NA*1
'
16.10.1.4 Metric (“Weak Factorial”) Invariance
Specify invariance of factor loadings across groups.
Code
cfaModel_metricInvariance <- '
#Fix factor loadings to be the same across groups
visual =~ c(lambdax1,lambdax1)*x1 + c(lambdax2,lambdax2)*x2 + c(lambdax3,lambdax3)*x3
textual =~ c(lambdax4,lambdax4)*x4 + c(lambdax5,lambdax5)*x5 + c(lambdax6,lambdax6)*x6
speed =~ c(lambdax7,lambdax7)*x7 + c(lambdax8,lambdax8)*x8 + c(lambdax9,lambdax9)*x9
#Fix latent means to zero
visual ~ 0
textual ~ 0
speed ~ 0
#Fix latent variances to one in group 1; free latent variances in group 2
visual ~~ c(1, NA)*visual
textual ~~ c(1, NA)*textual
speed ~~ c(1, NA)*speed
#Estimate covariances among latent variables
visual ~~ textual
visual ~~ speed
textual ~~ speed
#Estimate residual variances of manifest variables
x1 ~~ x1
x2 ~~ x2
x3 ~~ x3
x4 ~~ x4
x5 ~~ x5
x6 ~~ x6
x7 ~~ x7
x8 ~~ x8
x9 ~~ x9
#Free intercepts of manifest variables
x1 ~ NA*1
x2 ~ NA*1
x3 ~ NA*1
x4 ~ NA*1
x5 ~ NA*1
x6 ~ NA*1
x7 ~ NA*1
x8 ~ NA*1
x9 ~ NA*1
'
16.10.1.5 Scalar (“Strong Factorial”) Invariance
Specify invariance of factor loadings and intercepts across groups.
Code
cfaModel_scalarInvariance <- '
#Fix factor loadings to be the same across groups
visual =~ c(lambdax1,lambdax1)*x1 + c(lambdax2,lambdax2)*x2 + c(lambdax3,lambdax3)*x3
textual =~ c(lambdax4,lambdax4)*x4 + c(lambdax5,lambdax5)*x5 + c(lambdax6,lambdax6)*x6
speed =~ c(lambdax7,lambdax7)*x7 + c(lambdax8,lambdax8)*x8 + c(lambdax9,lambdax9)*x9
#Fix latent means to zero in group 1; free latent means in group 2
visual ~ c(0, NA)*1
textual ~ c(0, NA)*1
speed ~ c(0, NA)*1
#Fix latent variances to one in group 1; free latent variances in group 2
visual ~~ c(1, NA)*visual
textual ~~ c(1, NA)*textual
speed ~~ c(1, NA)*speed
#Estimate covariances among latent variables
visual ~~ textual
visual ~~ speed
textual ~~ speed
#Estimate residual variances of manifest variables
x1 ~~ x1
x2 ~~ x2
x3 ~~ x3
x4 ~~ x4
x5 ~~ x5
x6 ~~ x6
x7 ~~ x7
x8 ~~ x8
x9 ~~ x9
#Fix intercepts of manifest variables across groups
x1 ~ c(intx1, intx1)*1
x2 ~ c(intx2, intx2)*1
x3 ~ c(intx3, intx3)*1
x4 ~ c(intx4, intx4)*1
x5 ~ c(intx5, intx5)*1
x6 ~ c(intx6, intx6)*1
x7 ~ c(intx7, intx7)*1
x8 ~ c(intx8, intx8)*1
x9 ~ c(intx9, intx9)*1
'
16.10.1.6 Residual (“Strict Factorial”) Invariance
Specify invariance of factor loadings, intercepts, and residuals across groups.
Code
cfaModel_residualInvariance <- '
#Fix factor loadings to be the same across groups
visual =~ c(lambdax1,lambdax1)*x1 + c(lambdax2,lambdax2)*x2 + c(lambdax3,lambdax3)*x3
textual =~ c(lambdax4,lambdax4)*x4 + c(lambdax5,lambdax5)*x5 + c(lambdax6,lambdax6)*x6
speed =~ c(lambdax7,lambdax7)*x7 + c(lambdax8,lambdax8)*x8 + c(lambdax9,lambdax9)*x9
#Fix latent means to zero
visual ~ c(0, NA)*1
textual ~ c(0, NA)*1
speed ~ c(0, NA)*1
#Fix latent variances to one in group 1; free latent variances in group 2
visual ~~ c(1, NA)*visual
textual ~~ c(1, NA)*textual
speed ~~ c(1, NA)*speed
#Estimate covariances among latent variables
visual ~~ textual
visual ~~ speed
textual ~~ speed
#Fix residual variances of manifest variables across groups
x1 ~~ c(residx1, residx1)*x1
x2 ~~ c(residx2, residx2)*x2
x3 ~~ c(residx3, residx3)*x3
x4 ~~ c(residx4, residx4)*x4
x5 ~~ c(residx5, residx5)*x5
x6 ~~ c(residx6, residx6)*x6
x7 ~~ c(residx7, residx7)*x7
x8 ~~ c(residx8, residx8)*x8
x9 ~~ c(residx9, residx9)*x9
#Fix intercepts of manifest variables across groups
x1 ~ c(intx1, intx1)*1
x2 ~ c(intx2, intx2)*1
x3 ~ c(intx3, intx3)*1
x4 ~ c(intx4, intx4)*1
x5 ~ c(intx5, intx5)*1
x6 ~ c(intx6, intx6)*1
x7 ~ c(intx7, intx7)*1
x8 ~ c(intx8, intx8)*1
x9 ~ c(intx9, intx9)*1
'
16.10.3 Null Model
16.10.3.2 Model Summary
lavaan 0.6-19 ended normally after 39 iterations
Estimator ML
Optimization method NLMINB
Number of model parameters 36
Number of equality constraints 18
Number of observations per group:
Pasteur 156
Grant-White 145
Number of missing patterns per group:
Pasteur 9
Grant-White 9
Model Test User Model:
Standard Scaled
Test Statistic 1034.341 1001.376
Degrees of freedom 90 90
P-value (Chi-square) 0.000 0.000
Scaling correction factor 1.033
Yuan-Bentler correction (Mplus variant)
Test statistic for each group:
Pasteur 477.005 477.005
Grant-White 524.371 524.371
Model Test Baseline Model:
Test statistic 932.665 909.019
Degrees of freedom 72 72
P-value 0.000 0.000
Scaling correction factor 1.026
User Model versus Baseline Model:
Comparative Fit Index (CFI) 0.000 0.000
Tucker-Lewis Index (TLI) 0.122 0.129
Robust Comparative Fit Index (CFI) 0.000
Robust Tucker-Lewis Index (TLI) 0.125
Loglikelihood and Information Criteria:
Loglikelihood user model (H0) -4111.291 -4111.291
Scaling correction factor 0.520
for the MLR correction
Loglikelihood unrestricted model (H1) -3594.120 -3594.120
Scaling correction factor 1.034
for the MLR correction
Akaike (AIC) 8258.582 8258.582
Bayesian (BIC) 8325.310 8325.310
Sample-size adjusted Bayesian (SABIC) 8268.224 8268.224
Root Mean Square Error of Approximation:
RMSEA 0.264 0.259
90 Percent confidence interval - lower 0.250 0.245
90 Percent confidence interval - upper 0.279 0.274
P-value H_0: RMSEA <= 0.050 0.000 0.000
P-value H_0: RMSEA >= 0.080 1.000 1.000
Robust RMSEA 0.267
90 Percent confidence interval - lower 0.252
90 Percent confidence interval - upper 0.282
P-value H_0: Robust RMSEA <= 0.050 0.000
P-value H_0: Robust RMSEA >= 0.080 1.000
Standardized Root Mean Square Residual:
SRMR 0.284 0.284
Parameter Estimates:
Standard errors Sandwich
Information bread Observed
Observed information based on Hessian
Group 1 [Pasteur]:
Intercepts:
Estimate Std.Err z-value P(>|z|) Std.lv Std.all
x1 (tau1) 4.935 0.067 73.255 0.000 4.935 4.236
x2 (tau2) 6.085 0.068 89.601 0.000 6.085 5.173
x3 (tau3) 2.261 0.066 34.411 0.000 2.261 2.000
x4 (tau4) 3.050 0.067 45.313 0.000 3.050 2.634
x5 (tau5) 4.332 0.074 58.509 0.000 4.332 3.389
x6 (tau6) 2.195 0.063 34.800 0.000 2.195 2.013
x7 (tau7) 4.196 0.063 66.358 0.000 4.196 3.850
x8 (tau8) 5.524 0.059 94.261 0.000 5.524 5.451
x9 (tau9) 5.378 0.059 91.869 0.000 5.378 5.322
Variances:
Estimate Std.Err z-value P(>|z|) Std.lv Std.all
x1 (psi1) 1.357 0.120 11.283 0.000 1.357 1.000
x2 (psi2) 1.384 0.123 11.273 0.000 1.384 1.000
x3 (psi3) 1.278 0.077 16.500 0.000 1.278 1.000
x4 (psi4) 1.341 0.114 11.780 0.000 1.341 1.000
x5 (psi5) 1.634 0.116 14.144 0.000 1.634 1.000
x6 (psi6) 1.189 0.116 10.243 0.000 1.189 1.000
x7 (psi7) 1.188 0.090 13.206 0.000 1.188 1.000
x8 (psi8) 1.027 0.106 9.674 0.000 1.027 1.000
x9 (psi9) 1.021 0.090 11.389 0.000 1.021 1.000
Group 2 [Grant-White]:
Intercepts:
Estimate Std.Err z-value P(>|z|) Std.lv Std.all
x1 (tau1) 4.935 0.067 73.255 0.000 4.935 4.236
x2 (tau2) 6.085 0.068 89.601 0.000 6.085 5.173
x3 (tau3) 2.261 0.066 34.411 0.000 2.261 2.000
x4 (tau4) 3.050 0.067 45.313 0.000 3.050 2.634
x5 (tau5) 4.332 0.074 58.509 0.000 4.332 3.389
x6 (tau6) 2.195 0.063 34.800 0.000 2.195 2.013
x7 (tau7) 4.196 0.063 66.358 0.000 4.196 3.850
x8 (tau8) 5.524 0.059 94.261 0.000 5.524 5.451
x9 (tau9) 5.378 0.059 91.869 0.000 5.378 5.322
Variances:
Estimate Std.Err z-value P(>|z|) Std.lv Std.all
x1 (psi1) 1.357 0.120 11.283 0.000 1.357 1.000
x2 (psi2) 1.384 0.123 11.273 0.000 1.384 1.000
x3 (psi3) 1.278 0.077 16.500 0.000 1.278 1.000
x4 (psi4) 1.341 0.114 11.780 0.000 1.341 1.000
x5 (psi5) 1.634 0.116 14.144 0.000 1.634 1.000
x6 (psi6) 1.189 0.116 10.243 0.000 1.189 1.000
x7 (psi7) 1.188 0.090 13.206 0.000 1.188 1.000
x8 (psi8) 1.027 0.106 9.674 0.000 1.027 1.000
x9 (psi9) 1.021 0.090 11.389 0.000 1.021 1.000
16.10.4 Configural Invariance
Specify the same number of factors in each group, and which indicators load on which factors are the same in each group.
16.10.4.1 Model Syntax
Code
## LOADINGS:
visual =~ c(NA, NA)*x1 + c(lambda.1_1.g1, lambda.1_1.g2)*x1
visual =~ c(NA, NA)*x2 + c(lambda.2_1.g1, lambda.2_1.g2)*x2
visual =~ c(NA, NA)*x3 + c(lambda.3_1.g1, lambda.3_1.g2)*x3
textual =~ c(NA, NA)*x4 + c(lambda.4_2.g1, lambda.4_2.g2)*x4
textual =~ c(NA, NA)*x5 + c(lambda.5_2.g1, lambda.5_2.g2)*x5
textual =~ c(NA, NA)*x6 + c(lambda.6_2.g1, lambda.6_2.g2)*x6
speed =~ c(NA, NA)*x7 + c(lambda.7_3.g1, lambda.7_3.g2)*x7
speed =~ c(NA, NA)*x8 + c(lambda.8_3.g1, lambda.8_3.g2)*x8
speed =~ c(NA, NA)*x9 + c(lambda.9_3.g1, lambda.9_3.g2)*x9
## INTERCEPTS:
x1 ~ c(NA, NA)*1 + c(nu.1.g1, nu.1.g2)*1
x2 ~ c(NA, NA)*1 + c(nu.2.g1, nu.2.g2)*1
x3 ~ c(NA, NA)*1 + c(nu.3.g1, nu.3.g2)*1
x4 ~ c(NA, NA)*1 + c(nu.4.g1, nu.4.g2)*1
x5 ~ c(NA, NA)*1 + c(nu.5.g1, nu.5.g2)*1
x6 ~ c(NA, NA)*1 + c(nu.6.g1, nu.6.g2)*1
x7 ~ c(NA, NA)*1 + c(nu.7.g1, nu.7.g2)*1
x8 ~ c(NA, NA)*1 + c(nu.8.g1, nu.8.g2)*1
x9 ~ c(NA, NA)*1 + c(nu.9.g1, nu.9.g2)*1
## UNIQUE-FACTOR VARIANCES:
x1 ~~ c(NA, NA)*x1 + c(theta.1_1.g1, theta.1_1.g2)*x1
x2 ~~ c(NA, NA)*x2 + c(theta.2_2.g1, theta.2_2.g2)*x2
x3 ~~ c(NA, NA)*x3 + c(theta.3_3.g1, theta.3_3.g2)*x3
x4 ~~ c(NA, NA)*x4 + c(theta.4_4.g1, theta.4_4.g2)*x4
x5 ~~ c(NA, NA)*x5 + c(theta.5_5.g1, theta.5_5.g2)*x5
x6 ~~ c(NA, NA)*x6 + c(theta.6_6.g1, theta.6_6.g2)*x6
x7 ~~ c(NA, NA)*x7 + c(theta.7_7.g1, theta.7_7.g2)*x7
x8 ~~ c(NA, NA)*x8 + c(theta.8_8.g1, theta.8_8.g2)*x8
x9 ~~ c(NA, NA)*x9 + c(theta.9_9.g1, theta.9_9.g2)*x9
## LATENT MEANS/INTERCEPTS:
visual ~ c(0, 0)*1 + c(alpha.1.g1, alpha.1.g2)*1
textual ~ c(0, 0)*1 + c(alpha.2.g1, alpha.2.g2)*1
speed ~ c(0, 0)*1 + c(alpha.3.g1, alpha.3.g2)*1
## COMMON-FACTOR VARIANCES:
visual ~~ c(1, 1)*visual + c(psi.1_1.g1, psi.1_1.g2)*visual
textual ~~ c(1, 1)*textual + c(psi.2_2.g1, psi.2_2.g2)*textual
speed ~~ c(1, 1)*speed + c(psi.3_3.g1, psi.3_3.g2)*speed
## COMMON-FACTOR COVARIANCES:
visual ~~ c(NA, NA)*textual + c(psi.2_1.g1, psi.2_1.g2)*textual
visual ~~ c(NA, NA)*speed + c(psi.3_1.g1, psi.3_1.g2)*speed
textual ~~ c(NA, NA)*speed + c(psi.3_2.g1, psi.3_2.g2)*speed
16.10.4.1.1 Summary of Model Features
This lavaan model syntax specifies a CFA with 9 manifest indicators of 3 common factor(s).
To identify the location and scale of each common factor, the factor means and variances were fixed to 0 and 1, respectively, unless equality constraints on measurement parameters allow them to be freed.
Pattern matrix indicating num(eric), ord(ered), and lat(ent) indicators per factor:
visual textual speed
x1 num
x2 num
x3 num
x4 num
x5 num
x6 num
x7 num
x8 num
x9 num
This model hypothesizes only configural invariance.
16.10.4.3 Model Summary
Code
lavaan 0.6-19 ended normally after 64 iterations
Estimator ML
Optimization method NLMINB
Number of model parameters 60
Number of observations per group:
Pasteur 156
Grant-White 145
Number of missing patterns per group:
Pasteur 9
Grant-White 9
Model Test User Model:
Standard Scaled
Test Statistic 109.425 114.970
Degrees of freedom 48 48
P-value (Chi-square) 0.000 0.000
Scaling correction factor 0.952
Yuan-Bentler correction (Mplus variant)
Test statistic for each group:
Pasteur 63.000 63.000
Grant-White 51.970 51.970
Model Test Baseline Model:
Test statistic 932.665 909.019
Degrees of freedom 72 72
P-value 0.000 0.000
Scaling correction factor 1.026
User Model versus Baseline Model:
Comparative Fit Index (CFI) 0.929 0.920
Tucker-Lewis Index (TLI) 0.893 0.880
Robust Comparative Fit Index (CFI) 0.929
Robust Tucker-Lewis Index (TLI) 0.893
Loglikelihood and Information Criteria:
Loglikelihood user model (H0) -3648.833 -3648.833
Scaling correction factor 1.100
for the MLR correction
Loglikelihood unrestricted model (H1) -3594.120 -3594.120
Scaling correction factor 1.034
for the MLR correction
Akaike (AIC) 7417.666 7417.666
Bayesian (BIC) 7640.092 7640.092
Sample-size adjusted Bayesian (SABIC) 7449.806 7449.806
Root Mean Square Error of Approximation:
RMSEA 0.092 0.096
90 Percent confidence interval - lower 0.069 0.073
90 Percent confidence interval - upper 0.115 0.120
P-value H_0: RMSEA <= 0.050 0.002 0.001
P-value H_0: RMSEA >= 0.080 0.821 0.883
Robust RMSEA 0.093
90 Percent confidence interval - lower 0.070
90 Percent confidence interval - upper 0.117
P-value H_0: Robust RMSEA <= 0.050 0.002
P-value H_0: Robust RMSEA >= 0.080 0.833
Standardized Root Mean Square Residual:
SRMR 0.066 0.066
Parameter Estimates:
Standard errors Sandwich
Information bread Observed
Observed information based on Hessian
Group 1 [Pasteur]:
Latent Variables:
Estimate Std.Err z-value P(>|z|) Std.lv Std.all
visual =~
x1 (l.1_) 0.999 0.172 5.810 0.000 0.999 0.846
x2 (l.2_) 0.443 0.147 3.022 0.003 0.443 0.361
x3 (l.3_) 0.624 0.134 4.662 0.000 0.624 0.541
textual =~
x4 (l.4_) 0.922 0.085 10.830 0.000 0.922 0.811
x5 (l.5_) 1.126 0.072 15.723 0.000 1.126 0.864
x6 (l.6_) 0.833 0.081 10.242 0.000 0.833 0.843
speed =~
x7 (l.7_) 0.570 0.119 4.790 0.000 0.570 0.527
x8 (l.8_) 0.676 0.110 6.133 0.000 0.676 0.688
x9 (l.9_) 0.587 0.118 4.998 0.000 0.587 0.589
Covariances:
Estimate Std.Err z-value P(>|z|) Std.lv Std.all
visual ~~
textul (p.2_) 0.473 0.095 4.951 0.000 0.473 0.473
speed (p.3_1) 0.319 0.148 2.150 0.032 0.319 0.319
textual ~~
speed (p.3_2) 0.311 0.100 3.099 0.002 0.311 0.311
Intercepts:
Estimate Std.Err z-value P(>|z|) Std.lv Std.all
.x1 (n.1.) 4.947 0.095 52.269 0.000 4.947 4.191
.x2 (n.2.) 5.984 0.098 60.949 0.000 5.984 4.880
.x3 (n.3.) 2.510 0.093 26.912 0.000 2.510 2.178
.x4 (n.4.) 2.814 0.091 30.873 0.000 2.814 2.476
.x5 (n.5.) 4.002 0.105 38.256 0.000 4.002 3.070
.x6 (n.6.) 1.929 0.079 24.376 0.000 1.929 1.953
.x7 (n.7.) 4.439 0.087 51.126 0.000 4.439 4.104
.x8 (n.8.) 5.563 0.079 70.133 0.000 5.563 5.663
.x9 (n.9.) 5.426 0.080 67.537 0.000 5.426 5.443
visual (a.1.) 0.000 0.000 0.000
textual (a.2.) 0.000 0.000 0.000
speed (a.3.) 0.000 0.000 0.000
Variances:
Estimate Std.Err z-value P(>|z|) Std.lv Std.all
.x1 (t.1_) 0.396 0.298 1.330 0.183 0.396 0.284
.x2 (t.2_) 1.308 0.175 7.482 0.000 1.308 0.870
.x3 (t.3_) 0.939 0.158 5.959 0.000 0.939 0.707
.x4 (t.4_) 0.441 0.072 6.091 0.000 0.441 0.342
.x5 (t.5_) 0.431 0.083 5.169 0.000 0.431 0.254
.x6 (t.6_) 0.282 0.056 5.063 0.000 0.282 0.289
.x7 (t.7_) 0.845 0.132 6.394 0.000 0.845 0.723
.x8 (t.8_) 0.509 0.109 4.673 0.000 0.509 0.527
.x9 (t.9_) 0.649 0.134 4.824 0.000 0.649 0.653
visual (p.1_) 1.000 1.000 1.000
textual (p.2_) 1.000 1.000 1.000
speed (p.3_) 1.000 1.000 1.000
R-Square:
Estimate
x1 0.716
x2 0.130
x3 0.293
x4 0.658
x5 0.746
x6 0.711
x7 0.277
x8 0.473
x9 0.347
Group 2 [Grant-White]:
Latent Variables:
Estimate Std.Err z-value P(>|z|) Std.lv Std.all
visual =~
x1 (l.1_) 0.781 0.128 6.099 0.000 0.781 0.679
x2 (l.2_) 0.566 0.103 5.489 0.000 0.566 0.510
x3 (l.3_) 0.717 0.100 7.165 0.000 0.717 0.690
textual =~
x4 (l.4_) 0.958 0.083 11.489 0.000 0.958 0.856
x5 (l.5_) 0.973 0.090 10.798 0.000 0.973 0.835
x6 (l.6_) 0.947 0.088 10.753 0.000 0.947 0.830
speed =~
x7 (l.7_) 0.672 0.090 7.446 0.000 0.672 0.649
x8 (l.8_) 0.833 0.112 7.447 0.000 0.833 0.796
x9 (l.9_) 0.732 0.124 5.924 0.000 0.732 0.714
Covariances:
Estimate Std.Err z-value P(>|z|) Std.lv Std.all
visual ~~
textul (p.2_) 0.545 0.096 5.648 0.000 0.545 0.545
speed (p.3_1) 0.531 0.148 3.593 0.000 0.531 0.531
textual ~~
speed (p.3_2) 0.339 0.142 2.392 0.017 0.339 0.339
Intercepts:
Estimate Std.Err z-value P(>|z|) Std.lv Std.all
.x1 (n.1.) 4.929 0.096 51.433 0.000 4.929 4.286
.x2 (n.2.) 6.198 0.092 67.052 0.000 6.198 5.587
.x3 (n.3.) 1.992 0.086 23.048 0.000 1.992 1.916
.x4 (n.4.) 3.317 0.093 35.551 0.000 3.317 2.966
.x5 (n.5.) 4.712 0.096 49.039 0.000 4.712 4.045
.x6 (n.6.) 2.466 0.094 26.150 0.000 2.466 2.163
.x7 (n.7.) 3.923 0.087 45.300 0.000 3.923 3.793
.x8 (n.8.) 5.488 0.087 63.174 0.000 5.488 5.246
.x9 (n.9.) 5.338 0.085 62.640 0.000 5.338 5.210
visual (a.1.) 0.000 0.000 0.000
textual (a.2.) 0.000 0.000 0.000
speed (a.3.) 0.000 0.000 0.000
Variances:
Estimate Std.Err z-value P(>|z|) Std.lv Std.all
.x1 (t.1_) 0.712 0.183 3.884 0.000 0.712 0.538
.x2 (t.2_) 0.911 0.143 6.364 0.000 0.911 0.740
.x3 (t.3_) 0.566 0.123 4.601 0.000 0.566 0.524
.x4 (t.4_) 0.333 0.066 5.025 0.000 0.333 0.267
.x5 (t.5_) 0.411 0.074 5.570 0.000 0.411 0.303
.x6 (t.6_) 0.404 0.078 5.182 0.000 0.404 0.311
.x7 (t.7_) 0.619 0.101 6.105 0.000 0.619 0.578
.x8 (t.8_) 0.401 0.160 2.512 0.012 0.401 0.366
.x9 (t.9_) 0.514 0.140 3.684 0.000 0.514 0.490
visual (p.1_) 1.000 1.000 1.000
textual (p.2_) 1.000 1.000 1.000
speed (p.3_) 1.000 1.000 1.000
R-Square:
Estimate
x1 0.462
x2 0.260
x3 0.476
x4 0.733
x5 0.697
x6 0.689
x7 0.422
x8 0.634
x9 0.510
Code
lavaan 0.6-19 ended normally after 64 iterations
Estimator ML
Optimization method NLMINB
Number of model parameters 60
Number of observations per group:
Pasteur 156
Grant-White 145
Number of missing patterns per group:
Pasteur 9
Grant-White 9
Model Test User Model:
Standard Scaled
Test Statistic 109.425 114.970
Degrees of freedom 48 48
P-value (Chi-square) 0.000 0.000
Scaling correction factor 0.952
Yuan-Bentler correction (Mplus variant)
Test statistic for each group:
Pasteur 63.000 63.000
Grant-White 51.970 51.970
Model Test Baseline Model:
Test statistic 932.665 909.019
Degrees of freedom 72 72
P-value 0.000 0.000
Scaling correction factor 1.026
User Model versus Baseline Model:
Comparative Fit Index (CFI) 0.929 0.920
Tucker-Lewis Index (TLI) 0.893 0.880
Robust Comparative Fit Index (CFI) 0.929
Robust Tucker-Lewis Index (TLI) 0.893
Loglikelihood and Information Criteria:
Loglikelihood user model (H0) -3648.833 -3648.833
Scaling correction factor 1.100
for the MLR correction
Loglikelihood unrestricted model (H1) -3594.120 -3594.120
Scaling correction factor 1.034
for the MLR correction
Akaike (AIC) 7417.666 7417.666
Bayesian (BIC) 7640.092 7640.092
Sample-size adjusted Bayesian (SABIC) 7449.806 7449.806
Root Mean Square Error of Approximation:
RMSEA 0.092 0.096
90 Percent confidence interval - lower 0.069 0.073
90 Percent confidence interval - upper 0.115 0.120
P-value H_0: RMSEA <= 0.050 0.002 0.001
P-value H_0: RMSEA >= 0.080 0.821 0.883
Robust RMSEA 0.093
90 Percent confidence interval - lower 0.070
90 Percent confidence interval - upper 0.117
P-value H_0: Robust RMSEA <= 0.050 0.002
P-value H_0: Robust RMSEA >= 0.080 0.833
Standardized Root Mean Square Residual:
SRMR 0.066 0.066
Parameter Estimates:
Standard errors Sandwich
Information bread Observed
Observed information based on Hessian
Group 1 [Pasteur]:
Latent Variables:
Estimate Std.Err z-value P(>|z|) Std.lv Std.all
visual =~
x1 0.999 0.172 5.810 0.000 0.999 0.846
x2 0.443 0.147 3.022 0.003 0.443 0.361
x3 0.624 0.134 4.662 0.000 0.624 0.541
textual =~
x4 0.922 0.085 10.830 0.000 0.922 0.811
x5 1.126 0.072 15.723 0.000 1.126 0.864
x6 0.833 0.081 10.242 0.000 0.833 0.843
speed =~
x7 0.570 0.119 4.790 0.000 0.570 0.527
x8 0.676 0.110 6.133 0.000 0.676 0.688
x9 0.587 0.118 4.998 0.000 0.587 0.589
Covariances:
Estimate Std.Err z-value P(>|z|) Std.lv Std.all
visual ~~
textual 0.473 0.095 4.951 0.000 0.473 0.473
speed 0.319 0.148 2.150 0.032 0.319 0.319
textual ~~
speed 0.311 0.100 3.099 0.002 0.311 0.311
Intercepts:
Estimate Std.Err z-value P(>|z|) Std.lv Std.all
visual 0.000 0.000 0.000
textual 0.000 0.000 0.000
speed 0.000 0.000 0.000
.x1 4.947 0.095 52.269 0.000 4.947 4.191
.x2 5.984 0.098 60.949 0.000 5.984 4.880
.x3 2.510 0.093 26.912 0.000 2.510 2.178
.x4 2.814 0.091 30.873 0.000 2.814 2.476
.x5 4.002 0.105 38.256 0.000 4.002 3.070
.x6 1.929 0.079 24.376 0.000 1.929 1.953
.x7 4.439 0.087 51.126 0.000 4.439 4.104
.x8 5.563 0.079 70.133 0.000 5.563 5.663
.x9 5.426 0.080 67.537 0.000 5.426 5.443
Variances:
Estimate Std.Err z-value P(>|z|) Std.lv Std.all
visual 1.000 1.000 1.000
textual 1.000 1.000 1.000
speed 1.000 1.000 1.000
.x1 0.396 0.298 1.330 0.183 0.396 0.284
.x2 1.308 0.175 7.482 0.000 1.308 0.870
.x3 0.939 0.158 5.959 0.000 0.939 0.707
.x4 0.441 0.072 6.091 0.000 0.441 0.342
.x5 0.431 0.083 5.169 0.000 0.431 0.254
.x6 0.282 0.056 5.063 0.000 0.282 0.289
.x7 0.845 0.132 6.394 0.000 0.845 0.723
.x8 0.509 0.109 4.673 0.000 0.509 0.527
.x9 0.649 0.134 4.824 0.000 0.649 0.653
R-Square:
Estimate
x1 0.716
x2 0.130
x3 0.293
x4 0.658
x5 0.746
x6 0.711
x7 0.277
x8 0.473
x9 0.347
Group 2 [Grant-White]:
Latent Variables:
Estimate Std.Err z-value P(>|z|) Std.lv Std.all
visual =~
x1 0.781 0.128 6.099 0.000 0.781 0.679
x2 0.566 0.103 5.489 0.000 0.566 0.510
x3 0.717 0.100 7.165 0.000 0.717 0.690
textual =~
x4 0.958 0.083 11.489 0.000 0.958 0.856
x5 0.973 0.090 10.798 0.000 0.973 0.835
x6 0.947 0.088 10.753 0.000 0.947 0.830
speed =~
x7 0.672 0.090 7.446 0.000 0.672 0.649
x8 0.833 0.112 7.447 0.000 0.833 0.796
x9 0.732 0.124 5.924 0.000 0.732 0.714
Covariances:
Estimate Std.Err z-value P(>|z|) Std.lv Std.all
visual ~~
textual 0.545 0.096 5.648 0.000 0.545 0.545
speed 0.531 0.148 3.593 0.000 0.531 0.531
textual ~~
speed 0.339 0.142 2.392 0.017 0.339 0.339
Intercepts:
Estimate Std.Err z-value P(>|z|) Std.lv Std.all
visual 0.000 0.000 0.000
textual 0.000 0.000 0.000
speed 0.000 0.000 0.000
.x1 4.929 0.096 51.433 0.000 4.929 4.286
.x2 6.198 0.092 67.052 0.000 6.198 5.587
.x3 1.992 0.086 23.048 0.000 1.992 1.916
.x4 3.317 0.093 35.551 0.000 3.317 2.966
.x5 4.712 0.096 49.039 0.000 4.712 4.045
.x6 2.466 0.094 26.150 0.000 2.466 2.163
.x7 3.923 0.087 45.300 0.000 3.923 3.793
.x8 5.488 0.087 63.174 0.000 5.488 5.246
.x9 5.338 0.085 62.640 0.000 5.338 5.210
Variances:
Estimate Std.Err z-value P(>|z|) Std.lv Std.all
visual 1.000 1.000 1.000
textual 1.000 1.000 1.000
speed 1.000 1.000 1.000
.x1 0.712 0.183 3.884 0.000 0.712 0.538
.x2 0.911 0.143 6.364 0.000 0.911 0.740
.x3 0.566 0.123 4.601 0.000 0.566 0.524
.x4 0.333 0.066 5.025 0.000 0.333 0.267
.x5 0.411 0.074 5.570 0.000 0.411 0.303
.x6 0.404 0.078 5.182 0.000 0.404 0.311
.x7 0.619 0.101 6.105 0.000 0.619 0.578
.x8 0.401 0.160 2.512 0.012 0.401 0.366
.x9 0.514 0.140 3.684 0.000 0.514 0.490
R-Square:
Estimate
x1 0.462
x2 0.260
x3 0.476
x4 0.733
x5 0.697
x6 0.689
x7 0.422
x8 0.634
x9 0.510
16.10.4.4 Model Fit
You can specify the null model as the baseline model using: baseline.model = nullModelFit
npar fmin
60.000 0.182
chisq df
109.425 48.000
pvalue chisq.scaled
0.000 114.970
df.scaled pvalue.scaled
48.000 0.000
chisq.scaling.factor baseline.chisq
0.952 932.665
baseline.df baseline.pvalue
72.000 0.000
baseline.chisq.scaled baseline.df.scaled
909.019 72.000
baseline.pvalue.scaled baseline.chisq.scaling.factor
0.000 1.026
cfi tli
0.929 0.893
cfi.scaled tli.scaled
0.920 0.880
cfi.robust tli.robust
0.929 0.893
nnfi rfi
0.893 0.824
nfi pnfi
0.883 0.588
ifi rni
0.931 0.929
nnfi.scaled rfi.scaled
0.880 0.810
nfi.scaled pnfi.scaled
0.874 0.582
ifi.scaled rni.scaled
0.922 0.920
nnfi.robust rni.robust
0.893 0.929
logl unrestricted.logl
-3648.833 -3594.120
aic bic
7417.666 7640.092
ntotal bic2
301.000 7449.806
scaling.factor.h1 scaling.factor.h0
1.034 1.100
rmsea rmsea.ci.lower
0.092 0.069
rmsea.ci.upper rmsea.ci.level
0.115 0.900
rmsea.pvalue rmsea.close.h0
0.002 0.050
rmsea.notclose.pvalue rmsea.notclose.h0
0.821 0.080
rmsea.scaled rmsea.ci.lower.scaled
0.096 0.073
rmsea.ci.upper.scaled rmsea.pvalue.scaled
0.120 0.001
rmsea.notclose.pvalue.scaled rmsea.robust
0.883 0.093
rmsea.ci.lower.robust rmsea.ci.upper.robust
0.070 0.117
rmsea.pvalue.robust rmsea.notclose.pvalue.robust
0.002 0.833
rmr rmr_nomean
0.081 0.089
srmr srmr_bentler
0.066 0.066
srmr_bentler_nomean crmr
0.072 0.072
crmr_nomean srmr_mplus
0.080 0.066
srmr_mplus_nomean cn_05
0.072 180.268
cn_01 gfi
203.682 0.995
agfi pgfi
0.989 0.442
mfi ecvi
0.903 0.762
Code
configuralInvarianceModelFitIndices <- fitMeasures(
configuralInvarianceModel_fit)[c(
"cfi.robust", "rmsea.robust", "srmr")]
configuralInvarianceModel_chisquare <- fitMeasures(
configuralInvarianceModel_fit)[c("chisq.scaled")]
configuralInvarianceModel_chisquareScaling <- fitMeasures(
configuralInvarianceModel_fit)[c("chisq.scaling.factor")]
configuralInvarianceModel_df <- fitMeasures(
configuralInvarianceModel_fit)[c("df.scaled")]
configuralInvarianceModel_N <- lavInspect(
configuralInvarianceModel_fit,
what = "ntotal")
16.10.4.5 Effect size of non-invariance
16.10.4.5.1 dMACS
The effect size of measurement non-invariance, as described by Nye et al. (2019), was calculated using the dmacs
package (Dueber, 2019).
$DMACS
visual textual speed
x1 0.1865718 NA NA
x2 0.2102487 NA NA
x3 0.4774264 NA NA
x4 NA 0.4450412 NA
x5 NA 0.5889006 NA
x6 NA 0.5196869 NA
x7 NA NA 0.4941585
x8 NA NA 0.1714779
x9 NA NA 0.1669673
$ItemDeltaMean
visual textual speed
x1 -0.01829609 NA NA
x2 0.21407860 NA NA
x3 -0.51808612 NA NA
x4 NA 0.5031129 NA
x5 NA 0.7100353 NA
x6 NA 0.5368256 NA
x7 NA NA -0.51547978
x8 NA NA -0.07512450
x9 NA NA -0.08770776
$MeanDiff
visual textual speed
-0.3223036 1.7499738 -0.6783120
16.10.4.6 Equivalence Test
The petersenlab
package (Petersen, 2024b) contains the equiv_chi()
function from Counsell et al. (2020) that performs an equivalence test: https://osf.io/cqu8v.
An equivalence test evaluates the null hypothesis that a model is equivalent to another model.
In this case, the equivalence test evaluates the null hypothesis that a model is equivalent to another model in terms of (poor) fit.
Here, we operationalize a mediocre-fitting model as a model whose RMSEA is .08 or greater.
So, the equivalence test evaluates whether our invariance model fits significantly better than a mediocre-fitting model.
Thus, a statistically significant p-value indicates that our invariance model fits significantly better than the mediocre-fitting model (i.e., that invariance is established), whereas a non-significant p-value indicates that our invariance model does not fit significantly better than the mediocre-fitting model (i.e., that invariance is failed).
The chi-square equivalence test is non-significant, suggesting that the model fit of the configural invariance model is not acceptable (i.e., it is not better than the mediocre-fitting model). In other words, configural invariance failed.
Code
16.10.4.7 Permutation Test
Permutation procedures for testing measurement invariance are described in Jorgensen et al. (2018). The permutation test evaluates the null hypothesis that the model fit is not worse than the best-possible fitting model. A significant p-value indicates that the model fits significanlty worse than the best-possible fitting model. A non-significant p-value indicates that the model does not fit significantly worse than the best-possible fitting model.
For reproducibility, I set the seed below.
Using the same seed will yield the same answer every time.
There is nothing special about this particular seed.
You can specify the null model as the baseline model using: baseline.model = nullModelFit
.
Warning: this code takes a while to run based on \(100\) iterations.
You can reduce the number of iterations to be faster.
Code
set.seed(52242)
configuralInvarianceTest <- permuteMeasEq(
nPermute = numPermutations,
modelType = "mgcfa",
con = configuralInvarianceModel_fit,
uncon = NULL,
AFIs = myAFIs,
moreAFIs = moreAFIs,
parallelType = "multicore", #only 'snow' works on Windows, but right now, it is throwing an error
iseed = 52242)
Omnibus p value based on parametric chi-squared difference test:
Chisq diff Df diff Pr(>Chisq)
114.97 48.00 0.00
Omnibus p values based on nonparametric permutation method:
AFI.Difference p.value
chisq 109.425 0.17
chisq.scaled 114.970 0.15
rmsea 0.092 0.17
cfi 0.929 0.15
tli 0.893 0.15
srmr 0.066 0.15
rmsea.robust 0.093 0.15
cfi.robust 0.929 0.14
tli.robust 0.893 0.14
The p-values are non-significant, indicating that the model does not fit significantly worse than the best-possible fitting model. In other words, configural invariance held.
16.10.4.8 Internal Consistency Reliability
Internal consistency reliability of items composing the latent factors, as quantified by omega (\(\omega\)) and average variance extracted (AVE), was estimated using the semTools
package (Jorgensen et al., 2021).
16.10.4.9 Path Diagram
A path diagram of the model generated using the semPlot
package (Epskamp, 2022) is in the figures below (“1” = group 1; “2” = group 2).
Code
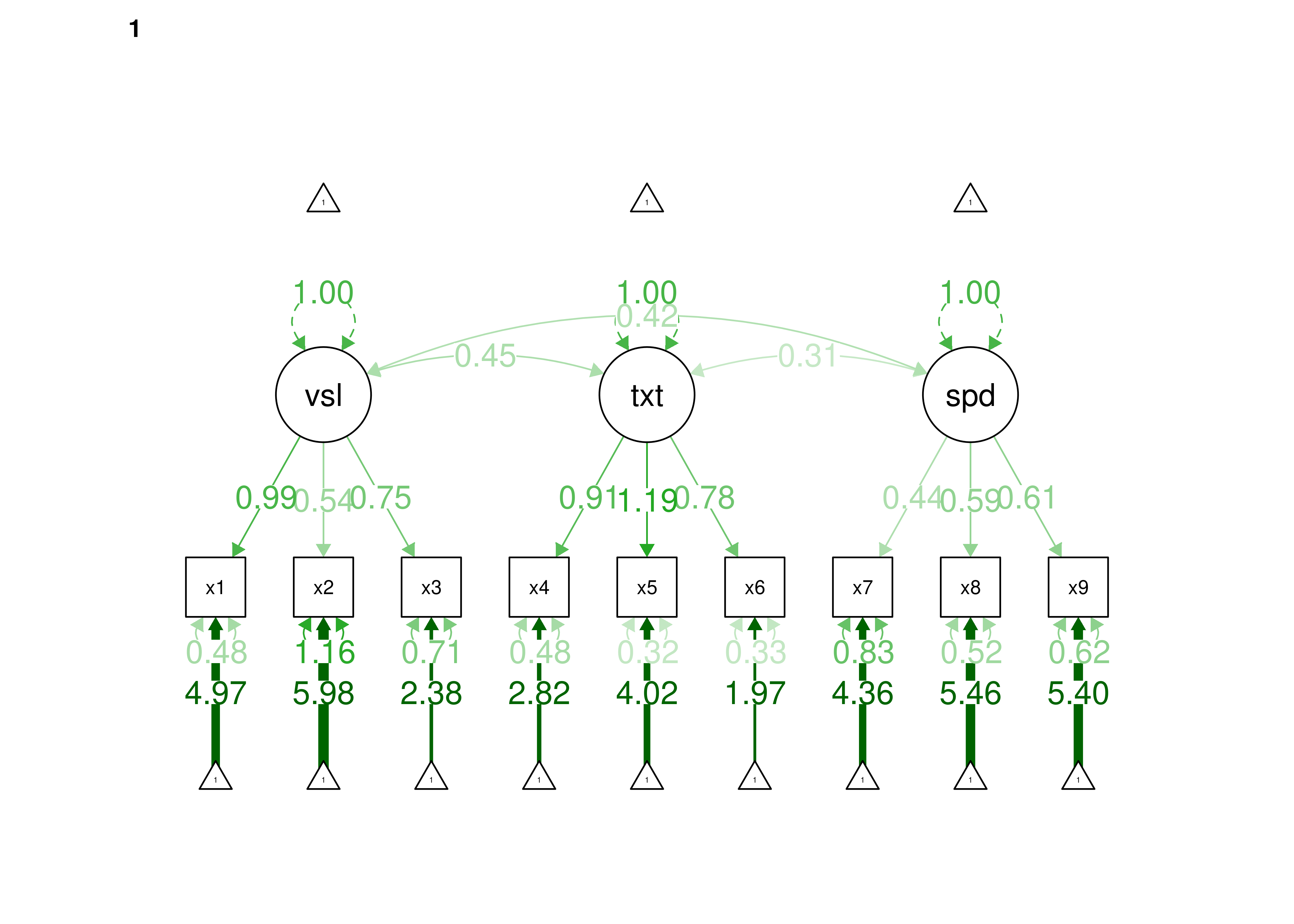
Figure 16.53: Configural Invariance Model in Confirmatory Factor Analysis (1 = Group 1; 2 = Group 2).
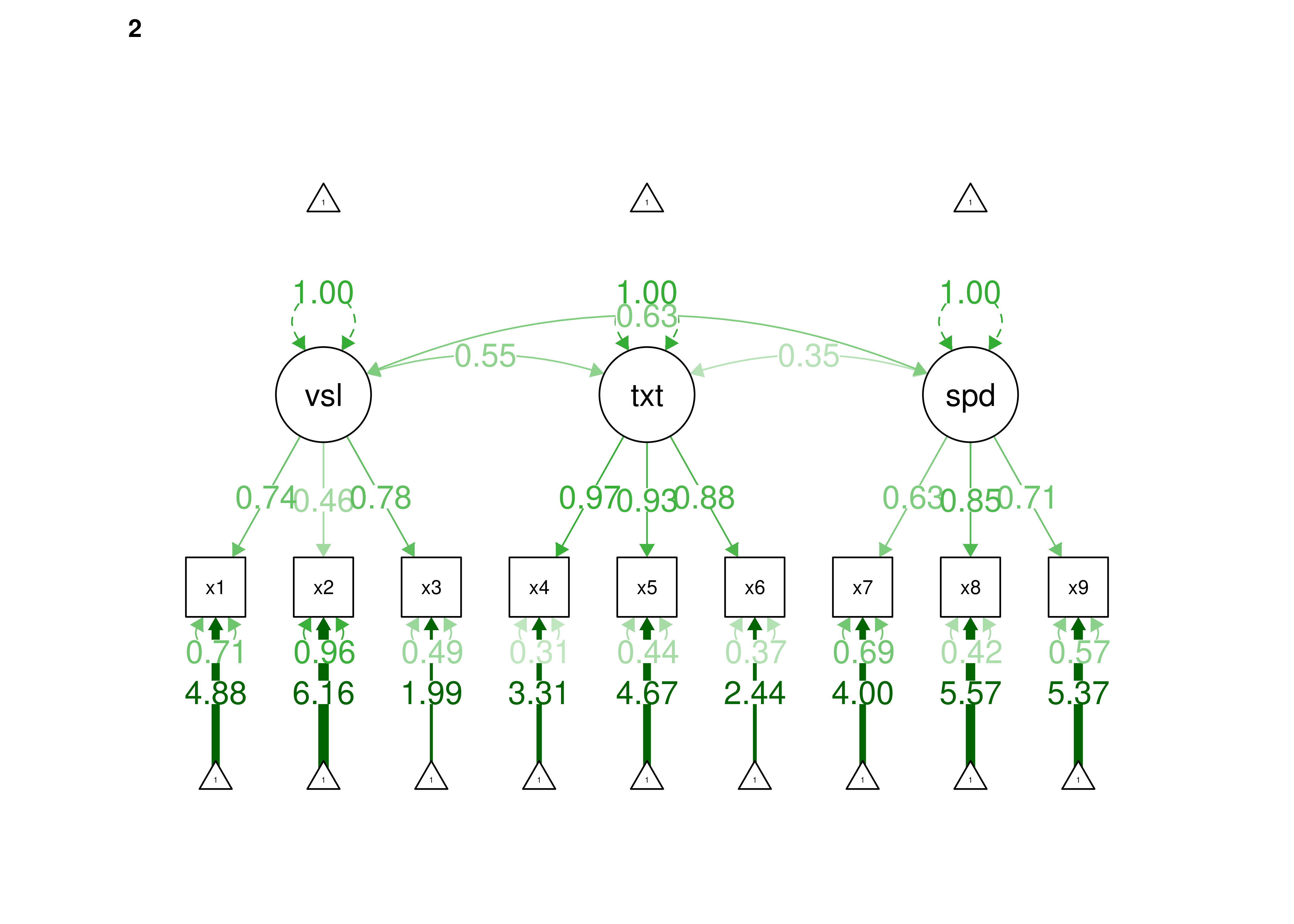
Figure 16.54: Configural Invariance Model in Confirmatory Factor Analysis (1 = Group 1; 2 = Group 2).
16.10.5 Metric (“Weak Factorial”) Invariance Model
Specify invariance of factor loadings across groups.
16.10.5.1 Model Syntax
Code
## LOADINGS:
visual =~ c(NA, NA)*x1 + c(lambda.1_1, lambda.1_1)*x1
visual =~ c(NA, NA)*x2 + c(lambda.2_1, lambda.2_1)*x2
visual =~ c(NA, NA)*x3 + c(lambda.3_1, lambda.3_1)*x3
textual =~ c(NA, NA)*x4 + c(lambda.4_2, lambda.4_2)*x4
textual =~ c(NA, NA)*x5 + c(lambda.5_2, lambda.5_2)*x5
textual =~ c(NA, NA)*x6 + c(lambda.6_2, lambda.6_2)*x6
speed =~ c(NA, NA)*x7 + c(lambda.7_3, lambda.7_3)*x7
speed =~ c(NA, NA)*x8 + c(lambda.8_3, lambda.8_3)*x8
speed =~ c(NA, NA)*x9 + c(lambda.9_3, lambda.9_3)*x9
## INTERCEPTS:
x1 ~ c(NA, NA)*1 + c(nu.1.g1, nu.1.g2)*1
x2 ~ c(NA, NA)*1 + c(nu.2.g1, nu.2.g2)*1
x3 ~ c(NA, NA)*1 + c(nu.3.g1, nu.3.g2)*1
x4 ~ c(NA, NA)*1 + c(nu.4.g1, nu.4.g2)*1
x5 ~ c(NA, NA)*1 + c(nu.5.g1, nu.5.g2)*1
x6 ~ c(NA, NA)*1 + c(nu.6.g1, nu.6.g2)*1
x7 ~ c(NA, NA)*1 + c(nu.7.g1, nu.7.g2)*1
x8 ~ c(NA, NA)*1 + c(nu.8.g1, nu.8.g2)*1
x9 ~ c(NA, NA)*1 + c(nu.9.g1, nu.9.g2)*1
## UNIQUE-FACTOR VARIANCES:
x1 ~~ c(NA, NA)*x1 + c(theta.1_1.g1, theta.1_1.g2)*x1
x2 ~~ c(NA, NA)*x2 + c(theta.2_2.g1, theta.2_2.g2)*x2
x3 ~~ c(NA, NA)*x3 + c(theta.3_3.g1, theta.3_3.g2)*x3
x4 ~~ c(NA, NA)*x4 + c(theta.4_4.g1, theta.4_4.g2)*x4
x5 ~~ c(NA, NA)*x5 + c(theta.5_5.g1, theta.5_5.g2)*x5
x6 ~~ c(NA, NA)*x6 + c(theta.6_6.g1, theta.6_6.g2)*x6
x7 ~~ c(NA, NA)*x7 + c(theta.7_7.g1, theta.7_7.g2)*x7
x8 ~~ c(NA, NA)*x8 + c(theta.8_8.g1, theta.8_8.g2)*x8
x9 ~~ c(NA, NA)*x9 + c(theta.9_9.g1, theta.9_9.g2)*x9
## LATENT MEANS/INTERCEPTS:
visual ~ c(0, 0)*1 + c(alpha.1.g1, alpha.1.g2)*1
textual ~ c(0, 0)*1 + c(alpha.2.g1, alpha.2.g2)*1
speed ~ c(0, 0)*1 + c(alpha.3.g1, alpha.3.g2)*1
## COMMON-FACTOR VARIANCES:
visual ~~ c(1, NA)*visual + c(psi.1_1.g1, psi.1_1.g2)*visual
textual ~~ c(1, NA)*textual + c(psi.2_2.g1, psi.2_2.g2)*textual
speed ~~ c(1, NA)*speed + c(psi.3_3.g1, psi.3_3.g2)*speed
## COMMON-FACTOR COVARIANCES:
visual ~~ c(NA, NA)*textual + c(psi.2_1.g1, psi.2_1.g2)*textual
visual ~~ c(NA, NA)*speed + c(psi.3_1.g1, psi.3_1.g2)*speed
textual ~~ c(NA, NA)*speed + c(psi.3_2.g1, psi.3_2.g2)*speed
16.10.5.1.1 Summary of Model Features
This lavaan model syntax specifies a CFA with 9 manifest indicators of 3 common factor(s).
To identify the location and scale of each common factor, the factor means and variances were fixed to 0 and 1, respectively, unless equality constraints on measurement parameters allow them to be freed.
Pattern matrix indicating num(eric), ord(ered), and lat(ent) indicators per factor:
visual textual speed
x1 num
x2 num
x3 num
x4 num
x5 num
x6 num
x7 num
x8 num
x9 num
The following types of parameter were constrained to equality across groups:
loadings
16.10.5.4 Model Summary
lavaan 0.6-19 ended normally after 73 iterations
Estimator ML
Optimization method NLMINB
Number of model parameters 63
Number of equality constraints 9
Number of observations per group:
Pasteur 156
Grant-White 145
Number of missing patterns per group:
Pasteur 9
Grant-White 9
Model Test User Model:
Standard Scaled
Test Statistic 116.313 117.955
Degrees of freedom 54 54
P-value (Chi-square) 0.000 0.000
Scaling correction factor 0.986
Yuan-Bentler correction (Mplus variant)
Test statistic for each group:
Pasteur 64.366 64.366
Grant-White 53.590 53.590
Model Test Baseline Model:
Test statistic 932.665 909.019
Degrees of freedom 72 72
P-value 0.000 0.000
Scaling correction factor 1.026
User Model versus Baseline Model:
Comparative Fit Index (CFI) 0.928 0.924
Tucker-Lewis Index (TLI) 0.903 0.898
Robust Comparative Fit Index (CFI) 0.928
Robust Tucker-Lewis Index (TLI) 0.905
Loglikelihood and Information Criteria:
Loglikelihood user model (H0) -3652.277 -3652.277
Scaling correction factor 0.928
for the MLR correction
Loglikelihood unrestricted model (H1) -3594.120 -3594.120
Scaling correction factor 1.034
for the MLR correction
Akaike (AIC) 7412.554 7412.554
Bayesian (BIC) 7612.738 7612.738
Sample-size adjusted Bayesian (SABIC) 7441.480 7441.480
Root Mean Square Error of Approximation:
RMSEA 0.088 0.089
90 Percent confidence interval - lower 0.066 0.067
90 Percent confidence interval - upper 0.109 0.111
P-value H_0: RMSEA <= 0.050 0.004 0.003
P-value H_0: RMSEA >= 0.080 0.730 0.757
Robust RMSEA 0.088
90 Percent confidence interval - lower 0.066
90 Percent confidence interval - upper 0.111
P-value H_0: Robust RMSEA <= 0.050 0.004
P-value H_0: Robust RMSEA >= 0.080 0.740
Standardized Root Mean Square Residual:
SRMR 0.069 0.069
Parameter Estimates:
Standard errors Sandwich
Information bread Observed
Observed information based on Hessian
Group 1 [Pasteur]:
Latent Variables:
Estimate Std.Err z-value P(>|z|) Std.lv Std.all
visual =~
x1 (l.1_) 0.885 0.122 7.258 0.000 0.885 0.759
x2 (l.2_) 0.536 0.088 6.106 0.000 0.536 0.432
x3 (l.3_) 0.707 0.087 8.148 0.000 0.707 0.608
textual =~
x4 (l.4_) 0.935 0.072 12.929 0.000 0.935 0.813
x5 (l.5_) 1.045 0.076 13.740 0.000 1.045 0.832
x6 (l.6_) 0.874 0.070 12.496 0.000 0.874 0.865
speed =~
x7 (l.7_) 0.550 0.075 7.346 0.000 0.550 0.510
x8 (l.8_) 0.675 0.087 7.784 0.000 0.675 0.686
x9 (l.9_) 0.602 0.088 6.867 0.000 0.602 0.603
Covariances:
Estimate Std.Err z-value P(>|z|) Std.lv Std.all
visual ~~
textul (p.2_) 0.473 0.105 4.484 0.000 0.473 0.473
speed (p.3_1) 0.347 0.125 2.778 0.005 0.347 0.347
textual ~~
speed (p.3_2) 0.320 0.096 3.342 0.001 0.320 0.320
Intercepts:
Estimate Std.Err z-value P(>|z|) Std.lv Std.all
.x1 (n.1.) 4.948 0.095 52.314 0.000 4.948 4.243
.x2 (n.2.) 5.984 0.098 60.949 0.000 5.984 4.823
.x3 (n.3.) 2.510 0.093 26.891 0.000 2.510 2.157
.x4 (n.4.) 2.814 0.091 30.886 0.000 2.814 2.448
.x5 (n.5.) 4.002 0.105 38.265 0.000 4.002 3.186
.x6 (n.6.) 1.929 0.079 24.377 0.000 1.929 1.907
.x7 (n.7.) 4.439 0.087 51.128 0.000 4.439 4.117
.x8 (n.8.) 5.564 0.079 70.170 0.000 5.564 5.655
.x9 (n.9.) 5.426 0.080 67.539 0.000 5.426 5.435
visual (a.1.) 0.000 0.000 0.000
textual (a.2.) 0.000 0.000 0.000
speed (a.3.) 0.000 0.000 0.000
Variances:
Estimate Std.Err z-value P(>|z|) Std.lv Std.all
.x1 (t.1_) 0.577 0.166 3.482 0.000 0.577 0.424
.x2 (t.2_) 1.252 0.162 7.718 0.000 1.252 0.813
.x3 (t.3_) 0.853 0.127 6.737 0.000 0.853 0.630
.x4 (t.4_) 0.447 0.072 6.189 0.000 0.447 0.339
.x5 (t.5_) 0.486 0.075 6.445 0.000 0.486 0.308
.x6 (t.6_) 0.258 0.057 4.499 0.000 0.258 0.252
.x7 (t.7_) 0.860 0.114 7.549 0.000 0.860 0.739
.x8 (t.8_) 0.512 0.097 5.296 0.000 0.512 0.529
.x9 (t.9_) 0.635 0.118 5.376 0.000 0.635 0.637
visual (p.1_) 1.000 1.000 1.000
textual (p.2_) 1.000 1.000 1.000
speed (p.3_) 1.000 1.000 1.000
R-Square:
Estimate
x1 0.576
x2 0.187
x3 0.370
x4 0.661
x5 0.692
x6 0.748
x7 0.261
x8 0.471
x9 0.363
Group 2 [Grant-White]:
Latent Variables:
Estimate Std.Err z-value P(>|z|) Std.lv Std.all
visual =~
x1 (l.1_) 0.885 0.122 7.258 0.000 0.844 0.723
x2 (l.2_) 0.536 0.088 6.106 0.000 0.512 0.467
x3 (l.3_) 0.707 0.087 8.148 0.000 0.675 0.655
textual =~
x4 (l.4_) 0.935 0.072 12.929 0.000 0.937 0.846
x5 (l.5_) 1.045 0.076 13.740 0.000 1.047 0.864
x6 (l.6_) 0.874 0.070 12.496 0.000 0.876 0.798
speed =~
x7 (l.7_) 0.550 0.075 7.346 0.000 0.674 0.649
x8 (l.8_) 0.675 0.087 7.784 0.000 0.826 0.790
x9 (l.9_) 0.602 0.088 6.867 0.000 0.736 0.719
Covariances:
Estimate Std.Err z-value P(>|z|) Std.lv Std.all
visual ~~
textul (p.2_) 0.520 0.134 3.867 0.000 0.544 0.544
speed (p.3_1) 0.638 0.211 3.023 0.002 0.546 0.546
textual ~~
speed (p.3_2) 0.429 0.193 2.219 0.026 0.350 0.350
Intercepts:
Estimate Std.Err z-value P(>|z|) Std.lv Std.all
.x1 (n.1.) 4.930 0.096 51.453 0.000 4.930 4.220
.x2 (n.2.) 6.198 0.092 67.063 0.000 6.198 5.654
.x3 (n.3.) 1.992 0.086 23.042 0.000 1.992 1.934
.x4 (n.4.) 3.318 0.093 35.501 0.000 3.318 2.997
.x5 (n.5.) 4.714 0.097 48.693 0.000 4.714 3.891
.x6 (n.6.) 2.467 0.094 26.165 0.000 2.467 2.246
.x7 (n.7.) 3.923 0.087 45.299 0.000 3.923 3.782
.x8 (n.8.) 5.488 0.087 63.174 0.000 5.488 5.250
.x9 (n.9.) 5.338 0.085 62.628 0.000 5.338 5.216
visual (a.1.) 0.000 0.000 0.000
textual (a.2.) 0.000 0.000 0.000
speed (a.3.) 0.000 0.000 0.000
Variances:
Estimate Std.Err z-value P(>|z|) Std.lv Std.all
.x1 (t.1_) 0.652 0.168 3.877 0.000 0.652 0.478
.x2 (t.2_) 0.940 0.145 6.473 0.000 0.940 0.782
.x3 (t.3_) 0.606 0.113 5.376 0.000 0.606 0.571
.x4 (t.4_) 0.348 0.066 5.257 0.000 0.348 0.284
.x5 (t.5_) 0.371 0.074 5.021 0.000 0.371 0.253
.x6 (t.6_) 0.439 0.076 5.738 0.000 0.439 0.364
.x7 (t.7_) 0.622 0.098 6.338 0.000 0.622 0.578
.x8 (t.8_) 0.410 0.148 2.765 0.006 0.410 0.375
.x9 (t.9_) 0.505 0.128 3.957 0.000 0.505 0.482
visual (p.1_) 0.910 0.234 3.895 0.000 1.000 1.000
textual (p.2_) 1.004 0.191 5.257 0.000 1.000 1.000
speed (p.3_) 1.498 0.357 4.201 0.000 1.000 1.000
R-Square:
Estimate
x1 0.522
x2 0.218
x3 0.429
x4 0.716
x5 0.747
x6 0.636
x7 0.422
x8 0.625
x9 0.518
Code
lavaan 0.6-19 ended normally after 73 iterations
Estimator ML
Optimization method NLMINB
Number of model parameters 63
Number of equality constraints 9
Number of observations per group:
Pasteur 156
Grant-White 145
Number of missing patterns per group:
Pasteur 9
Grant-White 9
Model Test User Model:
Standard Scaled
Test Statistic 116.313 117.955
Degrees of freedom 54 54
P-value (Chi-square) 0.000 0.000
Scaling correction factor 0.986
Yuan-Bentler correction (Mplus variant)
Test statistic for each group:
Pasteur 64.366 64.366
Grant-White 53.590 53.590
Model Test Baseline Model:
Test statistic 932.665 909.019
Degrees of freedom 72 72
P-value 0.000 0.000
Scaling correction factor 1.026
User Model versus Baseline Model:
Comparative Fit Index (CFI) 0.928 0.924
Tucker-Lewis Index (TLI) 0.903 0.898
Robust Comparative Fit Index (CFI) 0.928
Robust Tucker-Lewis Index (TLI) 0.905
Loglikelihood and Information Criteria:
Loglikelihood user model (H0) -3652.277 -3652.277
Scaling correction factor 0.928
for the MLR correction
Loglikelihood unrestricted model (H1) -3594.120 -3594.120
Scaling correction factor 1.034
for the MLR correction
Akaike (AIC) 7412.554 7412.554
Bayesian (BIC) 7612.738 7612.738
Sample-size adjusted Bayesian (SABIC) 7441.480 7441.480
Root Mean Square Error of Approximation:
RMSEA 0.088 0.089
90 Percent confidence interval - lower 0.066 0.067
90 Percent confidence interval - upper 0.109 0.111
P-value H_0: RMSEA <= 0.050 0.004 0.003
P-value H_0: RMSEA >= 0.080 0.730 0.757
Robust RMSEA 0.088
90 Percent confidence interval - lower 0.066
90 Percent confidence interval - upper 0.111
P-value H_0: Robust RMSEA <= 0.050 0.004
P-value H_0: Robust RMSEA >= 0.080 0.740
Standardized Root Mean Square Residual:
SRMR 0.069 0.069
Parameter Estimates:
Standard errors Sandwich
Information bread Observed
Observed information based on Hessian
Group 1 [Pasteur]:
Latent Variables:
Estimate Std.Err z-value P(>|z|) Std.lv Std.all
visual =~
x1 (lmb1) 0.885 0.122 7.258 0.000 0.885 0.759
x2 (lmb2) 0.536 0.088 6.106 0.000 0.536 0.432
x3 (lmb3) 0.707 0.087 8.148 0.000 0.707 0.608
textual =~
x4 (lmb4) 0.935 0.072 12.929 0.000 0.935 0.813
x5 (lmb5) 1.045 0.076 13.740 0.000 1.045 0.832
x6 (lmb6) 0.874 0.070 12.496 0.000 0.874 0.865
speed =~
x7 (lmb7) 0.550 0.075 7.346 0.000 0.550 0.510
x8 (lmb8) 0.675 0.087 7.784 0.000 0.675 0.686
x9 (lmb9) 0.602 0.088 6.867 0.000 0.602 0.603
Covariances:
Estimate Std.Err z-value P(>|z|) Std.lv Std.all
visual ~~
textual 0.473 0.105 4.484 0.000 0.473 0.473
speed 0.347 0.125 2.778 0.005 0.347 0.347
textual ~~
speed 0.320 0.096 3.342 0.001 0.320 0.320
Intercepts:
Estimate Std.Err z-value P(>|z|) Std.lv Std.all
visual 0.000 0.000 0.000
textual 0.000 0.000 0.000
speed 0.000 0.000 0.000
.x1 4.948 0.095 52.314 0.000 4.948 4.243
.x2 5.984 0.098 60.949 0.000 5.984 4.823
.x3 2.510 0.093 26.891 0.000 2.510 2.157
.x4 2.814 0.091 30.886 0.000 2.814 2.448
.x5 4.002 0.105 38.265 0.000 4.002 3.186
.x6 1.929 0.079 24.377 0.000 1.929 1.907
.x7 4.439 0.087 51.128 0.000 4.439 4.117
.x8 5.564 0.079 70.170 0.000 5.564 5.655
.x9 5.426 0.080 67.539 0.000 5.426 5.435
Variances:
Estimate Std.Err z-value P(>|z|) Std.lv Std.all
visual 1.000 1.000 1.000
textual 1.000 1.000 1.000
speed 1.000 1.000 1.000
.x1 0.577 0.166 3.482 0.000 0.577 0.424
.x2 1.252 0.162 7.718 0.000 1.252 0.813
.x3 0.853 0.127 6.737 0.000 0.853 0.630
.x4 0.447 0.072 6.189 0.000 0.447 0.339
.x5 0.486 0.075 6.445 0.000 0.486 0.308
.x6 0.258 0.057 4.499 0.000 0.258 0.252
.x7 0.860 0.114 7.549 0.000 0.860 0.739
.x8 0.512 0.097 5.296 0.000 0.512 0.529
.x9 0.635 0.118 5.376 0.000 0.635 0.637
R-Square:
Estimate
x1 0.576
x2 0.187
x3 0.370
x4 0.661
x5 0.692
x6 0.748
x7 0.261
x8 0.471
x9 0.363
Group 2 [Grant-White]:
Latent Variables:
Estimate Std.Err z-value P(>|z|) Std.lv Std.all
visual =~
x1 (lmb1) 0.885 0.122 7.258 0.000 0.844 0.723
x2 (lmb2) 0.536 0.088 6.106 0.000 0.512 0.467
x3 (lmb3) 0.707 0.087 8.148 0.000 0.675 0.655
textual =~
x4 (lmb4) 0.935 0.072 12.929 0.000 0.937 0.846
x5 (lmb5) 1.045 0.076 13.740 0.000 1.047 0.864
x6 (lmb6) 0.874 0.070 12.496 0.000 0.876 0.798
speed =~
x7 (lmb7) 0.550 0.075 7.346 0.000 0.674 0.649
x8 (lmb8) 0.675 0.087 7.784 0.000 0.826 0.790
x9 (lmb9) 0.602 0.088 6.867 0.000 0.736 0.719
Covariances:
Estimate Std.Err z-value P(>|z|) Std.lv Std.all
visual ~~
textual 0.520 0.134 3.867 0.000 0.544 0.544
speed 0.638 0.211 3.023 0.002 0.546 0.546
textual ~~
speed 0.429 0.193 2.219 0.026 0.350 0.350
Intercepts:
Estimate Std.Err z-value P(>|z|) Std.lv Std.all
visual 0.000 0.000 0.000
textual 0.000 0.000 0.000
speed 0.000 0.000 0.000
.x1 4.930 0.096 51.453 0.000 4.930 4.220
.x2 6.198 0.092 67.063 0.000 6.198 5.654
.x3 1.992 0.086 23.042 0.000 1.992 1.934
.x4 3.318 0.093 35.501 0.000 3.318 2.997
.x5 4.714 0.097 48.693 0.000 4.714 3.891
.x6 2.467 0.094 26.165 0.000 2.467 2.246
.x7 3.923 0.087 45.299 0.000 3.923 3.782
.x8 5.488 0.087 63.174 0.000 5.488 5.250
.x9 5.338 0.085 62.628 0.000 5.338 5.216
Variances:
Estimate Std.Err z-value P(>|z|) Std.lv Std.all
visual 0.910 0.234 3.895 0.000 1.000 1.000
textual 1.004 0.191 5.257 0.000 1.000 1.000
speed 1.498 0.357 4.201 0.000 1.000 1.000
.x1 0.652 0.168 3.877 0.000 0.652 0.478
.x2 0.940 0.145 6.473 0.000 0.940 0.782
.x3 0.606 0.113 5.376 0.000 0.606 0.571
.x4 0.348 0.066 5.257 0.000 0.348 0.284
.x5 0.371 0.074 5.021 0.000 0.371 0.253
.x6 0.439 0.076 5.738 0.000 0.439 0.364
.x7 0.622 0.098 6.338 0.000 0.622 0.578
.x8 0.410 0.148 2.765 0.006 0.410 0.375
.x9 0.505 0.128 3.957 0.000 0.505 0.482
R-Square:
Estimate
x1 0.522
x2 0.218
x3 0.429
x4 0.716
x5 0.747
x6 0.636
x7 0.422
x8 0.625
x9 0.518
16.10.5.5 Model Fit
You can specify the null model as the baseline model using: baseline.model = nullModelFit
npar fmin
54.000 0.193
chisq df
116.313 54.000
pvalue chisq.scaled
0.000 117.955
df.scaled pvalue.scaled
54.000 0.000
chisq.scaling.factor baseline.chisq
0.986 932.665
baseline.df baseline.pvalue
72.000 0.000
baseline.chisq.scaled baseline.df.scaled
909.019 72.000
baseline.pvalue.scaled baseline.chisq.scaling.factor
0.000 1.026
cfi tli
0.928 0.903
cfi.scaled tli.scaled
0.924 0.898
cfi.robust tli.robust
0.928 0.905
nnfi rfi
0.903 0.834
nfi pnfi
0.875 0.656
ifi rni
0.929 0.928
nnfi.scaled rfi.scaled
0.898 0.827
nfi.scaled pnfi.scaled
0.870 0.653
ifi.scaled rni.scaled
0.925 0.924
nnfi.robust rni.robust
0.905 0.928
logl unrestricted.logl
-3652.277 -3594.120
aic bic
7412.554 7612.738
ntotal bic2
301.000 7441.480
scaling.factor.h1 scaling.factor.h0
1.034 0.928
rmsea rmsea.ci.lower
0.088 0.066
rmsea.ci.upper rmsea.ci.level
0.109 0.900
rmsea.pvalue rmsea.close.h0
0.004 0.050
rmsea.notclose.pvalue rmsea.notclose.h0
0.730 0.080
rmsea.scaled rmsea.ci.lower.scaled
0.089 0.067
rmsea.ci.upper.scaled rmsea.pvalue.scaled
0.111 0.003
rmsea.notclose.pvalue.scaled rmsea.robust
0.757 0.088
rmsea.ci.lower.robust rmsea.ci.upper.robust
0.066 0.111
rmsea.pvalue.robust rmsea.notclose.pvalue.robust
0.004 0.740
rmr rmr_nomean
0.085 0.093
srmr srmr_bentler
0.069 0.069
srmr_bentler_nomean crmr
0.075 0.073
crmr_nomean srmr_mplus
0.081 0.072
srmr_mplus_nomean cn_05
0.074 187.721
cn_01 gfi
210.793 0.995
agfi pgfi
0.989 0.497
mfi ecvi
0.902 0.745
Code
metricInvarianceModelFitIndices <- fitMeasures(
metricInvarianceModel_fit)[c(
"cfi.robust", "rmsea.robust", "srmr")]
metricInvarianceModel_chisquare <- fitMeasures(
metricInvarianceModel_fit)[c("chisq.scaled")]
metricInvarianceModel_chisquareScaling <- fitMeasures(
metricInvarianceModel_fit)[c("chisq.scaling.factor")]
metricInvarianceModel_df <- fitMeasures(
metricInvarianceModel_fit)[c("df.scaled")]
metricInvarianceModel_N <- lavInspect(
metricInvarianceModel_fit, what = "ntotal")
16.10.5.6 Compare Model Fit
16.10.5.6.1 Nested Model (\(\chi^2\)) Difference Test
The configural invariance model and the metric (“weak factorial”) invariance model are considered “nested” models. The metric invariance model is nested within the configural invariance model because the configural invariance model includes all of the terms of the metric invariance model along with additional terms. Model fit of nested models can be compared with a chi-square difference test, also known as a likelihood ratio test or deviance test. A significant chi-square difference test would indicate that the simplified model with additional constraints (the metric invariance model) is significantly worse fitting than the more complex model that has fewer constraints (the configural invariance model).
The metric invariance model did not fit significantly worse than the configural invariance model, so metric invariance held. This provides evidence of measurement invariance of factor loadings across groups. Measurement invariance of factor loadings across groups provides support for examining whether the groups show different associations of the factor with other constructs (Little et al., 2007).
The petersenlab
package (Petersen, 2024b) contains the satorraBentlerScaledChiSquareDifferenceTestStatistic()
function that performs a Satorra-Bentler scaled chi-square difference test:
Below is a Satorra-Bentler scaled chi-square difference test, where \(c0\) and \(c1\) are the scaling correction factor for the nested model and comparison model, respectively; \(d0\) and \(d1\) are the degrees of freedom of the nested model and comparison model, respectively; and \(T0\) and \(T1\) are the chi-square values of the nested model and comparison model, respectively.
Code
metricInvarianceModel_chisquareDiff <-
satorraBentlerScaledChiSquareDifferenceTestStatistic(
T0 = metricInvarianceModel_chisquare,
c0 = metricInvarianceModel_chisquareScaling,
d0 = metricInvarianceModel_df,
T1 = configuralInvarianceModel_chisquare,
c1 = configuralInvarianceModel_chisquareScaling,
d1 = configuralInvarianceModel_df)
metricInvarianceModel_chisquareDiff
chisq.scaled
5.464443
16.10.5.6.3 Score-Based Test
Score-based tests of measurement invariance are implemented using the strucchange
package and are described by T. Wang et al. (2014).
lambda.1_1 lambda.2_1 lambda.3_1 lambda.4_2 lambda.5_2 lambda.6_2
0.885 0.536 0.707 0.935 1.045 0.874
lambda.7_3 lambda.8_3 lambda.9_3 nu.1.g1 nu.2.g1 nu.3.g1
0.550 0.675 0.602 4.948 5.984 2.510
nu.4.g1 nu.5.g1 nu.6.g1 nu.7.g1 nu.8.g1 nu.9.g1
2.814 4.002 1.929 4.439 5.564 5.426
theta.1_1.g1 theta.2_2.g1 theta.3_3.g1 theta.4_4.g1 theta.5_5.g1 theta.6_6.g1
0.577 1.252 0.853 0.447 0.486 0.258
theta.7_7.g1 theta.8_8.g1 theta.9_9.g1 psi.2_1.g1 psi.3_1.g1 psi.3_2.g1
0.860 0.512 0.635 0.473 0.347 0.320
lambda.1_1 lambda.2_1 lambda.3_1 lambda.4_2 lambda.5_2 lambda.6_2
0.885 0.536 0.707 0.935 1.045 0.874
lambda.7_3 lambda.8_3 lambda.9_3 nu.1.g2 nu.2.g2 nu.3.g2
0.550 0.675 0.602 4.930 6.198 1.992
nu.4.g2 nu.5.g2 nu.6.g2 nu.7.g2 nu.8.g2 nu.9.g2
3.318 4.714 2.467 3.923 5.488 5.338
theta.1_1.g2 theta.2_2.g2 theta.3_3.g2 theta.4_4.g2 theta.5_5.g2 theta.6_6.g2
0.652 0.940 0.606 0.348 0.371 0.439
theta.7_7.g2 theta.8_8.g2 theta.9_9.g2 psi.1_1.g2 psi.2_2.g2 psi.3_3.g2
0.622 0.410 0.505 0.910 1.004 1.498
psi.2_1.g2 psi.3_1.g2 psi.3_2.g2
0.520 0.638 0.429
lambda.1_1 lambda.2_1 lambda.3_1 lambda.4_2 lambda.5_2 lambda.6_2 lambda.7_3
0.8850052 0.5363099 0.7072357 0.9347277 1.0449934 0.8743059 0.5504042
lambda.8_3 lambda.9_3
0.6751277 0.6015120
Code
M-fluctuation test
data: metricInvarianceModel_fit
f(efp) = 5.0601, p-value = 0.829
A score-based test and expected parameter change (EPC) estimates (Oberski, 2014; Oberski et al., 2015) are provided by the lavaan
package (Rosseel et al., 2022).
$test
total score test:
test X2 df p.value
1 score 6.199 9 0.72
$uni
univariate score tests:
lhs op rhs X2 df p.value
1 .p1. == .p37. 1.303 1 0.254
2 .p2. == .p38. 0.468 1 0.494
3 .p3. == .p39. 0.334 1 0.563
4 .p4. == .p40. 0.314 1 0.575
5 .p5. == .p41. 4.447 1 0.035
6 .p6. == .p42. 2.539 1 0.111
7 .p7. == .p43. 0.027 1 0.869
8 .p8. == .p44. 0.005 1 0.942
9 .p9. == .p45. 0.007 1 0.932
$epc
expected parameter changes (epc) and expected parameter values (epv):
lhs op rhs block group free label plabel est epc epv
1 visual =~ x1 1 1 1 lambda.1_1 .p1. 0.885 0.072 0.957
2 visual =~ x2 1 1 2 lambda.2_1 .p2. 0.536 -0.060 0.476
3 visual =~ x3 1 1 3 lambda.3_1 .p3. 0.707 -0.052 0.655
4 textual =~ x4 1 1 4 lambda.4_2 .p4. 0.935 -0.018 0.916
5 textual =~ x5 1 1 5 lambda.5_2 .p5. 1.045 0.081 1.126
6 textual =~ x6 1 1 6 lambda.6_2 .p6. 0.874 -0.042 0.832
7 speed =~ x7 1 1 7 lambda.7_3 .p7. 0.550 0.013 0.563
8 speed =~ x8 1 1 8 lambda.8_3 .p8. 0.675 -0.004 0.671
9 speed =~ x9 1 1 9 lambda.9_3 .p9. 0.602 -0.004 0.597
10 x1 ~1 1 1 10 nu.1.g1 .p10. 4.948 0.000 4.948
11 x2 ~1 1 1 11 nu.2.g1 .p11. 5.984 0.000 5.984
12 x3 ~1 1 1 12 nu.3.g1 .p12. 2.510 0.000 2.510
13 x4 ~1 1 1 13 nu.4.g1 .p13. 2.814 0.000 2.814
14 x5 ~1 1 1 14 nu.5.g1 .p14. 4.002 0.000 4.002
15 x6 ~1 1 1 15 nu.6.g1 .p15. 1.929 0.000 1.929
16 x7 ~1 1 1 16 nu.7.g1 .p16. 4.439 0.000 4.439
17 x8 ~1 1 1 17 nu.8.g1 .p17. 5.564 0.000 5.564
18 x9 ~1 1 1 18 nu.9.g1 .p18. 5.426 0.000 5.426
19 x1 ~~ x1 1 1 19 theta.1_1.g1 .p19. 0.577 -0.094 0.483
20 x2 ~~ x2 1 1 20 theta.2_2.g1 .p20. 1.252 0.029 1.280
21 x3 ~~ x3 1 1 21 theta.3_3.g1 .p21. 0.853 0.048 0.901
22 x4 ~~ x4 1 1 22 theta.4_4.g1 .p22. 0.447 0.005 0.453
23 x5 ~~ x5 1 1 23 theta.5_5.g1 .p23. 0.486 -0.048 0.439
24 x6 ~~ x6 1 1 24 theta.6_6.g1 .p24. 0.258 0.026 0.284
25 x7 ~~ x7 1 1 25 theta.7_7.g1 .p25. 0.860 -0.007 0.853
26 x8 ~~ x8 1 1 26 theta.8_8.g1 .p26. 0.512 0.004 0.516
27 x9 ~~ x9 1 1 27 theta.9_9.g1 .p27. 0.635 0.002 0.637
28 visual ~1 1 1 0 alpha.1.g1 .p28. 0.000 NA NA
29 textual ~1 1 1 0 alpha.2.g1 .p29. 0.000 NA NA
30 speed ~1 1 1 0 alpha.3.g1 .p30. 0.000 NA NA
31 visual ~~ visual 1 1 0 psi.1_1.g1 .p31. 1.000 NA NA
32 textual ~~ textual 1 1 0 psi.2_2.g1 .p32. 1.000 NA NA
33 speed ~~ speed 1 1 0 psi.3_3.g1 .p33. 1.000 NA NA
34 visual ~~ textual 1 1 28 psi.2_1.g1 .p34. 0.473 -0.009 0.464
35 visual ~~ speed 1 1 29 psi.3_1.g1 .p35. 0.347 -0.007 0.341
36 textual ~~ speed 1 1 30 psi.3_2.g1 .p36. 0.320 0.001 0.320
37 visual =~ x1 2 2 31 lambda.1_1 .p37. 0.885 -0.056 0.829
38 visual =~ x2 2 2 32 lambda.2_1 .p38. 0.536 0.046 0.583
39 visual =~ x3 2 2 33 lambda.3_1 .p39. 0.707 0.033 0.740
40 textual =~ x4 2 2 34 lambda.4_2 .p40. 0.935 0.023 0.958
41 textual =~ x5 2 2 35 lambda.5_2 .p41. 1.045 -0.072 0.973
42 textual =~ x6 2 2 36 lambda.6_2 .p42. 0.874 0.065 0.939
43 speed =~ x7 2 2 37 lambda.7_3 .p43. 0.550 -0.005 0.545
44 speed =~ x8 2 2 38 lambda.8_3 .p44. 0.675 0.002 0.677
45 speed =~ x9 2 2 39 lambda.9_3 .p45. 0.602 0.002 0.604
46 x1 ~1 2 2 40 nu.1.g2 .p46. 4.930 0.000 4.930
47 x2 ~1 2 2 41 nu.2.g2 .p47. 6.198 0.000 6.198
48 x3 ~1 2 2 42 nu.3.g2 .p48. 1.992 0.000 1.992
49 x4 ~1 2 2 43 nu.4.g2 .p49. 3.318 0.000 3.318
50 x5 ~1 2 2 44 nu.5.g2 .p50. 4.714 0.000 4.714
51 x6 ~1 2 2 45 nu.6.g2 .p51. 2.467 0.000 2.467
52 x7 ~1 2 2 46 nu.7.g2 .p52. 3.923 0.000 3.923
53 x8 ~1 2 2 47 nu.8.g2 .p53. 5.488 0.000 5.488
54 x9 ~1 2 2 48 nu.9.g2 .p54. 5.338 0.000 5.338
55 x1 ~~ x1 2 2 49 theta.1_1.g2 .p55. 0.652 0.051 0.702
56 x2 ~~ x2 2 2 50 theta.2_2.g2 .p56. 0.940 -0.015 0.924
57 x3 ~~ x3 2 2 51 theta.3_3.g2 .p57. 0.606 -0.022 0.584
58 x4 ~~ x4 2 2 52 theta.4_4.g2 .p58. 0.348 -0.018 0.330
59 x5 ~~ x5 2 2 53 theta.5_5.g2 .p59. 0.371 0.050 0.421
60 x6 ~~ x6 2 2 54 theta.6_6.g2 .p60. 0.439 -0.027 0.412
61 x7 ~~ x7 2 2 55 theta.7_7.g2 .p61. 0.622 0.003 0.625
62 x8 ~~ x8 2 2 56 theta.8_8.g2 .p62. 0.410 -0.002 0.408
63 x9 ~~ x9 2 2 57 theta.9_9.g2 .p63. 0.505 -0.001 0.504
64 visual ~1 2 2 0 alpha.1.g2 .p64. 0.000 NA NA
65 textual ~1 2 2 0 alpha.2.g2 .p65. 0.000 NA NA
66 speed ~1 2 2 0 alpha.3.g2 .p66. 0.000 NA NA
67 visual ~~ visual 2 2 58 psi.1_1.g2 .p67. 0.910 -0.002 0.907
68 textual ~~ textual 2 2 59 psi.2_2.g2 .p68. 1.004 0.000 1.005
69 speed ~~ speed 2 2 60 psi.3_3.g2 .p69. 1.498 -0.001 1.497
70 visual ~~ textual 2 2 61 psi.2_1.g2 .p70. 0.520 0.003 0.523
71 visual ~~ speed 2 2 62 psi.3_1.g2 .p71. 0.638 0.003 0.641
72 textual ~~ speed 2 2 63 psi.3_2.g2 .p72. 0.429 0.001 0.430
sepc.lv sepc.all sepc.nox
1 0.072 0.062 0.062
2 -0.060 -0.048 -0.048
3 -0.052 -0.045 -0.045
4 -0.018 -0.016 -0.016
5 0.081 0.065 0.065
6 -0.042 -0.042 -0.042
7 0.013 0.012 0.012
8 -0.004 -0.004 -0.004
9 -0.004 -0.004 -0.004
10 0.000 0.000 0.000
11 0.000 0.000 0.000
12 0.000 0.000 0.000
13 0.000 0.000 0.000
14 0.000 0.000 0.000
15 0.000 0.000 0.000
16 0.000 0.000 0.000
17 0.000 0.000 0.000
18 0.000 0.000 0.000
19 -0.577 -0.424 -0.424
20 1.252 0.813 0.813
21 0.853 0.630 0.630
22 0.447 0.339 0.339
23 -0.486 -0.308 -0.308
24 0.258 0.252 0.252
25 -0.860 -0.739 -0.739
26 0.512 0.529 0.529
27 0.635 0.637 0.637
28 NA NA NA
29 NA NA NA
30 NA NA NA
31 NA NA NA
32 NA NA NA
33 NA NA NA
34 -0.009 -0.009 -0.009
35 -0.007 -0.007 -0.007
36 0.001 0.001 0.001
37 -0.053 -0.045 -0.045
38 0.044 0.040 0.040
39 0.031 0.030 0.030
40 0.023 0.021 0.021
41 -0.072 -0.060 -0.060
42 0.065 0.059 0.059
43 -0.006 -0.006 -0.006
44 0.002 0.002 0.002
45 0.003 0.003 0.003
46 0.000 0.000 0.000
47 0.000 0.000 0.000
48 0.000 0.000 0.000
49 0.000 0.000 0.000
50 0.000 0.000 0.000
51 0.000 0.000 0.000
52 0.000 0.000 0.000
53 0.000 0.000 0.000
54 0.000 0.000 0.000
55 0.652 0.478 0.478
56 -0.940 -0.782 -0.782
57 -0.606 -0.571 -0.571
58 -0.348 -0.284 -0.284
59 0.371 0.253 0.253
60 -0.439 -0.364 -0.364
61 0.622 0.578 0.578
62 -0.410 -0.375 -0.375
63 -0.505 -0.482 -0.482
64 NA NA NA
65 NA NA NA
66 NA NA NA
67 -1.000 -1.000 -1.000
68 1.000 1.000 1.000
69 -1.000 -1.000 -1.000
70 0.003 0.003 0.003
71 0.002 0.002 0.002
72 0.000 0.000 0.000
16.10.5.6.4 Equivalence Test
The petersenlab
package (Petersen, 2024b) contains the equiv_chi()
function from Counsell et al. (2020) that performs an equivalence test: https://osf.io/cqu8v.
The chi-square equivalence test is non-significant, suggesting that the model fit is not acceptable.
Code
Moreover, the equivalence test of the chi-square difference test is non-significant, suggesting that the degree of worsening of model fit is not acceptable. In other words, metric invariance failed.
Code
16.10.5.6.5 Permutation Test
Permutation procedures for testing measurement invariance are described in (Jorgensen et al., 2018).
For reproducibility, I set the seed below.
Using the same seed will yield the same answer every time.
There is nothing special about this particular seed.
You can specify the null model as the baseline model using: baseline.model = nullModelFit
.
Warning: this code takes a while to run based on \(100\) iterations.
You can reduce the number of iterations to be faster.
Code
set.seed(52242)
metricInvarianceTest <- permuteMeasEq(
nPermute = numPermutations,
modelType = "mgcfa",
con = metricInvarianceModel_fit,
uncon = configuralInvarianceModel_fit,
AFIs = myAFIs,
moreAFIs = moreAFIs,
parallelType = "multicore", #only 'snow' works on Windows, but right now, it is throwing an error
iseed = 52242)
Omnibus p value based on parametric chi-squared difference test:
Chisq diff Df diff Pr(>Chisq)
5.464 6.000 0.486
Omnibus p values based on nonparametric permutation method:
AFI.Difference p.value
chisq 6.888 0.57
chisq.scaled 2.986 0.59
rmsea -0.005 0.60
cfi -0.001 0.57
tli 0.011 0.61
srmr 0.003 0.71
rmsea.robust -0.005 0.61
cfi.robust -0.001 0.59
tli.robust 0.011 0.64
The p-values are non-significant, indicating that the model does not fit significantly worse than the configural invariance model. In other words, metric invariance held.
16.10.5.7 Internal Consistency Reliability
Internal consistency reliability of items composing the latent factors, as quantified by omega (\(\omega\)) and average variance extracted (AVE), was estimated using the semTools
package (Jorgensen et al., 2021).
16.10.5.8 Path Diagram
A path diagram of the model generated using the semPlot
package (Epskamp, 2022) is below.
Code
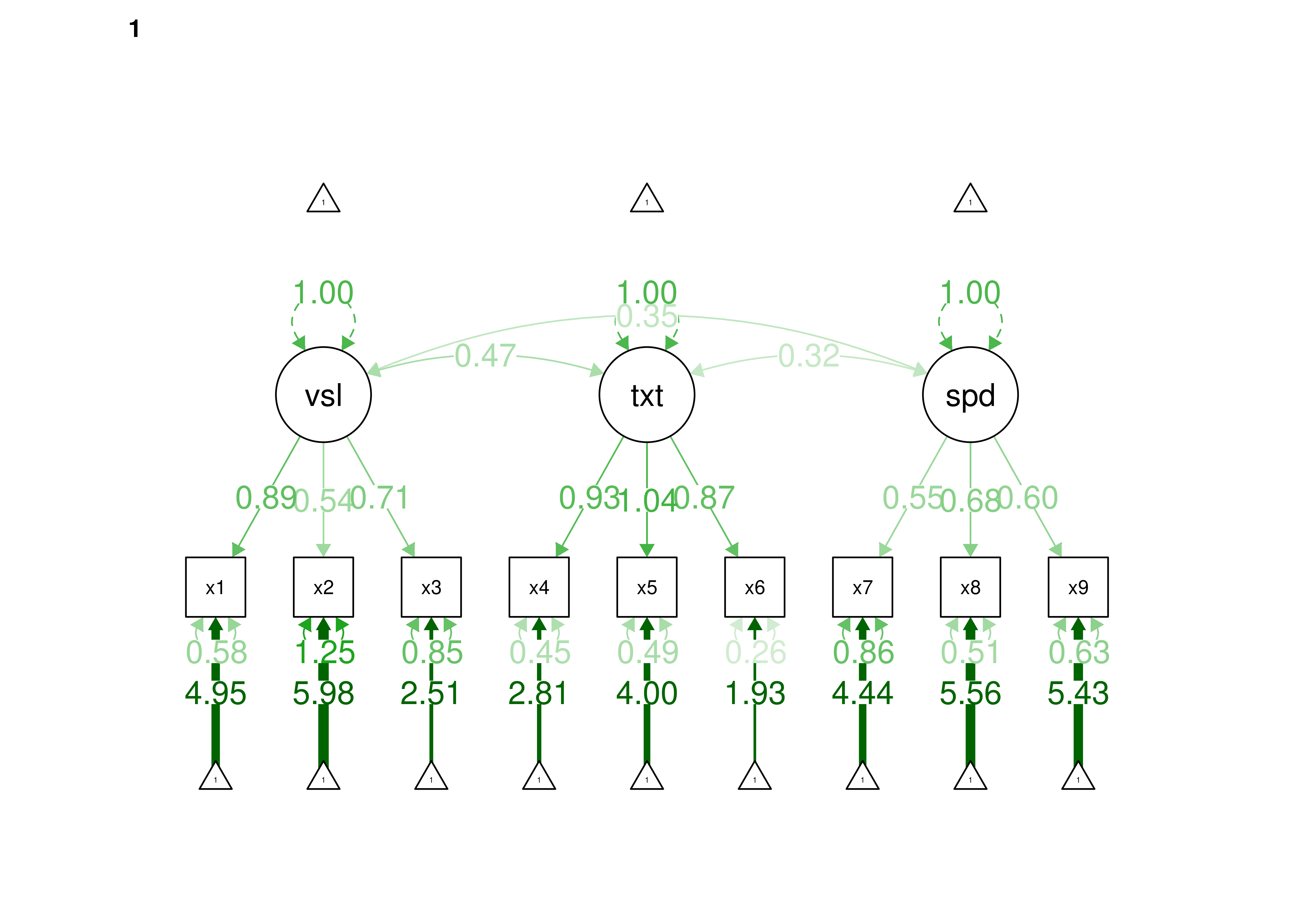
Figure 16.55: Metric Invariance Model in Confirmatory Factor Analysis.
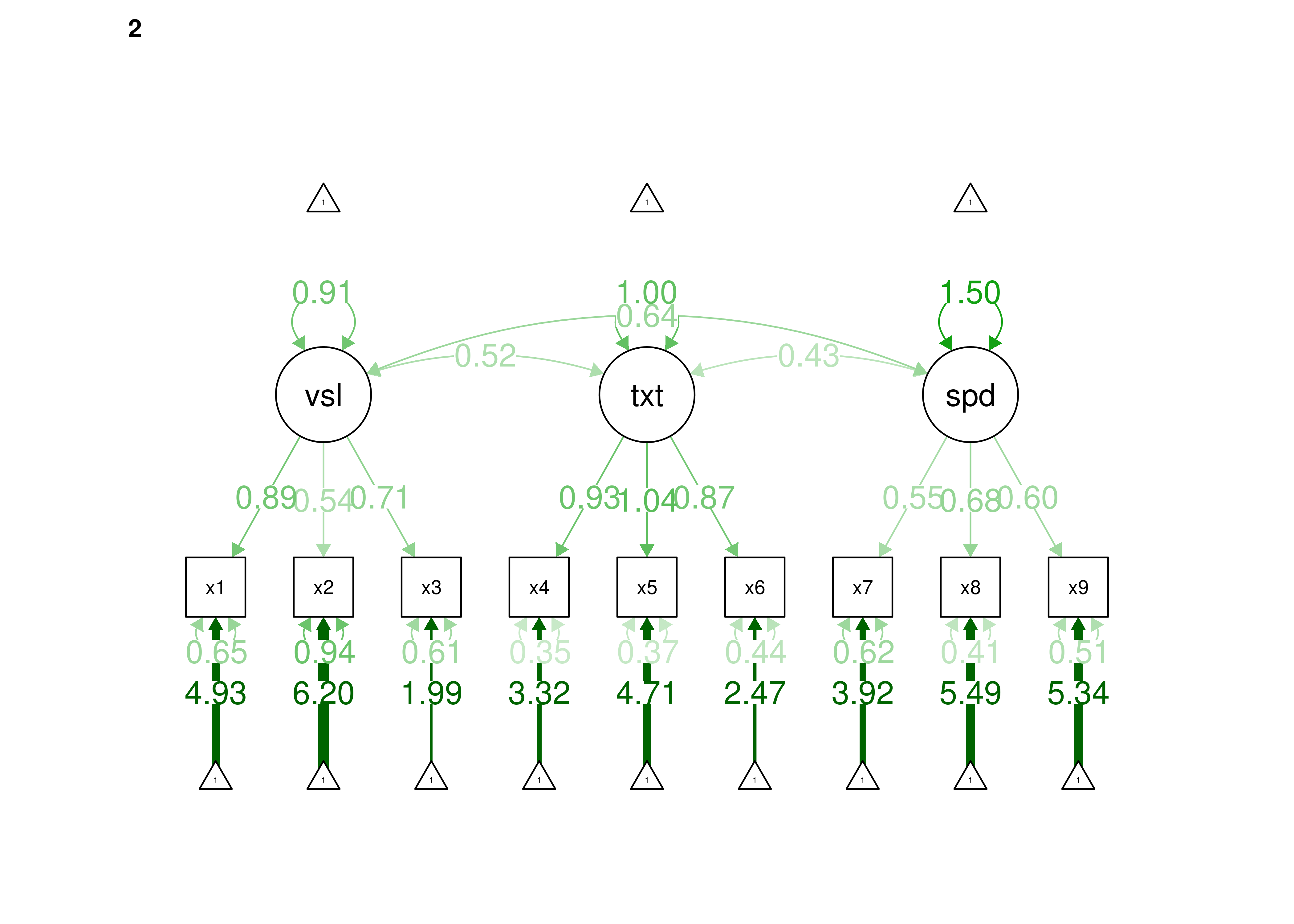
Figure 16.56: Metric Invariance Model in Confirmatory Factor Analysis.
16.10.6 Scalar (“Strong Factorial”) Invariance Model
Specify invariance of factor loadings and intercepts across groups.
16.10.6.1 Model Syntax
Code
## LOADINGS:
visual =~ c(NA, NA)*x1 + c(lambda.1_1, lambda.1_1)*x1
visual =~ c(NA, NA)*x2 + c(lambda.2_1, lambda.2_1)*x2
visual =~ c(NA, NA)*x3 + c(lambda.3_1, lambda.3_1)*x3
textual =~ c(NA, NA)*x4 + c(lambda.4_2, lambda.4_2)*x4
textual =~ c(NA, NA)*x5 + c(lambda.5_2, lambda.5_2)*x5
textual =~ c(NA, NA)*x6 + c(lambda.6_2, lambda.6_2)*x6
speed =~ c(NA, NA)*x7 + c(lambda.7_3, lambda.7_3)*x7
speed =~ c(NA, NA)*x8 + c(lambda.8_3, lambda.8_3)*x8
speed =~ c(NA, NA)*x9 + c(lambda.9_3, lambda.9_3)*x9
## INTERCEPTS:
x1 ~ c(NA, NA)*1 + c(nu.1, nu.1)*1
x2 ~ c(NA, NA)*1 + c(nu.2, nu.2)*1
x3 ~ c(NA, NA)*1 + c(nu.3, nu.3)*1
x4 ~ c(NA, NA)*1 + c(nu.4, nu.4)*1
x5 ~ c(NA, NA)*1 + c(nu.5, nu.5)*1
x6 ~ c(NA, NA)*1 + c(nu.6, nu.6)*1
x7 ~ c(NA, NA)*1 + c(nu.7, nu.7)*1
x8 ~ c(NA, NA)*1 + c(nu.8, nu.8)*1
x9 ~ c(NA, NA)*1 + c(nu.9, nu.9)*1
## UNIQUE-FACTOR VARIANCES:
x1 ~~ c(NA, NA)*x1 + c(theta.1_1.g1, theta.1_1.g2)*x1
x2 ~~ c(NA, NA)*x2 + c(theta.2_2.g1, theta.2_2.g2)*x2
x3 ~~ c(NA, NA)*x3 + c(theta.3_3.g1, theta.3_3.g2)*x3
x4 ~~ c(NA, NA)*x4 + c(theta.4_4.g1, theta.4_4.g2)*x4
x5 ~~ c(NA, NA)*x5 + c(theta.5_5.g1, theta.5_5.g2)*x5
x6 ~~ c(NA, NA)*x6 + c(theta.6_6.g1, theta.6_6.g2)*x6
x7 ~~ c(NA, NA)*x7 + c(theta.7_7.g1, theta.7_7.g2)*x7
x8 ~~ c(NA, NA)*x8 + c(theta.8_8.g1, theta.8_8.g2)*x8
x9 ~~ c(NA, NA)*x9 + c(theta.9_9.g1, theta.9_9.g2)*x9
## LATENT MEANS/INTERCEPTS:
visual ~ c(0, NA)*1 + c(alpha.1.g1, alpha.1.g2)*1
textual ~ c(0, NA)*1 + c(alpha.2.g1, alpha.2.g2)*1
speed ~ c(0, NA)*1 + c(alpha.3.g1, alpha.3.g2)*1
## COMMON-FACTOR VARIANCES:
visual ~~ c(1, NA)*visual + c(psi.1_1.g1, psi.1_1.g2)*visual
textual ~~ c(1, NA)*textual + c(psi.2_2.g1, psi.2_2.g2)*textual
speed ~~ c(1, NA)*speed + c(psi.3_3.g1, psi.3_3.g2)*speed
## COMMON-FACTOR COVARIANCES:
visual ~~ c(NA, NA)*textual + c(psi.2_1.g1, psi.2_1.g2)*textual
visual ~~ c(NA, NA)*speed + c(psi.3_1.g1, psi.3_1.g2)*speed
textual ~~ c(NA, NA)*speed + c(psi.3_2.g1, psi.3_2.g2)*speed
16.10.6.1.1 Summary of Model Features
This lavaan model syntax specifies a CFA with 9 manifest indicators of 3 common factor(s).
To identify the location and scale of each common factor, the factor means and variances were fixed to 0 and 1, respectively, unless equality constraints on measurement parameters allow them to be freed.
Pattern matrix indicating num(eric), ord(ered), and lat(ent) indicators per factor:
visual textual speed
x1 num
x2 num
x3 num
x4 num
x5 num
x6 num
x7 num
x8 num
x9 num
The following types of parameter were constrained to equality across groups:
loadings
intercepts
16.10.6.4 Model Summary
lavaan 0.6-19 ended normally after 66 iterations
Estimator ML
Optimization method NLMINB
Number of model parameters 66
Number of equality constraints 18
Number of observations per group:
Pasteur 156
Grant-White 145
Number of missing patterns per group:
Pasteur 9
Grant-White 9
Model Test User Model:
Standard Scaled
Test Statistic 156.955 159.442
Degrees of freedom 60 60
P-value (Chi-square) 0.000 0.000
Scaling correction factor 0.984
Yuan-Bentler correction (Mplus variant)
Test statistic for each group:
Pasteur 86.521 86.521
Grant-White 72.921 72.921
Model Test Baseline Model:
Test statistic 932.665 909.019
Degrees of freedom 72 72
P-value 0.000 0.000
Scaling correction factor 1.026
User Model versus Baseline Model:
Comparative Fit Index (CFI) 0.887 0.881
Tucker-Lewis Index (TLI) 0.865 0.857
Robust Comparative Fit Index (CFI) 0.890
Robust Tucker-Lewis Index (TLI) 0.868
Loglikelihood and Information Criteria:
Loglikelihood user model (H0) -3672.597 -3672.597
Scaling correction factor 0.797
for the MLR correction
Loglikelihood unrestricted model (H1) -3594.120 -3594.120
Scaling correction factor 1.034
for the MLR correction
Akaike (AIC) 7441.195 7441.195
Bayesian (BIC) 7619.136 7619.136
Sample-size adjusted Bayesian (SABIC) 7466.908 7466.908
Root Mean Square Error of Approximation:
RMSEA 0.104 0.105
90 Percent confidence interval - lower 0.084 0.085
90 Percent confidence interval - upper 0.124 0.125
P-value H_0: RMSEA <= 0.050 0.000 0.000
P-value H_0: RMSEA >= 0.080 0.975 0.980
Robust RMSEA 0.104
90 Percent confidence interval - lower 0.083
90 Percent confidence interval - upper 0.124
P-value H_0: Robust RMSEA <= 0.050 0.000
P-value H_0: Robust RMSEA >= 0.080 0.972
Standardized Root Mean Square Residual:
SRMR 0.080 0.080
Parameter Estimates:
Standard errors Sandwich
Information bread Observed
Observed information based on Hessian
Group 1 [Pasteur]:
Latent Variables:
Estimate Std.Err z-value P(>|z|) Std.lv Std.all
visual =~
x1 (l.1_) 0.874 0.132 6.630 0.000 0.874 0.751
x2 (l.2_) 0.512 0.086 5.984 0.000 0.512 0.411
x3 (l.3_) 0.722 0.095 7.610 0.000 0.722 0.604
textual =~
x4 (l.4_) 0.921 0.071 12.920 0.000 0.921 0.807
x5 (l.5_) 1.059 0.077 13.763 0.000 1.059 0.837
x6 (l.6_) 0.875 0.066 13.259 0.000 0.875 0.865
speed =~
x7 (l.7_) 0.566 0.081 6.946 0.000 0.566 0.512
x8 (l.8_) 0.657 0.082 8.028 0.000 0.657 0.669
x9 (l.9_) 0.602 0.085 7.049 0.000 0.602 0.604
Covariances:
Estimate Std.Err z-value P(>|z|) Std.lv Std.all
visual ~~
textul (p.2_) 0.473 0.108 4.397 0.000 0.473 0.473
speed (p.3_1) 0.355 0.125 2.842 0.004 0.355 0.355
textual ~~
speed (p.3_2) 0.322 0.095 3.377 0.001 0.322 0.322
Intercepts:
Estimate Std.Err z-value P(>|z|) Std.lv Std.all
.x1 (nu.1) 5.016 0.094 53.146 0.000 5.016 4.309
.x2 (nu.2) 6.156 0.088 69.594 0.000 6.156 4.944
.x3 (nu.3) 2.292 0.098 23.329 0.000 2.292 1.916
.x4 (nu.4) 2.780 0.086 32.469 0.000 2.780 2.435
.x5 (nu.5) 4.035 0.104 38.981 0.000 4.035 3.189
.x6 (nu.6) 1.928 0.074 25.882 0.000 1.928 1.907
.x7 (nu.7) 4.244 0.078 54.164 0.000 4.244 3.840
.x8 (nu.8) 5.627 0.076 73.915 0.000 5.627 5.726
.x9 (nu.9) 5.474 0.073 74.934 0.000 5.474 5.492
visual (a.1.) 0.000 0.000 0.000
textual (a.2.) 0.000 0.000 0.000
speed (a.3.) 0.000 0.000 0.000
Variances:
Estimate Std.Err z-value P(>|z|) Std.lv Std.all
.x1 (t.1_) 0.591 0.179 3.295 0.001 0.591 0.436
.x2 (t.2_) 1.288 0.160 8.075 0.000 1.288 0.831
.x3 (t.3_) 0.910 0.151 6.046 0.000 0.910 0.636
.x4 (t.4_) 0.454 0.073 6.245 0.000 0.454 0.349
.x5 (t.5_) 0.480 0.076 6.295 0.000 0.480 0.300
.x6 (t.6_) 0.257 0.058 4.401 0.000 0.257 0.252
.x7 (t.7_) 0.901 0.129 7.000 0.000 0.901 0.738
.x8 (t.8_) 0.534 0.091 5.838 0.000 0.534 0.553
.x9 (t.9_) 0.631 0.115 5.469 0.000 0.631 0.635
visual (p.1_) 1.000 1.000 1.000
textual (p.2_) 1.000 1.000 1.000
speed (p.3_) 1.000 1.000 1.000
R-Square:
Estimate
x1 0.564
x2 0.169
x3 0.364
x4 0.651
x5 0.700
x6 0.748
x7 0.262
x8 0.447
x9 0.365
Group 2 [Grant-White]:
Latent Variables:
Estimate Std.Err z-value P(>|z|) Std.lv Std.all
visual =~
x1 (l.1_) 0.874 0.132 6.630 0.000 0.827 0.711
x2 (l.2_) 0.512 0.086 5.984 0.000 0.485 0.441
x3 (l.3_) 0.722 0.095 7.610 0.000 0.684 0.649
textual =~
x4 (l.4_) 0.921 0.071 12.920 0.000 0.921 0.838
x5 (l.5_) 1.059 0.077 13.763 0.000 1.059 0.869
x6 (l.6_) 0.875 0.066 13.259 0.000 0.875 0.797
speed =~
x7 (l.7_) 0.566 0.081 6.946 0.000 0.692 0.651
x8 (l.8_) 0.657 0.082 8.028 0.000 0.803 0.773
x9 (l.9_) 0.602 0.085 7.049 0.000 0.736 0.722
Covariances:
Estimate Std.Err z-value P(>|z|) Std.lv Std.all
visual ~~
textul (p.2_) 0.519 0.136 3.829 0.000 0.549 0.549
speed (p.3_1) 0.649 0.215 3.014 0.003 0.561 0.561
textual ~~
speed (p.3_2) 0.442 0.189 2.335 0.020 0.361 0.361
Intercepts:
Estimate Std.Err z-value P(>|z|) Std.lv Std.all
.x1 (nu.1) 5.016 0.094 53.146 0.000 5.016 4.312
.x2 (nu.2) 6.156 0.088 69.594 0.000 6.156 5.602
.x3 (nu.3) 2.292 0.098 23.329 0.000 2.292 2.177
.x4 (nu.4) 2.780 0.086 32.469 0.000 2.780 2.529
.x5 (nu.5) 4.035 0.104 38.981 0.000 4.035 3.312
.x6 (nu.6) 1.928 0.074 25.882 0.000 1.928 1.756
.x7 (nu.7) 4.244 0.078 54.164 0.000 4.244 3.990
.x8 (nu.8) 5.627 0.076 73.915 0.000 5.627 5.412
.x9 (nu.9) 5.474 0.073 74.934 0.000 5.474 5.370
visual (a.1.) -0.193 0.175 -1.101 0.271 -0.204 -0.204
textual (a.2.) 0.617 0.135 4.587 0.000 0.617 0.617
speed (a.3.) -0.294 0.161 -1.828 0.068 -0.240 -0.240
Variances:
Estimate Std.Err z-value P(>|z|) Std.lv Std.all
.x1 (t.1_) 0.668 0.176 3.793 0.000 0.668 0.494
.x2 (t.2_) 0.973 0.152 6.384 0.000 0.973 0.805
.x3 (t.3_) 0.641 0.133 4.833 0.000 0.641 0.578
.x4 (t.4_) 0.359 0.066 5.464 0.000 0.359 0.297
.x5 (t.5_) 0.363 0.074 4.883 0.000 0.363 0.245
.x6 (t.6_) 0.441 0.075 5.851 0.000 0.441 0.366
.x7 (t.7_) 0.652 0.108 6.016 0.000 0.652 0.577
.x8 (t.8_) 0.436 0.152 2.872 0.004 0.436 0.403
.x9 (t.9_) 0.497 0.127 3.918 0.000 0.497 0.478
visual (p.1_) 0.896 0.235 3.816 0.000 1.000 1.000
textual (p.2_) 1.000 0.194 5.157 0.000 1.000 1.000
speed (p.3_) 1.495 0.360 4.156 0.000 1.000 1.000
R-Square:
Estimate
x1 0.506
x2 0.195
x3 0.422
x4 0.703
x5 0.755
x6 0.634
x7 0.423
x8 0.597
x9 0.522
Code
lavaan 0.6-19 ended normally after 66 iterations
Estimator ML
Optimization method NLMINB
Number of model parameters 66
Number of equality constraints 18
Number of observations per group:
Pasteur 156
Grant-White 145
Number of missing patterns per group:
Pasteur 9
Grant-White 9
Model Test User Model:
Standard Scaled
Test Statistic 156.955 159.442
Degrees of freedom 60 60
P-value (Chi-square) 0.000 0.000
Scaling correction factor 0.984
Yuan-Bentler correction (Mplus variant)
Test statistic for each group:
Pasteur 86.520 86.520
Grant-White 72.921 72.921
Model Test Baseline Model:
Test statistic 932.665 909.019
Degrees of freedom 72 72
P-value 0.000 0.000
Scaling correction factor 1.026
User Model versus Baseline Model:
Comparative Fit Index (CFI) 0.887 0.881
Tucker-Lewis Index (TLI) 0.865 0.857
Robust Comparative Fit Index (CFI) 0.890
Robust Tucker-Lewis Index (TLI) 0.868
Loglikelihood and Information Criteria:
Loglikelihood user model (H0) -3672.597 -3672.597
Scaling correction factor 0.797
for the MLR correction
Loglikelihood unrestricted model (H1) -3594.120 -3594.120
Scaling correction factor 1.034
for the MLR correction
Akaike (AIC) 7441.195 7441.195
Bayesian (BIC) 7619.136 7619.136
Sample-size adjusted Bayesian (SABIC) 7466.908 7466.908
Root Mean Square Error of Approximation:
RMSEA 0.104 0.105
90 Percent confidence interval - lower 0.084 0.085
90 Percent confidence interval - upper 0.124 0.125
P-value H_0: RMSEA <= 0.050 0.000 0.000
P-value H_0: RMSEA >= 0.080 0.975 0.980
Robust RMSEA 0.104
90 Percent confidence interval - lower 0.083
90 Percent confidence interval - upper 0.124
P-value H_0: Robust RMSEA <= 0.050 0.000
P-value H_0: Robust RMSEA >= 0.080 0.972
Standardized Root Mean Square Residual:
SRMR 0.080 0.080
Parameter Estimates:
Standard errors Sandwich
Information bread Observed
Observed information based on Hessian
Group 1 [Pasteur]:
Latent Variables:
Estimate Std.Err z-value P(>|z|) Std.lv Std.all
visual =~
x1 (lmb1) 0.874 0.132 6.630 0.000 0.874 0.751
x2 (lmb2) 0.512 0.086 5.984 0.000 0.512 0.411
x3 (lmb3) 0.722 0.095 7.610 0.000 0.722 0.604
textual =~
x4 (lmb4) 0.921 0.071 12.920 0.000 0.921 0.807
x5 (lmb5) 1.059 0.077 13.763 0.000 1.059 0.837
x6 (lmb6) 0.875 0.066 13.259 0.000 0.875 0.865
speed =~
x7 (lmb7) 0.566 0.081 6.946 0.000 0.566 0.512
x8 (lmb8) 0.657 0.082 8.028 0.000 0.657 0.669
x9 (lmb9) 0.602 0.085 7.049 0.000 0.602 0.604
Covariances:
Estimate Std.Err z-value P(>|z|) Std.lv Std.all
visual ~~
textual 0.473 0.108 4.397 0.000 0.473 0.473
speed 0.355 0.125 2.842 0.004 0.355 0.355
textual ~~
speed 0.322 0.095 3.377 0.001 0.322 0.322
Intercepts:
Estimate Std.Err z-value P(>|z|) Std.lv Std.all
visual 0.000 0.000 0.000
textual 0.000 0.000 0.000
speed 0.000 0.000 0.000
.x1 (int1) 5.016 0.094 53.146 0.000 5.016 4.309
.x2 (int2) 6.156 0.088 69.594 0.000 6.156 4.944
.x3 (int3) 2.292 0.098 23.329 0.000 2.292 1.916
.x4 (int4) 2.780 0.086 32.469 0.000 2.780 2.435
.x5 (int5) 4.035 0.104 38.981 0.000 4.035 3.189
.x6 (int6) 1.928 0.074 25.882 0.000 1.928 1.907
.x7 (int7) 4.244 0.078 54.164 0.000 4.244 3.840
.x8 (int8) 5.627 0.076 73.915 0.000 5.627 5.726
.x9 (int9) 5.474 0.073 74.934 0.000 5.474 5.492
Variances:
Estimate Std.Err z-value P(>|z|) Std.lv Std.all
visual 1.000 1.000 1.000
textual 1.000 1.000 1.000
speed 1.000 1.000 1.000
.x1 0.591 0.179 3.295 0.001 0.591 0.436
.x2 1.288 0.160 8.075 0.000 1.288 0.831
.x3 0.910 0.151 6.046 0.000 0.910 0.636
.x4 0.454 0.073 6.245 0.000 0.454 0.349
.x5 0.480 0.076 6.295 0.000 0.480 0.300
.x6 0.257 0.058 4.401 0.000 0.257 0.252
.x7 0.901 0.129 7.000 0.000 0.901 0.738
.x8 0.534 0.091 5.838 0.000 0.534 0.553
.x9 0.631 0.115 5.469 0.000 0.631 0.635
R-Square:
Estimate
x1 0.564
x2 0.169
x3 0.364
x4 0.651
x5 0.700
x6 0.748
x7 0.262
x8 0.447
x9 0.365
Group 2 [Grant-White]:
Latent Variables:
Estimate Std.Err z-value P(>|z|) Std.lv Std.all
visual =~
x1 (lmb1) 0.874 0.132 6.630 0.000 0.827 0.711
x2 (lmb2) 0.512 0.086 5.984 0.000 0.485 0.441
x3 (lmb3) 0.722 0.095 7.610 0.000 0.684 0.649
textual =~
x4 (lmb4) 0.921 0.071 12.920 0.000 0.921 0.838
x5 (lmb5) 1.059 0.077 13.763 0.000 1.059 0.869
x6 (lmb6) 0.875 0.066 13.259 0.000 0.875 0.797
speed =~
x7 (lmb7) 0.566 0.081 6.946 0.000 0.692 0.651
x8 (lmb8) 0.657 0.082 8.028 0.000 0.803 0.773
x9 (lmb9) 0.602 0.085 7.049 0.000 0.736 0.722
Covariances:
Estimate Std.Err z-value P(>|z|) Std.lv Std.all
visual ~~
textual 0.519 0.136 3.829 0.000 0.549 0.549
speed 0.649 0.215 3.014 0.003 0.561 0.561
textual ~~
speed 0.442 0.189 2.335 0.020 0.361 0.361
Intercepts:
Estimate Std.Err z-value P(>|z|) Std.lv Std.all
visual -0.193 0.175 -1.101 0.271 -0.204 -0.204
textual 0.617 0.135 4.587 0.000 0.617 0.617
speed -0.294 0.161 -1.828 0.068 -0.240 -0.240
.x1 (int1) 5.016 0.094 53.146 0.000 5.016 4.312
.x2 (int2) 6.156 0.088 69.594 0.000 6.156 5.602
.x3 (int3) 2.292 0.098 23.329 0.000 2.292 2.177
.x4 (int4) 2.780 0.086 32.469 0.000 2.780 2.529
.x5 (int5) 4.035 0.104 38.981 0.000 4.035 3.312
.x6 (int6) 1.928 0.074 25.882 0.000 1.928 1.756
.x7 (int7) 4.244 0.078 54.164 0.000 4.244 3.990
.x8 (int8) 5.627 0.076 73.915 0.000 5.627 5.412
.x9 (int9) 5.474 0.073 74.934 0.000 5.474 5.370
Variances:
Estimate Std.Err z-value P(>|z|) Std.lv Std.all
visual 0.896 0.235 3.815 0.000 1.000 1.000
textual 1.000 0.194 5.157 0.000 1.000 1.000
speed 1.495 0.360 4.156 0.000 1.000 1.000
.x1 0.668 0.176 3.793 0.000 0.668 0.494
.x2 0.973 0.152 6.384 0.000 0.973 0.805
.x3 0.641 0.133 4.833 0.000 0.641 0.578
.x4 0.359 0.066 5.464 0.000 0.359 0.297
.x5 0.363 0.074 4.883 0.000 0.363 0.245
.x6 0.441 0.075 5.851 0.000 0.441 0.366
.x7 0.652 0.108 6.016 0.000 0.652 0.577
.x8 0.436 0.152 2.872 0.004 0.436 0.403
.x9 0.497 0.127 3.918 0.000 0.497 0.478
R-Square:
Estimate
x1 0.506
x2 0.195
x3 0.422
x4 0.703
x5 0.755
x6 0.634
x7 0.423
x8 0.597
x9 0.522
16.10.6.5 Model Fit
You can specify the null model as the baseline model using: baseline.model = nullModelFit
npar fmin
48.000 0.261
chisq df
156.955 60.000
pvalue chisq.scaled
0.000 159.442
df.scaled pvalue.scaled
60.000 0.000
chisq.scaling.factor baseline.chisq
0.984 932.665
baseline.df baseline.pvalue
72.000 0.000
baseline.chisq.scaled baseline.df.scaled
909.019 72.000
baseline.pvalue.scaled baseline.chisq.scaling.factor
0.000 1.026
cfi tli
0.887 0.865
cfi.scaled tli.scaled
0.881 0.857
cfi.robust tli.robust
0.890 0.868
nnfi rfi
0.865 0.798
nfi pnfi
0.832 0.693
ifi rni
0.889 0.887
nnfi.scaled rfi.scaled
0.857 0.790
nfi.scaled pnfi.scaled
0.825 0.687
ifi.scaled rni.scaled
0.883 0.881
nnfi.robust rni.robust
0.868 0.890
logl unrestricted.logl
-3672.597 -3594.120
aic bic
7441.195 7619.136
ntotal bic2
301.000 7466.908
scaling.factor.h1 scaling.factor.h0
1.034 0.797
rmsea rmsea.ci.lower
0.104 0.084
rmsea.ci.upper rmsea.ci.level
0.124 0.900
rmsea.pvalue rmsea.close.h0
0.000 0.050
rmsea.notclose.pvalue rmsea.notclose.h0
0.975 0.080
rmsea.scaled rmsea.ci.lower.scaled
0.105 0.085
rmsea.ci.upper.scaled rmsea.pvalue.scaled
0.125 0.000
rmsea.notclose.pvalue.scaled rmsea.robust
0.980 0.104
rmsea.ci.lower.robust rmsea.ci.upper.robust
0.083 0.124
rmsea.pvalue.robust rmsea.notclose.pvalue.robust
0.000 0.972
rmr rmr_nomean
0.097 0.095
srmr srmr_bentler
0.080 0.080
srmr_bentler_nomean crmr
0.076 0.084
crmr_nomean srmr_mplus
0.081 0.084
srmr_mplus_nomean cn_05
0.075 152.660
cn_01 gfi
170.490 0.993
agfi pgfi
0.987 0.552
mfi ecvi
0.851 0.840
Code
scalarInvarianceModelFitIndices <- fitMeasures(
scalarInvarianceModel_fit)[c(
"cfi.robust", "rmsea.robust", "srmr")]
scalarInvarianceModel_chisquare <- fitMeasures(
scalarInvarianceModel_fit)[c("chisq.scaled")]
scalarInvarianceModel_chisquareScaling <- fitMeasures(
scalarInvarianceModel_fit)[c("chisq.scaling.factor")]
scalarInvarianceModel_df <- fitMeasures(
scalarInvarianceModel_fit)[c("df.scaled")]
scalarInvarianceModel_N <- lavInspect(
scalarInvarianceModel_fit, what = "ntotal")
16.10.6.6 Compare Model Fit
16.10.6.6.1 Nested Model (\(\chi^2\)) Difference Test
The metric invariance model and the scalar (“strong factorial”) invariance model are considered “nested” models. The scalar invariance model is nested within the metric invariance model because the metric invariance model includes all of the terms of the metric invariance model along with additional terms. Model fit of nested models can be compared with a chi-square difference test, also known as a likelihood ratio test or deviance test. A significant chi-square difference test would indicate that the simplified model with additional constraints (the scalar invariance model) is significantly worse fitting than the more complex model that has fewer constraints (the metric invariance model).
The scalar invariance model fit significantly worse than the configural invariance model, so scalar invariance did not hold. This provides evidence of measurement non-invariance of indicator intercepts across groups. Measurement non-invariance of indicator intercepts poses challenges to being able to meaningfully compare levels on the latent factor across groups (Little et al., 2007).
The petersenlab
package (Petersen, 2024b) contains the satorraBentlerScaledChiSquareDifferenceTestStatistic()
function that performs a Satorra-Bentler scaled chi-square difference test:
Code
scalarInvarianceModel_chisquareDiff <-
satorraBentlerScaledChiSquareDifferenceTestStatistic(
T0 = scalarInvarianceModel_chisquare,
c0 = scalarInvarianceModel_chisquareScaling,
d0 = scalarInvarianceModel_df,
T1 = metricInvarianceModel_chisquare,
c1 = metricInvarianceModel_chisquareScaling,
d1 = metricInvarianceModel_df)
scalarInvarianceModel_chisquareDiff
chisq.scaled
41.928
16.10.6.6.3 Score-Based Test
Score-based tests of measurement invariance are implemented using the strucchange
package and are described by T. Wang et al. (2014).
lambda.1_1 lambda.2_1 lambda.3_1 lambda.4_2 lambda.5_2 lambda.6_2
0.874 0.512 0.722 0.921 1.059 0.875
lambda.7_3 lambda.8_3 lambda.9_3 nu.1 nu.2 nu.3
0.566 0.657 0.602 5.016 6.156 2.292
nu.4 nu.5 nu.6 nu.7 nu.8 nu.9
2.780 4.035 1.928 4.244 5.627 5.474
theta.1_1.g1 theta.2_2.g1 theta.3_3.g1 theta.4_4.g1 theta.5_5.g1 theta.6_6.g1
0.591 1.288 0.910 0.454 0.480 0.257
theta.7_7.g1 theta.8_8.g1 theta.9_9.g1 psi.2_1.g1 psi.3_1.g1 psi.3_2.g1
0.901 0.534 0.631 0.473 0.355 0.322
lambda.1_1 lambda.2_1 lambda.3_1 lambda.4_2 lambda.5_2 lambda.6_2
0.874 0.512 0.722 0.921 1.059 0.875
lambda.7_3 lambda.8_3 lambda.9_3 nu.1 nu.2 nu.3
0.566 0.657 0.602 5.016 6.156 2.292
nu.4 nu.5 nu.6 nu.7 nu.8 nu.9
2.780 4.035 1.928 4.244 5.627 5.474
theta.1_1.g2 theta.2_2.g2 theta.3_3.g2 theta.4_4.g2 theta.5_5.g2 theta.6_6.g2
0.668 0.973 0.641 0.359 0.363 0.441
theta.7_7.g2 theta.8_8.g2 theta.9_9.g2 alpha.1.g2 alpha.2.g2 alpha.3.g2
0.652 0.436 0.497 -0.193 0.617 -0.294
psi.1_1.g2 psi.2_2.g2 psi.3_3.g2 psi.2_1.g2 psi.3_1.g2 psi.3_2.g2
0.896 1.000 1.495 0.519 0.649 0.442
nu.1 nu.2 nu.3 nu.4 nu.5 nu.6 nu.7 nu.8
5.015537 6.155701 2.292365 2.779781 4.034564 1.928163 4.243593 5.626570
nu.9
5.474024
Code
M-fluctuation test
data: scalarInvarianceModel_fit
f(efp) = 44.639, p-value = 1.076e-06
A score-based test and expected parameter change (EPC) estimates (Oberski, 2014; Oberski et al., 2015) are provided by the lavaan
package (Rosseel et al., 2022).
$test
total score test:
test X2 df p.value
1 score 46.446 18 0
$uni
univariate score tests:
lhs op rhs X2 df p.value
1 .p1. == .p37. 2.216 1 0.137
2 .p2. == .p38. 0.115 1 0.734
3 .p3. == .p39. 1.483 1 0.223
4 .p4. == .p40. 0.039 1 0.843
5 .p5. == .p41. 2.724 1 0.099
6 .p6. == .p42. 2.205 1 0.138
7 .p7. == .p43. 0.088 1 0.767
8 .p8. == .p44. 0.035 1 0.851
9 .p9. == .p45. 0.010 1 0.922
10 .p10. == .p46. 6.336 1 0.012
11 .p11. == .p47. 7.421 1 0.006
12 .p12. == .p48. 20.450 1 0.000
13 .p13. == .p49. 1.216 1 0.270
14 .p14. == .p50. 1.146 1 0.284
15 .p15. == .p51. 0.001 1 0.976
16 .p16. == .p52. 15.301 1 0.000
17 .p17. == .p53. 4.102 1 0.043
18 .p18. == .p54. 1.602 1 0.206
$epc
expected parameter changes (epc) and expected parameter values (epv):
lhs op rhs block group free label plabel est epc epv
1 visual =~ x1 1 1 1 lambda.1_1 .p1. 0.874 0.100 0.974
2 visual =~ x2 1 1 2 lambda.2_1 .p2. 0.512 -0.038 0.474
3 visual =~ x3 1 1 3 lambda.3_1 .p3. 0.722 -0.098 0.624
4 textual =~ x4 1 1 4 lambda.4_2 .p4. 0.921 -0.006 0.915
5 textual =~ x5 1 1 5 lambda.5_2 .p5. 1.059 0.067 1.126
6 textual =~ x6 1 1 6 lambda.6_2 .p6. 0.875 -0.042 0.833
7 speed =~ x7 1 1 7 lambda.7_3 .p7. 0.566 -0.024 0.542
8 speed =~ x8 1 1 8 lambda.8_3 .p8. 0.657 0.012 0.669
9 speed =~ x9 1 1 9 lambda.9_3 .p9. 0.602 0.004 0.606
10 x1 ~1 1 1 10 nu.1 .p10. 5.016 -0.068 4.948
11 x2 ~1 1 1 11 nu.2 .p11. 6.156 -0.172 5.984
12 x3 ~1 1 1 12 nu.3 .p12. 2.292 0.217 2.510
13 x4 ~1 1 1 13 nu.4 .p13. 2.780 0.034 2.814
14 x5 ~1 1 1 14 nu.5 .p14. 4.035 -0.032 4.002
15 x6 ~1 1 1 15 nu.6 .p15. 1.928 0.001 1.929
16 x7 ~1 1 1 16 nu.7 .p16. 4.244 0.195 4.439
17 x8 ~1 1 1 17 nu.8 .p17. 5.627 -0.063 5.563
18 x9 ~1 1 1 18 nu.9 .p18. 5.474 -0.048 5.426
19 x1 ~~ x1 1 1 19 theta.1_1.g1 .p19. 0.591 -0.132 0.459
20 x2 ~~ x2 1 1 20 theta.2_2.g1 .p20. 1.288 0.022 1.310
21 x3 ~~ x3 1 1 21 theta.3_3.g1 .p21. 0.910 0.086 0.997
22 x4 ~~ x4 1 1 22 theta.4_4.g1 .p22. 0.454 0.000 0.454
23 x5 ~~ x5 1 1 23 theta.5_5.g1 .p23. 0.480 -0.042 0.437
24 x6 ~~ x6 1 1 24 theta.6_6.g1 .p24. 0.257 0.026 0.283
25 x7 ~~ x7 1 1 25 theta.7_7.g1 .p25. 0.901 0.013 0.914
26 x8 ~~ x8 1 1 26 theta.8_8.g1 .p26. 0.534 -0.010 0.524
27 x9 ~~ x9 1 1 27 theta.9_9.g1 .p27. 0.631 -0.002 0.629
28 visual ~1 1 1 0 alpha.1.g1 .p28. 0.000 NA NA
29 textual ~1 1 1 0 alpha.2.g1 .p29. 0.000 NA NA
30 speed ~1 1 1 0 alpha.3.g1 .p30. 0.000 NA NA
31 visual ~~ visual 1 1 0 psi.1_1.g1 .p31. 1.000 NA NA
32 textual ~~ textual 1 1 0 psi.2_2.g1 .p32. 1.000 NA NA
33 speed ~~ speed 1 1 0 psi.3_3.g1 .p33. 1.000 NA NA
34 visual ~~ textual 1 1 28 psi.2_1.g1 .p34. 0.473 -0.013 0.460
35 visual ~~ speed 1 1 29 psi.3_1.g1 .p35. 0.355 -0.010 0.345
36 textual ~~ speed 1 1 30 psi.3_2.g1 .p36. 0.322 0.000 0.322
37 visual =~ x1 2 2 31 lambda.1_1 .p37. 0.874 -0.035 0.839
38 visual =~ x2 2 2 32 lambda.2_1 .p38. 0.512 0.068 0.580
39 visual =~ x3 2 2 33 lambda.3_1 .p39. 0.722 -0.002 0.721
40 textual =~ x4 2 2 34 lambda.4_2 .p40. 0.921 0.038 0.959
41 textual =~ x5 2 2 35 lambda.5_2 .p41. 1.059 -0.086 0.973
42 textual =~ x6 2 2 36 lambda.6_2 .p42. 0.875 0.067 0.942
43 speed =~ x7 2 2 37 lambda.7_3 .p43. 0.566 -0.036 0.530
44 speed =~ x8 2 2 38 lambda.8_3 .p44. 0.657 0.017 0.674
45 speed =~ x9 2 2 39 lambda.9_3 .p45. 0.602 0.009 0.611
46 x1 ~1 2 2 40 nu.1 .p46. 5.016 0.066 5.081
47 x2 ~1 2 2 41 nu.2 .p47. 6.156 0.148 6.304
48 x3 ~1 2 2 42 nu.3 .p48. 2.292 -0.169 2.123
49 x4 ~1 2 2 43 nu.4 .p49. 2.780 -0.053 2.727
50 x5 ~1 2 2 44 nu.5 .p50. 4.035 0.080 4.115
51 x6 ~1 2 2 45 nu.6 .p51. 1.928 -0.042 1.886
52 x7 ~1 2 2 46 nu.7 .p52. 4.244 -0.158 4.086
53 x8 ~1 2 2 47 nu.8 .p53. 5.627 0.068 5.695
54 x9 ~1 2 2 48 nu.9 .p54. 5.474 0.051 5.525
55 x1 ~~ x1 2 2 49 theta.1_1.g2 .p55. 0.668 0.029 0.697
56 x2 ~~ x2 2 2 50 theta.2_2.g2 .p56. 0.973 -0.021 0.952
57 x3 ~~ x3 2 2 51 theta.3_3.g2 .p57. 0.641 -0.002 0.639
58 x4 ~~ x4 2 2 52 theta.4_4.g2 .p58. 0.359 -0.026 0.333
59 x5 ~~ x5 2 2 53 theta.5_5.g2 .p59. 0.363 0.064 0.427
60 x6 ~~ x6 2 2 54 theta.6_6.g2 .p60. 0.441 -0.029 0.411
61 x7 ~~ x7 2 2 55 theta.7_7.g2 .p61. 0.652 0.021 0.674
62 x8 ~~ x8 2 2 56 theta.8_8.g2 .p62. 0.436 -0.015 0.420
63 x9 ~~ x9 2 2 57 theta.9_9.g2 .p63. 0.497 -0.004 0.493
64 visual ~1 2 2 58 alpha.1.g2 .p64. -0.193 0.011 -0.182
65 textual ~1 2 2 59 alpha.2.g2 .p65. 0.617 -0.001 0.617
66 speed ~1 2 2 60 alpha.3.g2 .p66. -0.294 -0.013 -0.307
67 visual ~~ visual 2 2 61 psi.1_1.g2 .p67. 0.896 0.001 0.897
68 textual ~~ textual 2 2 62 psi.2_2.g2 .p68. 1.000 0.001 1.000
69 speed ~~ speed 2 2 63 psi.3_3.g2 .p69. 1.495 -0.003 1.492
70 visual ~~ textual 2 2 64 psi.2_1.g2 .p70. 0.519 0.004 0.524
71 visual ~~ speed 2 2 65 psi.3_1.g2 .p71. 0.649 0.002 0.650
72 textual ~~ speed 2 2 66 psi.3_2.g2 .p72. 0.442 0.000 0.442
sepc.lv sepc.all sepc.nox
1 0.100 0.086 0.086
2 -0.038 -0.031 -0.031
3 -0.098 -0.082 -0.082
4 -0.006 -0.005 -0.005
5 0.067 0.053 0.053
6 -0.042 -0.042 -0.042
7 -0.024 -0.021 -0.021
8 0.012 0.012 0.012
9 0.004 0.004 0.004
10 -0.068 -0.058 -0.058
11 -0.172 -0.138 -0.138
12 0.217 0.182 0.182
13 0.034 0.030 0.030
14 -0.032 -0.026 -0.026
15 0.001 0.001 0.001
16 0.195 0.177 0.177
17 -0.063 -0.064 -0.064
18 -0.048 -0.048 -0.048
19 -0.591 -0.436 -0.436
20 1.288 0.831 0.831
21 0.910 0.636 0.636
22 0.454 0.349 0.349
23 -0.480 -0.300 -0.300
24 0.257 0.252 0.252
25 0.901 0.738 0.738
26 -0.534 -0.553 -0.553
27 -0.631 -0.635 -0.635
28 NA NA NA
29 NA NA NA
30 NA NA NA
31 NA NA NA
32 NA NA NA
33 NA NA NA
34 -0.013 -0.013 -0.013
35 -0.010 -0.010 -0.010
36 0.000 0.000 0.000
37 -0.033 -0.028 -0.028
38 0.064 0.059 0.059
39 -0.002 -0.001 -0.001
40 0.038 0.034 0.034
41 -0.086 -0.070 -0.070
42 0.067 0.061 0.061
43 -0.044 -0.041 -0.041
44 0.021 0.020 0.020
45 0.011 0.011 0.011
46 0.066 0.057 0.057
47 0.148 0.135 0.135
48 -0.169 -0.161 -0.161
49 -0.053 -0.048 -0.048
50 0.080 0.066 0.066
51 -0.042 -0.038 -0.038
52 -0.158 -0.148 -0.148
53 0.068 0.066 0.066
54 0.051 0.050 0.050
55 0.668 0.494 0.494
56 -0.973 -0.805 -0.805
57 -0.641 -0.578 -0.578
58 -0.359 -0.297 -0.297
59 0.363 0.245 0.245
60 -0.441 -0.366 -0.366
61 0.652 0.577 0.577
62 -0.436 -0.403 -0.403
63 -0.497 -0.478 -0.478
64 0.012 0.012 0.012
65 -0.001 -0.001 -0.001
66 -0.011 -0.011 -0.011
67 1.000 1.000 1.000
68 1.000 1.000 1.000
69 -1.000 -1.000 -1.000
70 0.004 0.004 0.004
71 0.001 0.001 0.001
72 0.000 0.000 0.000
16.10.6.6.4 Equivalence Test
The petersenlab
package (Petersen, 2024b) contains the equiv_chi()
function from Counsell et al. (2020) that performs an equivalence test: https://osf.io/cqu8v.
The chi-square equivalence test is non-significant, suggesting that the model fit is not acceptable.
Code
Moreover, the equivalence test of the chi-square difference test is non-significant, suggesting that the degree of worsening of model fit is not acceptable. In other words, scalar invariance failed.
Code
16.10.6.6.5 Permutation Test
Permutation procedures for testing measurement invariance are described in (Jorgensen et al., 2018).
For reproducibility, I set the seed below.
Using the same seed will yield the same answer every time.
There is nothing special about this particular seed.
You can specify the null model as the baseline model using: baseline.model = nullModelFit
Warning: this code takes a while to run based on 100 iterations. You can reduce the number of iterations to be faster.
Code
set.seed(52242)
scalarInvarianceTest <- permuteMeasEq(
nPermute = numPermutations,
modelType = "mgcfa",
con = scalarInvarianceModel_fit,
uncon = metricInvarianceModel_fit,
AFIs = myAFIs,
moreAFIs = moreAFIs,
parallelType = "multicore", #only 'snow' works on Windows, but right now, it is throwing an error
iseed = 52242)
Omnibus p value based on parametric chi-squared difference test:
Chisq diff Df diff Pr(>Chisq)
41.928 6.000 0.000
Omnibus p values based on nonparametric permutation method:
AFI.Difference p.value
chisq 40.641 0
chisq.scaled 41.486 0
rmsea 0.016 0
cfi -0.040 0
tli -0.039 0
srmr 0.011 0
rmsea.robust 0.015 0
cfi.robust -0.038 0
tli.robust -0.036 0
The p-values are significant, indicating that the model fit significantly worse than the metric invariance model. In other words, scalar invariance failed.
16.10.6.7 Internal Consistency Reliability
Internal consistency reliability of items composing the latent factors, as quantified by omega (\(\omega\)) and average variance extracted (AVE), was estimated using the semTools
package (Jorgensen et al., 2021).
16.10.6.8 Path Diagram
A path diagram of the model generated using the semPlot
package (Epskamp, 2022) is below.
Code
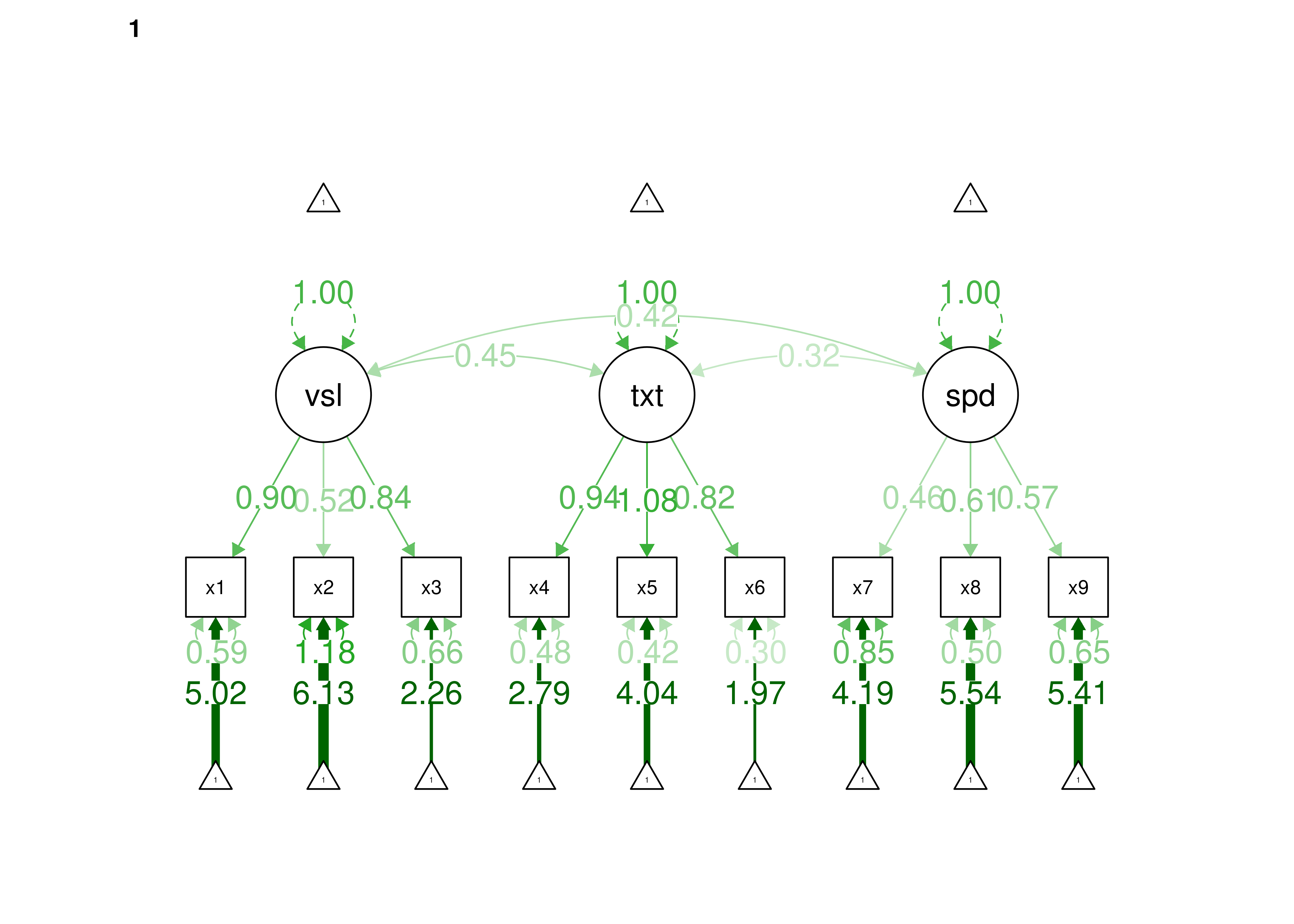
Figure 16.57: Scalar Invariance Model in Confirmatory Factor Analysis.
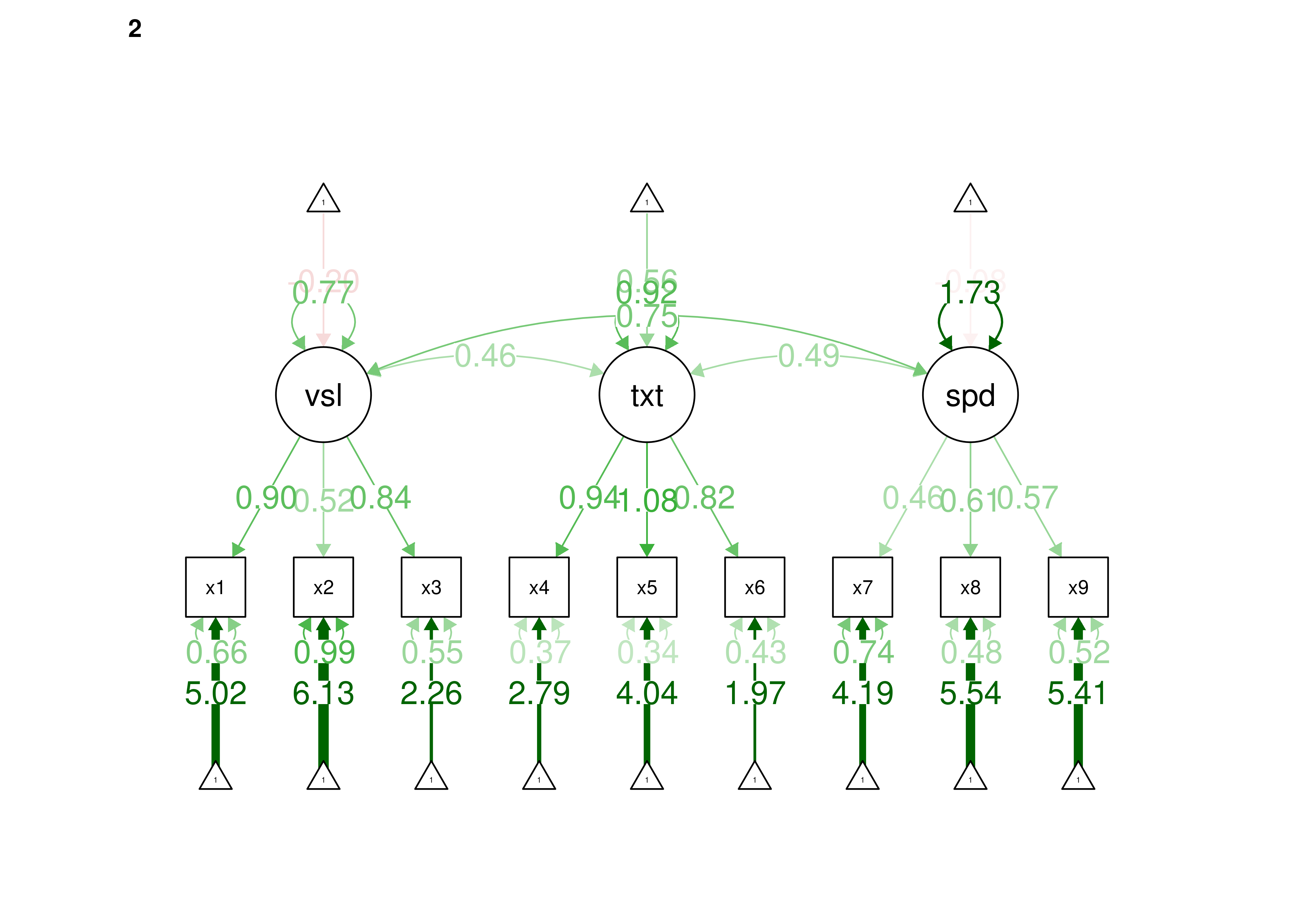
Figure 16.58: Scalar Invariance Model in Confirmatory Factor Analysis.
16.10.7 Residual (“Strict Factorial”) Invariance Model
Specify invariance of factor loadings, intercepts, and residuals across groups.
16.10.7.1 Model Syntax
Code
## LOADINGS:
visual =~ c(NA, NA)*x1 + c(lambda.1_1, lambda.1_1)*x1
visual =~ c(NA, NA)*x2 + c(lambda.2_1, lambda.2_1)*x2
visual =~ c(NA, NA)*x3 + c(lambda.3_1, lambda.3_1)*x3
textual =~ c(NA, NA)*x4 + c(lambda.4_2, lambda.4_2)*x4
textual =~ c(NA, NA)*x5 + c(lambda.5_2, lambda.5_2)*x5
textual =~ c(NA, NA)*x6 + c(lambda.6_2, lambda.6_2)*x6
speed =~ c(NA, NA)*x7 + c(lambda.7_3, lambda.7_3)*x7
speed =~ c(NA, NA)*x8 + c(lambda.8_3, lambda.8_3)*x8
speed =~ c(NA, NA)*x9 + c(lambda.9_3, lambda.9_3)*x9
## INTERCEPTS:
x1 ~ c(NA, NA)*1 + c(nu.1, nu.1)*1
x2 ~ c(NA, NA)*1 + c(nu.2, nu.2)*1
x3 ~ c(NA, NA)*1 + c(nu.3, nu.3)*1
x4 ~ c(NA, NA)*1 + c(nu.4, nu.4)*1
x5 ~ c(NA, NA)*1 + c(nu.5, nu.5)*1
x6 ~ c(NA, NA)*1 + c(nu.6, nu.6)*1
x7 ~ c(NA, NA)*1 + c(nu.7, nu.7)*1
x8 ~ c(NA, NA)*1 + c(nu.8, nu.8)*1
x9 ~ c(NA, NA)*1 + c(nu.9, nu.9)*1
## UNIQUE-FACTOR VARIANCES:
x1 ~~ c(NA, NA)*x1 + c(theta.1_1, theta.1_1)*x1
x2 ~~ c(NA, NA)*x2 + c(theta.2_2, theta.2_2)*x2
x3 ~~ c(NA, NA)*x3 + c(theta.3_3, theta.3_3)*x3
x4 ~~ c(NA, NA)*x4 + c(theta.4_4, theta.4_4)*x4
x5 ~~ c(NA, NA)*x5 + c(theta.5_5, theta.5_5)*x5
x6 ~~ c(NA, NA)*x6 + c(theta.6_6, theta.6_6)*x6
x7 ~~ c(NA, NA)*x7 + c(theta.7_7, theta.7_7)*x7
x8 ~~ c(NA, NA)*x8 + c(theta.8_8, theta.8_8)*x8
x9 ~~ c(NA, NA)*x9 + c(theta.9_9, theta.9_9)*x9
## LATENT MEANS/INTERCEPTS:
visual ~ c(0, NA)*1 + c(alpha.1.g1, alpha.1.g2)*1
textual ~ c(0, NA)*1 + c(alpha.2.g1, alpha.2.g2)*1
speed ~ c(0, NA)*1 + c(alpha.3.g1, alpha.3.g2)*1
## COMMON-FACTOR VARIANCES:
visual ~~ c(1, NA)*visual + c(psi.1_1.g1, psi.1_1.g2)*visual
textual ~~ c(1, NA)*textual + c(psi.2_2.g1, psi.2_2.g2)*textual
speed ~~ c(1, NA)*speed + c(psi.3_3.g1, psi.3_3.g2)*speed
## COMMON-FACTOR COVARIANCES:
visual ~~ c(NA, NA)*textual + c(psi.2_1.g1, psi.2_1.g2)*textual
visual ~~ c(NA, NA)*speed + c(psi.3_1.g1, psi.3_1.g2)*speed
textual ~~ c(NA, NA)*speed + c(psi.3_2.g1, psi.3_2.g2)*speed
16.10.7.1.1 Summary of Model Features
This lavaan model syntax specifies a CFA with 9 manifest indicators of 3 common factor(s).
To identify the location and scale of each common factor, the factor means and variances were fixed to 0 and 1, respectively, unless equality constraints on measurement parameters allow them to be freed.
Pattern matrix indicating num(eric), ord(ered), and lat(ent) indicators per factor:
visual textual speed
x1 num
x2 num
x3 num
x4 num
x5 num
x6 num
x7 num
x8 num
x9 num
The following types of parameter were constrained to equality across groups:
loadings
intercepts
residuals
16.10.7.4 Model Summary
Code
lavaan 0.6-19 ended normally after 62 iterations
Estimator ML
Optimization method NLMINB
Number of model parameters 66
Number of equality constraints 27
Number of observations per group:
Pasteur 156
Grant-White 145
Number of missing patterns per group:
Pasteur 9
Grant-White 9
Model Test User Model:
Standard Scaled
Test Statistic 172.960 172.714
Degrees of freedom 69 69
P-value (Chi-square) 0.000 0.000
Scaling correction factor 1.001
Yuan-Bentler correction (Mplus variant)
Test statistic for each group:
Pasteur 87.707 87.707
Grant-White 85.007 85.007
Model Test Baseline Model:
Test statistic 932.665 909.019
Degrees of freedom 72 72
P-value 0.000 0.000
Scaling correction factor 1.026
User Model versus Baseline Model:
Comparative Fit Index (CFI) 0.879 0.876
Tucker-Lewis Index (TLI) 0.874 0.871
Robust Comparative Fit Index (CFI) 0.883
Robust Tucker-Lewis Index (TLI) 0.878
Loglikelihood and Information Criteria:
Loglikelihood user model (H0) -3680.600 -3680.600
Scaling correction factor 0.645
for the MLR correction
Loglikelihood unrestricted model (H1) -3594.120 -3594.120
Scaling correction factor 1.034
for the MLR correction
Akaike (AIC) 7439.201 7439.201
Bayesian (BIC) 7583.778 7583.778
Sample-size adjusted Bayesian (SABIC) 7460.092 7460.092
Root Mean Square Error of Approximation:
RMSEA 0.100 0.100
90 Percent confidence interval - lower 0.082 0.081
90 Percent confidence interval - upper 0.119 0.119
P-value H_0: RMSEA <= 0.050 0.000 0.000
P-value H_0: RMSEA >= 0.080 0.962 0.961
Robust RMSEA 0.100
90 Percent confidence interval - lower 0.081
90 Percent confidence interval - upper 0.119
P-value H_0: Robust RMSEA <= 0.050 0.000
P-value H_0: Robust RMSEA >= 0.080 0.955
Standardized Root Mean Square Residual:
SRMR 0.086 0.086
Parameter Estimates:
Standard errors Sandwich
Information bread Observed
Observed information based on Hessian
Group 1 [Pasteur]:
Latent Variables:
Estimate Std.Err z-value P(>|z|) Std.lv Std.all
visual =~
x1 (l.1_) 0.862 0.126 6.855 0.000 0.862 0.728
x2 (l.2_) 0.516 0.091 5.678 0.000 0.516 0.436
x3 (l.3_) 0.745 0.112 6.630 0.000 0.745 0.651
textual =~
x4 (l.4_) 0.928 0.071 13.094 0.000 0.928 0.828
x5 (l.5_) 1.069 0.076 14.113 0.000 1.069 0.855
x6 (l.6_) 0.882 0.062 14.125 0.000 0.882 0.830
speed =~
x7 (l.7_) 0.577 0.087 6.658 0.000 0.577 0.544
x8 (l.8_) 0.669 0.083 8.109 0.000 0.669 0.688
x9 (l.9_) 0.632 0.085 7.461 0.000 0.632 0.649
Covariances:
Estimate Std.Err z-value P(>|z|) Std.lv Std.all
visual ~~
textul (p.2_) 0.430 0.124 3.472 0.001 0.430 0.430
speed (p.3_1) 0.351 0.129 2.710 0.007 0.351 0.351
textual ~~
speed (p.3_2) 0.302 0.093 3.250 0.001 0.302 0.302
Intercepts:
Estimate Std.Err z-value P(>|z|) Std.lv Std.all
.x1 (nu.1) 5.025 0.095 52.938 0.000 5.025 4.243
.x2 (nu.2) 6.138 0.086 71.711 0.000 6.138 5.188
.x3 (nu.3) 2.332 0.099 23.546 0.000 2.332 2.039
.x4 (nu.4) 2.784 0.086 32.453 0.000 2.784 2.483
.x5 (nu.5) 4.030 0.102 39.369 0.000 4.030 3.224
.x6 (nu.6) 1.928 0.075 25.828 0.000 1.928 1.815
.x7 (nu.7) 4.270 0.076 55.911 0.000 4.270 4.027
.x8 (nu.8) 5.619 0.075 74.597 0.000 5.619 5.775
.x9 (nu.9) 5.470 0.073 74.756 0.000 5.470 5.616
visual (a.1.) 0.000 0.000 0.000
textual (a.2.) 0.000 0.000 0.000
speed (a.3.) 0.000 0.000 0.000
Variances:
Estimate Std.Err z-value P(>|z|) Std.lv Std.all
.x1 (t.1_) 0.660 0.165 4.008 0.000 0.660 0.470
.x2 (t.2_) 1.133 0.110 10.337 0.000 1.133 0.810
.x3 (t.3_) 0.754 0.131 5.749 0.000 0.754 0.576
.x4 (t.4_) 0.396 0.050 7.904 0.000 0.396 0.315
.x5 (t.5_) 0.420 0.057 7.385 0.000 0.420 0.269
.x6 (t.6_) 0.350 0.048 7.311 0.000 0.350 0.310
.x7 (t.7_) 0.792 0.094 8.413 0.000 0.792 0.704
.x8 (t.8_) 0.499 0.095 5.244 0.000 0.499 0.527
.x9 (t.9_) 0.549 0.100 5.479 0.000 0.549 0.579
visual (p.1_) 1.000 1.000 1.000
textual (p.2_) 1.000 1.000 1.000
speed (p.3_) 1.000 1.000 1.000
R-Square:
Estimate
x1 0.530
x2 0.190
x3 0.424
x4 0.685
x5 0.731
x6 0.690
x7 0.296
x8 0.473
x9 0.421
Group 2 [Grant-White]:
Latent Variables:
Estimate Std.Err z-value P(>|z|) Std.lv Std.all
visual =~
x1 (l.1_) 0.862 0.126 6.855 0.000 0.796 0.700
x2 (l.2_) 0.516 0.091 5.678 0.000 0.476 0.408
x3 (l.3_) 0.745 0.112 6.630 0.000 0.687 0.621
textual =~
x4 (l.4_) 0.928 0.071 13.094 0.000 0.926 0.827
x5 (l.5_) 1.069 0.076 14.113 0.000 1.068 0.855
x6 (l.6_) 0.882 0.062 14.125 0.000 0.881 0.830
speed =~
x7 (l.7_) 0.577 0.087 6.658 0.000 0.670 0.601
x8 (l.8_) 0.669 0.083 8.109 0.000 0.777 0.740
x9 (l.9_) 0.632 0.085 7.461 0.000 0.733 0.703
Covariances:
Estimate Std.Err z-value P(>|z|) Std.lv Std.all
visual ~~
textul (p.2_) 0.519 0.135 3.852 0.000 0.563 0.563
speed (p.3_1) 0.643 0.202 3.183 0.001 0.600 0.600
textual ~~
speed (p.3_2) 0.426 0.181 2.351 0.019 0.368 0.368
Intercepts:
Estimate Std.Err z-value P(>|z|) Std.lv Std.all
.x1 (nu.1) 5.025 0.095 52.938 0.000 5.025 4.420
.x2 (nu.2) 6.138 0.086 71.711 0.000 6.138 5.263
.x3 (nu.3) 2.332 0.099 23.546 0.000 2.332 2.106
.x4 (nu.4) 2.784 0.086 32.453 0.000 2.784 2.486
.x5 (nu.5) 4.030 0.102 39.369 0.000 4.030 3.228
.x6 (nu.6) 1.928 0.075 25.828 0.000 1.928 1.817
.x7 (nu.7) 4.270 0.076 55.911 0.000 4.270 3.835
.x8 (nu.8) 5.619 0.075 74.597 0.000 5.619 5.353
.x9 (nu.9) 5.470 0.073 74.756 0.000 5.470 5.247
visual (a.1.) -0.208 0.181 -1.149 0.251 -0.225 -0.225
textual (a.2.) 0.611 0.132 4.646 0.000 0.612 0.612
speed (a.3.) -0.283 0.155 -1.824 0.068 -0.244 -0.244
Variances:
Estimate Std.Err z-value P(>|z|) Std.lv Std.all
.x1 (t.1_) 0.660 0.165 4.008 0.000 0.660 0.510
.x2 (t.2_) 1.133 0.110 10.337 0.000 1.133 0.833
.x3 (t.3_) 0.754 0.131 5.749 0.000 0.754 0.615
.x4 (t.4_) 0.396 0.050 7.904 0.000 0.396 0.316
.x5 (t.5_) 0.420 0.057 7.385 0.000 0.420 0.269
.x6 (t.6_) 0.350 0.048 7.311 0.000 0.350 0.311
.x7 (t.7_) 0.792 0.094 8.413 0.000 0.792 0.638
.x8 (t.8_) 0.499 0.095 5.244 0.000 0.499 0.453
.x9 (t.9_) 0.549 0.100 5.479 0.000 0.549 0.505
visual (p.1_) 0.852 0.217 3.932 0.000 1.000 1.000
textual (p.2_) 0.997 0.193 5.160 0.000 1.000 1.000
speed (p.3_) 1.346 0.328 4.101 0.000 1.000 1.000
R-Square:
Estimate
x1 0.490
x2 0.167
x3 0.385
x4 0.684
x5 0.731
x6 0.689
x7 0.362
x8 0.547
x9 0.495
Code
lavaan 0.6-19 ended normally after 59 iterations
Estimator ML
Optimization method NLMINB
Number of model parameters 66
Number of equality constraints 27
Number of observations per group:
Pasteur 156
Grant-White 145
Number of missing patterns per group:
Pasteur 9
Grant-White 9
Model Test User Model:
Standard Scaled
Test Statistic 172.960 172.714
Degrees of freedom 69 69
P-value (Chi-square) 0.000 0.000
Scaling correction factor 1.001
Yuan-Bentler correction (Mplus variant)
Test statistic for each group:
Pasteur 87.707 87.707
Grant-White 85.007 85.007
Model Test Baseline Model:
Test statistic 932.665 909.019
Degrees of freedom 72 72
P-value 0.000 0.000
Scaling correction factor 1.026
User Model versus Baseline Model:
Comparative Fit Index (CFI) 0.879 0.876
Tucker-Lewis Index (TLI) 0.874 0.871
Robust Comparative Fit Index (CFI) 0.883
Robust Tucker-Lewis Index (TLI) 0.878
Loglikelihood and Information Criteria:
Loglikelihood user model (H0) -3680.600 -3680.600
Scaling correction factor 0.645
for the MLR correction
Loglikelihood unrestricted model (H1) -3594.120 -3594.120
Scaling correction factor 1.034
for the MLR correction
Akaike (AIC) 7439.201 7439.201
Bayesian (BIC) 7583.778 7583.778
Sample-size adjusted Bayesian (SABIC) 7460.092 7460.092
Root Mean Square Error of Approximation:
RMSEA 0.100 0.100
90 Percent confidence interval - lower 0.082 0.081
90 Percent confidence interval - upper 0.119 0.119
P-value H_0: RMSEA <= 0.050 0.000 0.000
P-value H_0: RMSEA >= 0.080 0.962 0.961
Robust RMSEA 0.100
90 Percent confidence interval - lower 0.081
90 Percent confidence interval - upper 0.119
P-value H_0: Robust RMSEA <= 0.050 0.000
P-value H_0: Robust RMSEA >= 0.080 0.955
Standardized Root Mean Square Residual:
SRMR 0.086 0.086
Parameter Estimates:
Standard errors Sandwich
Information bread Observed
Observed information based on Hessian
Group 1 [Pasteur]:
Latent Variables:
Estimate Std.Err z-value P(>|z|) Std.lv Std.all
visual =~
x1 (lmb1) 0.862 0.126 6.855 0.000 0.862 0.728
x2 (lmb2) 0.516 0.091 5.678 0.000 0.516 0.436
x3 (lmb3) 0.745 0.112 6.630 0.000 0.745 0.651
textual =~
x4 (lmb4) 0.928 0.071 13.094 0.000 0.928 0.828
x5 (lmb5) 1.069 0.076 14.113 0.000 1.069 0.855
x6 (lmb6) 0.882 0.062 14.125 0.000 0.882 0.830
speed =~
x7 (lmb7) 0.577 0.087 6.658 0.000 0.577 0.544
x8 (lmb8) 0.669 0.083 8.110 0.000 0.669 0.688
x9 (lmb9) 0.632 0.085 7.461 0.000 0.632 0.649
Covariances:
Estimate Std.Err z-value P(>|z|) Std.lv Std.all
visual ~~
textual 0.430 0.124 3.472 0.001 0.430 0.430
speed 0.351 0.129 2.710 0.007 0.351 0.351
textual ~~
speed 0.302 0.093 3.250 0.001 0.302 0.302
Intercepts:
Estimate Std.Err z-value P(>|z|) Std.lv Std.all
visual 0.000 0.000 0.000
textual 0.000 0.000 0.000
speed 0.000 0.000 0.000
.x1 (int1) 5.025 0.095 52.938 0.000 5.025 4.243
.x2 (int2) 6.138 0.086 71.710 0.000 6.138 5.188
.x3 (int3) 2.332 0.099 23.546 0.000 2.332 2.039
.x4 (int4) 2.784 0.086 32.453 0.000 2.784 2.483
.x5 (int5) 4.030 0.102 39.369 0.000 4.030 3.224
.x6 (int6) 1.928 0.075 25.828 0.000 1.928 1.815
.x7 (int7) 4.270 0.076 55.911 0.000 4.270 4.027
.x8 (int8) 5.619 0.075 74.598 0.000 5.619 5.775
.x9 (int9) 5.470 0.073 74.756 0.000 5.470 5.616
Variances:
Estimate Std.Err z-value P(>|z|) Std.lv Std.all
visual 1.000 1.000 1.000
textual 1.000 1.000 1.000
speed 1.000 1.000 1.000
.x1 (rsd1) 0.660 0.165 4.008 0.000 0.660 0.470
.x2 (rsd2) 1.133 0.110 10.337 0.000 1.133 0.810
.x3 (rsd3) 0.754 0.131 5.749 0.000 0.754 0.576
.x4 (rsd4) 0.396 0.050 7.904 0.000 0.396 0.315
.x5 (rsd5) 0.420 0.057 7.385 0.000 0.420 0.269
.x6 (rsd6) 0.350 0.048 7.311 0.000 0.350 0.310
.x7 (rsd7) 0.792 0.094 8.414 0.000 0.792 0.704
.x8 (rsd8) 0.499 0.095 5.244 0.000 0.499 0.527
.x9 (rsd9) 0.549 0.100 5.479 0.000 0.549 0.579
R-Square:
Estimate
x1 0.530
x2 0.190
x3 0.424
x4 0.685
x5 0.731
x6 0.690
x7 0.296
x8 0.473
x9 0.421
Group 2 [Grant-White]:
Latent Variables:
Estimate Std.Err z-value P(>|z|) Std.lv Std.all
visual =~
x1 (lmb1) 0.862 0.126 6.855 0.000 0.796 0.700
x2 (lmb2) 0.516 0.091 5.678 0.000 0.476 0.408
x3 (lmb3) 0.745 0.112 6.630 0.000 0.687 0.621
textual =~
x4 (lmb4) 0.928 0.071 13.094 0.000 0.926 0.827
x5 (lmb5) 1.069 0.076 14.113 0.000 1.068 0.855
x6 (lmb6) 0.882 0.062 14.125 0.000 0.881 0.830
speed =~
x7 (lmb7) 0.577 0.087 6.658 0.000 0.670 0.601
x8 (lmb8) 0.669 0.083 8.110 0.000 0.777 0.740
x9 (lmb9) 0.632 0.085 7.461 0.000 0.733 0.703
Covariances:
Estimate Std.Err z-value P(>|z|) Std.lv Std.all
visual ~~
textual 0.519 0.135 3.852 0.000 0.563 0.563
speed 0.643 0.202 3.183 0.001 0.600 0.600
textual ~~
speed 0.426 0.181 2.351 0.019 0.368 0.368
Intercepts:
Estimate Std.Err z-value P(>|z|) Std.lv Std.all
visual -0.208 0.181 -1.149 0.251 -0.225 -0.225
textual 0.611 0.132 4.646 0.000 0.612 0.612
speed -0.283 0.155 -1.824 0.068 -0.244 -0.244
.x1 (int1) 5.025 0.095 52.938 0.000 5.025 4.420
.x2 (int2) 6.138 0.086 71.710 0.000 6.138 5.263
.x3 (int3) 2.332 0.099 23.546 0.000 2.332 2.106
.x4 (int4) 2.784 0.086 32.453 0.000 2.784 2.486
.x5 (int5) 4.030 0.102 39.369 0.000 4.030 3.228
.x6 (int6) 1.928 0.075 25.828 0.000 1.928 1.817
.x7 (int7) 4.270 0.076 55.911 0.000 4.270 3.835
.x8 (int8) 5.619 0.075 74.598 0.000 5.619 5.353
.x9 (int9) 5.470 0.073 74.756 0.000 5.470 5.247
Variances:
Estimate Std.Err z-value P(>|z|) Std.lv Std.all
visual 0.852 0.217 3.932 0.000 1.000 1.000
textual 0.997 0.193 5.160 0.000 1.000 1.000
speed 1.346 0.328 4.101 0.000 1.000 1.000
.x1 (rsd1) 0.660 0.165 4.008 0.000 0.660 0.510
.x2 (rsd2) 1.133 0.110 10.337 0.000 1.133 0.833
.x3 (rsd3) 0.754 0.131 5.749 0.000 0.754 0.615
.x4 (rsd4) 0.396 0.050 7.904 0.000 0.396 0.316
.x5 (rsd5) 0.420 0.057 7.385 0.000 0.420 0.269
.x6 (rsd6) 0.350 0.048 7.311 0.000 0.350 0.311
.x7 (rsd7) 0.792 0.094 8.414 0.000 0.792 0.638
.x8 (rsd8) 0.499 0.095 5.244 0.000 0.499 0.453
.x9 (rsd9) 0.549 0.100 5.479 0.000 0.549 0.505
R-Square:
Estimate
x1 0.490
x2 0.167
x3 0.385
x4 0.684
x5 0.731
x6 0.689
x7 0.362
x8 0.547
x9 0.495
16.10.7.5 Model Fit
You can specify the null model as the baseline model using: baseline.model = nullModelFit
npar fmin
39.000 0.287
chisq df
172.960 69.000
pvalue chisq.scaled
0.000 172.714
df.scaled pvalue.scaled
69.000 0.000
chisq.scaling.factor baseline.chisq
1.001 932.665
baseline.df baseline.pvalue
72.000 0.000
baseline.chisq.scaled baseline.df.scaled
909.019 72.000
baseline.pvalue.scaled baseline.chisq.scaling.factor
0.000 1.026
cfi tli
0.879 0.874
cfi.scaled tli.scaled
0.876 0.871
cfi.robust tli.robust
0.883 0.878
nnfi rfi
0.874 0.806
nfi pnfi
0.815 0.781
ifi rni
0.880 0.879
nnfi.scaled rfi.scaled
0.871 0.802
nfi.scaled pnfi.scaled
0.810 0.776
ifi.scaled rni.scaled
0.877 0.876
nnfi.robust rni.robust
0.878 0.883
logl unrestricted.logl
-3680.600 -3594.120
aic bic
7439.201 7583.778
ntotal bic2
301.000 7460.092
scaling.factor.h1 scaling.factor.h0
1.034 0.645
rmsea rmsea.ci.lower
0.100 0.082
rmsea.ci.upper rmsea.ci.level
0.119 0.900
rmsea.pvalue rmsea.close.h0
0.000 0.050
rmsea.notclose.pvalue rmsea.notclose.h0
0.962 0.080
rmsea.scaled rmsea.ci.lower.scaled
0.100 0.081
rmsea.ci.upper.scaled rmsea.pvalue.scaled
0.119 0.000
rmsea.notclose.pvalue.scaled rmsea.robust
0.961 0.100
rmsea.ci.lower.robust rmsea.ci.upper.robust
0.081 0.119
rmsea.pvalue.robust rmsea.notclose.pvalue.robust
0.000 0.955
rmr rmr_nomean
0.103 0.102
srmr srmr_bentler
0.086 0.086
srmr_bentler_nomean crmr
0.083 0.086
crmr_nomean srmr_mplus
0.083 0.104
srmr_mplus_nomean cn_05
0.084 156.566
cn_01 gfi
173.684 0.992
agfi pgfi
0.988 0.634
mfi ecvi
0.841 0.834
Code
residualInvarianceModelFitIndices <- fitMeasures(
residualInvarianceModel_fit)[c(
"cfi.robust", "rmsea.robust", "srmr")]
residualInvarianceModel_chisquare <- fitMeasures(
residualInvarianceModel_fit)[c("chisq.scaled")]
residualInvarianceModel_chisquareScaling <- fitMeasures(
residualInvarianceModel_fit)[c("chisq.scaling.factor")]
residualInvarianceModel_df <- fitMeasures(
residualInvarianceModel_fit)[c("df.scaled")]
residualInvarianceModel_N <- lavInspect(
residualInvarianceModel_fit,
what = "ntotal")
16.10.7.6 Compare Model Fit
16.10.7.6.1 Nested Model (\(\chi^2\)) Difference Test
The scalar invariance model and the residual invariance model are considered “nested” models. The residual invariance model is nested within the scalar invariance model because the scalar invariance model includes all of the terms of the residual invariance model along with additional terms. Model fit of nested models can be compared with a chi-square difference test, also known as a likelihood ratio test or deviance test. A significant chi-square difference test would indicate that the simplified model with additional constraints (the residual invariance model) is significantly worse fitting than the more complex model that has fewer constraints (the scalar invariance model).
In this instance, you do not need to examine whether residual invariance held because the preceding level of measurement invariance (scalar invariance) did not hold.
The petersenlab
package (Petersen, 2024b) contains the satorraBentlerScaledChiSquareDifferenceTestStatistic()
function that performs a Satorra-Bentler scaled chi-square difference test:
Code
residualInvarianceModel_chisquareDiff <-
satorraBentlerScaledChiSquareDifferenceTestStatistic(
T0 = residualInvarianceModel_chisquare,
c0 = residualInvarianceModel_chisquareScaling,
d0 = residualInvarianceModel_df,
T1 = scalarInvarianceModel_chisquare,
c1 = scalarInvarianceModel_chisquareScaling,
d1 = scalarInvarianceModel_df)
residualInvarianceModel_chisquareDiff
chisq.scaled
14.35586
16.10.7.6.3 Score-Based Test
Score-based tests of measurement invariance are implemented using the strucchange
package and are described by T. Wang et al. (2014).
lambda.1_1 lambda.2_1 lambda.3_1 lambda.4_2 lambda.5_2 lambda.6_2 lambda.7_3
0.862 0.516 0.745 0.928 1.069 0.882 0.577
lambda.8_3 lambda.9_3 nu.1 nu.2 nu.3 nu.4 nu.5
0.669 0.632 5.025 6.138 2.332 2.784 4.030
nu.6 nu.7 nu.8 nu.9 theta.1_1 theta.2_2 theta.3_3
1.928 4.270 5.619 5.470 0.660 1.133 0.754
theta.4_4 theta.5_5 theta.6_6 theta.7_7 theta.8_8 theta.9_9 psi.2_1.g1
0.396 0.420 0.350 0.792 0.499 0.549 0.430
psi.3_1.g1 psi.3_2.g1 lambda.1_1 lambda.2_1 lambda.3_1 lambda.4_2 lambda.5_2
0.351 0.302 0.862 0.516 0.745 0.928 1.069
lambda.6_2 lambda.7_3 lambda.8_3 lambda.9_3 nu.1 nu.2 nu.3
0.882 0.577 0.669 0.632 5.025 6.138 2.332
nu.4 nu.5 nu.6 nu.7 nu.8 nu.9 theta.1_1
2.784 4.030 1.928 4.270 5.619 5.470 0.660
theta.2_2 theta.3_3 theta.4_4 theta.5_5 theta.6_6 theta.7_7 theta.8_8
1.133 0.754 0.396 0.420 0.350 0.792 0.499
theta.9_9 alpha.1.g2 alpha.2.g2 alpha.3.g2 psi.1_1.g2 psi.2_2.g2 psi.3_3.g2
0.549 -0.208 0.611 -0.283 0.852 0.997 1.346
psi.2_1.g2 psi.3_1.g2 psi.3_2.g2
0.519 0.643 0.426
theta.1_1 theta.2_2 theta.3_3 theta.4_4 theta.5_5 theta.6_6 theta.7_7 theta.8_8
0.6598353 1.1333770 0.7536794 0.3957809 0.4196626 0.3504637 0.7916721 0.4987961
theta.9_9
0.5490913
Code
M-fluctuation test
data: residualInvarianceModel_fit
f(efp) = 10.64, p-value = 0.3012
A score-based test and expected parameter change (EPC) estimates (Oberski, 2014; Oberski et al., 2015) are provided by the lavaan
package (Rosseel et al., 2022).
$test
total score test:
test X2 df p.value
1 score 60.136 27 0
$uni
univariate score tests:
lhs op rhs X2 df p.value
1 .p1. == .p37. 0.011 1 0.915
2 .p2. == .p38. 0.043 1 0.836
3 .p3. == .p39. 0.062 1 0.803
4 .p4. == .p40. 0.089 1 0.766
5 .p5. == .p41. 4.083 1 0.043
6 .p6. == .p42. 5.815 1 0.016
7 .p7. == .p43. 0.026 1 0.872
8 .p8. == .p44. 0.003 1 0.954
9 .p9. == .p45. 0.006 1 0.939
10 .p10. == .p46. 6.331 1 0.012
11 .p11. == .p47. 7.812 1 0.005
12 .p12. == .p48. 20.213 1 0.000
13 .p13. == .p49. 1.231 1 0.267
14 .p14. == .p50. 1.155 1 0.283
15 .p15. == .p51. 0.001 1 0.982
16 .p16. == .p52. 14.986 1 0.000
17 .p17. == .p53. 3.574 1 0.059
18 .p18. == .p54. 1.735 1 0.188
19 .p19. == .p55. 0.055 1 0.815
20 .p20. == .p56. 2.026 1 0.155
21 .p21. == .p57. 1.867 1 0.172
22 .p22. == .p58. 0.754 1 0.385
23 .p23. == .p59. 0.619 1 0.432
24 .p24. == .p60. 3.852 1 0.050
25 .p25. == .p61. 2.273 1 0.132
26 .p26. == .p62. 0.674 1 0.412
27 .p27. == .p63. 1.954 1 0.162
$epc
expected parameter changes (epc) and expected parameter values (epv):
lhs op rhs block group free label plabel est epc epv
1 visual =~ x1 1 1 1 lambda.1_1 .p1. 0.862 0.083 0.945
2 visual =~ x2 1 1 2 lambda.2_1 .p2. 0.516 -0.017 0.499
3 visual =~ x3 1 1 3 lambda.3_1 .p3. 0.745 -0.095 0.650
4 textual =~ x4 1 1 4 lambda.4_2 .p4. 0.928 -0.006 0.922
5 textual =~ x5 1 1 5 lambda.5_2 .p5. 1.069 0.060 1.130
6 textual =~ x6 1 1 6 lambda.6_2 .p6. 0.882 -0.054 0.828
7 speed =~ x7 1 1 7 lambda.7_3 .p7. 0.577 -0.036 0.541
8 speed =~ x8 1 1 8 lambda.8_3 .p8. 0.669 0.006 0.676
9 speed =~ x9 1 1 9 lambda.9_3 .p9. 0.632 -0.028 0.604
10 x1 ~1 1 1 10 nu.1 .p10. 5.025 -0.076 4.949
11 x2 ~1 1 1 11 nu.2 .p11. 6.138 -0.154 5.984
12 x3 ~1 1 1 12 nu.3 .p12. 2.332 0.177 2.509
13 x4 ~1 1 1 13 nu.4 .p13. 2.784 0.030 2.814
14 x5 ~1 1 1 14 nu.5 .p14. 4.030 -0.029 4.002
15 x6 ~1 1 1 15 nu.6 .p15. 1.928 0.001 1.929
16 x7 ~1 1 1 16 nu.7 .p16. 4.270 0.168 4.439
17 x8 ~1 1 1 17 nu.8 .p17. 5.619 -0.055 5.564
18 x9 ~1 1 1 18 nu.9 .p18. 5.470 -0.043 5.427
19 x1 ~~ x1 1 1 19 theta.1_1 .p19. 0.660 -0.145 0.514
20 x2 ~~ x2 1 1 20 theta.2_2 .p20. 1.133 0.145 1.279
21 x3 ~~ x3 1 1 21 theta.3_3 .p21. 0.754 0.194 0.947
22 x4 ~~ x4 1 1 22 theta.4_4 .p22. 0.396 0.046 0.442
23 x5 ~~ x5 1 1 23 theta.5_5 .p23. 0.420 0.009 0.428
24 x6 ~~ x6 1 1 24 theta.6_6 .p24. 0.350 -0.059 0.291
25 x7 ~~ x7 1 1 25 theta.7_7 .p25. 0.792 0.115 0.906
26 x8 ~~ x8 1 1 26 theta.8_8 .p26. 0.499 0.016 0.515
27 x9 ~~ x9 1 1 27 theta.9_9 .p27. 0.549 0.084 0.633
28 visual ~1 1 1 0 alpha.1.g1 .p28. 0.000 NA NA
29 textual ~1 1 1 0 alpha.2.g1 .p29. 0.000 NA NA
30 speed ~1 1 1 0 alpha.3.g1 .p30. 0.000 NA NA
31 visual ~~ visual 1 1 0 psi.1_1.g1 .p31. 1.000 NA NA
32 textual ~~ textual 1 1 0 psi.2_2.g1 .p32. 1.000 NA NA
33 speed ~~ speed 1 1 0 psi.3_3.g1 .p33. 1.000 NA NA
34 visual ~~ textual 1 1 28 psi.2_1.g1 .p34. 0.430 -0.002 0.428
35 visual ~~ speed 1 1 29 psi.3_1.g1 .p35. 0.351 0.007 0.358
36 textual ~~ speed 1 1 30 psi.3_2.g1 .p36. 0.302 0.007 0.309
37 visual =~ x1 2 2 31 lambda.1_1 .p37. 0.862 0.000 0.862
38 visual =~ x2 2 2 32 lambda.2_1 .p38. 0.516 0.063 0.579
39 visual =~ x3 2 2 33 lambda.3_1 .p39. 0.745 -0.024 0.721
40 textual =~ x4 2 2 34 lambda.4_2 .p40. 0.928 0.036 0.963
41 textual =~ x5 2 2 35 lambda.5_2 .p41. 1.069 -0.091 0.978
42 textual =~ x6 2 2 36 lambda.6_2 .p42. 0.882 0.061 0.944
43 speed =~ x7 2 2 37 lambda.7_3 .p43. 0.577 -0.035 0.542
44 speed =~ x8 2 2 38 lambda.8_3 .p44. 0.669 0.020 0.689
45 speed =~ x9 2 2 39 lambda.9_3 .p45. 0.632 0.033 0.665
46 x1 ~1 2 2 40 nu.1 .p46. 5.025 0.075 5.100
47 x2 ~1 2 2 41 nu.2 .p47. 6.138 0.176 6.314
48 x3 ~1 2 2 42 nu.3 .p48. 2.332 -0.197 2.135
49 x4 ~1 2 2 43 nu.4 .p49. 2.784 -0.057 2.726
50 x5 ~1 2 2 44 nu.5 .p50. 4.030 0.085 4.115
51 x6 ~1 2 2 45 nu.6 .p51. 1.928 -0.040 1.888
52 x7 ~1 2 2 46 nu.7 .p52. 4.270 -0.185 4.086
53 x8 ~1 2 2 47 nu.8 .p53. 5.619 0.074 5.694
54 x9 ~1 2 2 48 nu.9 .p54. 5.470 0.065 5.535
55 x1 ~~ x1 2 2 49 theta.1_1 .p55. 0.660 0.021 0.681
56 x2 ~~ x2 2 2 50 theta.2_2 .p56. 1.133 -0.162 0.971
57 x3 ~~ x3 2 2 51 theta.3_3 .p57. 0.754 -0.091 0.663
58 x4 ~~ x4 2 2 52 theta.4_4 .p58. 0.396 -0.064 0.332
59 x5 ~~ x5 2 2 53 theta.5_5 .p59. 0.420 0.009 0.428
60 x6 ~~ x6 2 2 54 theta.6_6 .p60. 0.350 0.062 0.412
61 x7 ~~ x7 2 2 55 theta.7_7 .p61. 0.792 -0.088 0.704
62 x8 ~~ x8 2 2 56 theta.8_8 .p62. 0.499 -0.044 0.455
63 x9 ~~ x9 2 2 57 theta.9_9 .p63. 0.549 -0.095 0.454
64 visual ~1 2 2 58 alpha.1.g2 .p64. -0.208 0.009 -0.200
65 textual ~1 2 2 59 alpha.2.g2 .p65. 0.611 0.002 0.614
66 speed ~1 2 2 60 alpha.3.g2 .p66. -0.283 -0.016 -0.298
67 visual ~~ visual 2 2 61 psi.1_1.g2 .p67. 0.852 0.016 0.868
68 textual ~~ textual 2 2 62 psi.2_2.g2 .p68. 0.997 -0.004 0.993
69 speed ~~ speed 2 2 63 psi.3_3.g2 .p69. 1.346 0.008 1.354
70 visual ~~ textual 2 2 64 psi.2_1.g2 .p70. 0.519 -0.002 0.517
71 visual ~~ speed 2 2 65 psi.3_1.g2 .p71. 0.643 -0.014 0.629
72 textual ~~ speed 2 2 66 psi.3_2.g2 .p72. 0.426 -0.009 0.417
sepc.lv sepc.all sepc.nox
1 0.083 0.070 0.070
2 -0.017 -0.014 -0.014
3 -0.095 -0.083 -0.083
4 -0.006 -0.005 -0.005
5 0.060 0.048 0.048
6 -0.054 -0.051 -0.051
7 -0.036 -0.034 -0.034
8 0.006 0.006 0.006
9 -0.028 -0.029 -0.029
10 -0.076 -0.065 -0.065
11 -0.154 -0.130 -0.130
12 0.177 0.155 0.155
13 0.030 0.027 0.027
14 -0.029 -0.023 -0.023
15 0.001 0.001 0.001
16 0.168 0.159 0.159
17 -0.055 -0.057 -0.057
18 -0.043 -0.044 -0.044
19 -0.660 -0.470 -0.470
20 1.133 0.810 0.810
21 0.754 0.576 0.576
22 0.396 0.315 0.315
23 0.420 0.269 0.269
24 -0.350 -0.310 -0.310
25 0.792 0.704 0.704
26 0.499 0.527 0.527
27 0.549 0.579 0.579
28 NA NA NA
29 NA NA NA
30 NA NA NA
31 NA NA NA
32 NA NA NA
33 NA NA NA
34 -0.002 -0.002 -0.002
35 0.007 0.007 0.007
36 0.007 0.007 0.007
37 0.000 0.000 0.000
38 0.058 0.050 0.050
39 -0.022 -0.020 -0.020
40 0.036 0.032 0.032
41 -0.091 -0.073 -0.073
42 0.061 0.058 0.058
43 -0.041 -0.037 -0.037
44 0.023 0.022 0.022
45 0.038 0.037 0.037
46 0.075 0.066 0.066
47 0.176 0.151 0.151
48 -0.197 -0.178 -0.178
49 -0.057 -0.051 -0.051
50 0.085 0.068 0.068
51 -0.040 -0.038 -0.038
52 -0.185 -0.166 -0.166
53 0.074 0.071 0.071
54 0.065 0.063 0.063
55 0.660 0.510 0.510
56 -1.133 -0.833 -0.833
57 -0.754 -0.615 -0.615
58 -0.396 -0.316 -0.316
59 0.420 0.269 0.269
60 0.350 0.311 0.311
61 -0.792 -0.638 -0.638
62 -0.499 -0.453 -0.453
63 -0.549 -0.505 -0.505
64 0.009 0.009 0.009
65 0.002 0.002 0.002
66 -0.013 -0.013 -0.013
67 1.000 1.000 1.000
68 -1.000 -1.000 -1.000
69 1.000 1.000 1.000
70 -0.002 -0.002 -0.002
71 -0.013 -0.013 -0.013
72 -0.007 -0.007 -0.007
16.10.7.6.4 Equivalence Test
The petersenlab
package (Petersen, 2024b) contains the equiv_chi()
function from Counsell et al. (2020) that performs an equivalence test: https://osf.io/cqu8v.
The chi-square equivalence test is non-significant, suggesting that the model fit is not acceptable.
The equivalence test of the chi-square difference test is non-significant, suggesting that the degree of worsening of model fit is not acceptable.
Code
Moreover, the equivalence test of the chi-square difference test is non-significant, suggesting that the degree of worsening of model fit is not acceptable. In other words, residual invariance failed.
Code
16.10.7.6.5 Permutation Test
Permutation procedures for testing measurement invariance are described in (Jorgensen et al., 2018).
For reproducibility, I set the seed below.
Using the same seed will yield the same answer every time.
There is nothing special about this particular seed.
You can specify the null model as the baseline model using: baseline.model = nullModelFit
.
Warning: this code takes a while to run based on \(100\) iterations. You can reduce the number of iterations to be faster.
Code
set.seed(52242)
residualInvarianceTest <- permuteMeasEq(
nPermute = numPermutations,
modelType = "mgcfa",
con = residualInvarianceModel_fit,
uncon = scalarInvarianceModel_fit,
AFIs = myAFIs,
moreAFIs = moreAFIs,
parallelType = "multicore", #only 'snow' works on Windows, but right now, it is throwing an error
iseed = 52242)
Omnibus p value based on parametric chi-squared difference test:
Chisq diff Df diff Pr(>Chisq)
14.356 9.000 0.110
Omnibus p values based on nonparametric permutation method:
AFI.Difference p.value
chisq 16.006 0.15
chisq.scaled 13.272 0.24
rmsea -0.004 0.38
cfi -0.008 0.15
tli 0.009 0.52
srmr 0.006 0.20
rmsea.robust -0.004 0.38
cfi.robust -0.007 0.15
tli.robust 0.010 0.50
The p-values are non-significant, indicating that the model did not fit significantly worse than the scalar invariance model.
16.10.7.7 Internal Consistency Reliability
Internal consistency reliability of items composing the latent factors, as quantified by omega (\(\omega\)) and average variance extracted (AVE), was estimated using the semTools
package (Jorgensen et al., 2021).
16.10.7.8 Path Diagram
A path diagram of the model generated using the semPlot
package (Epskamp, 2022) is below.
Code
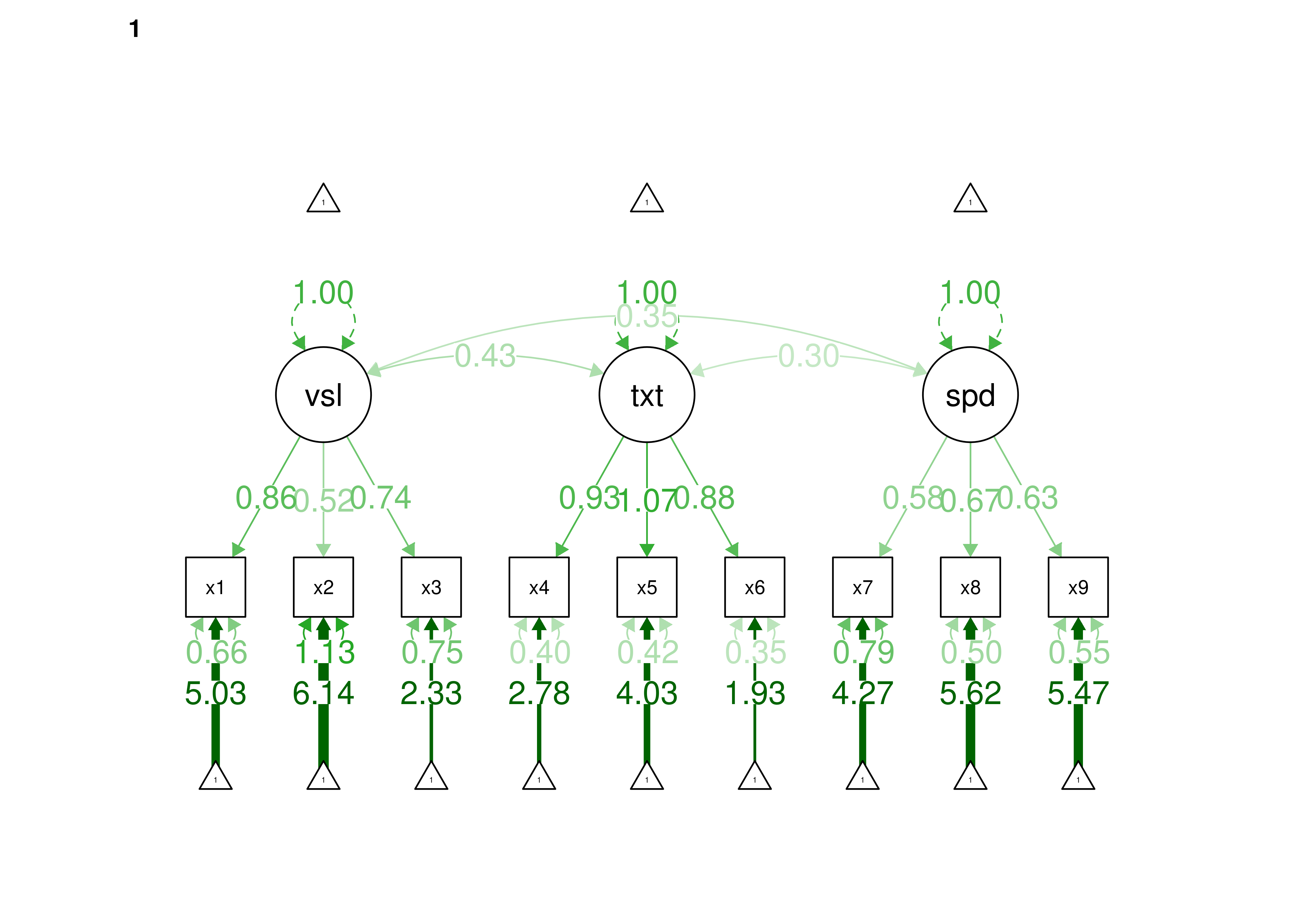
Figure 16.59: Residual Invariance Model in Confirmatory Factor Analysis.
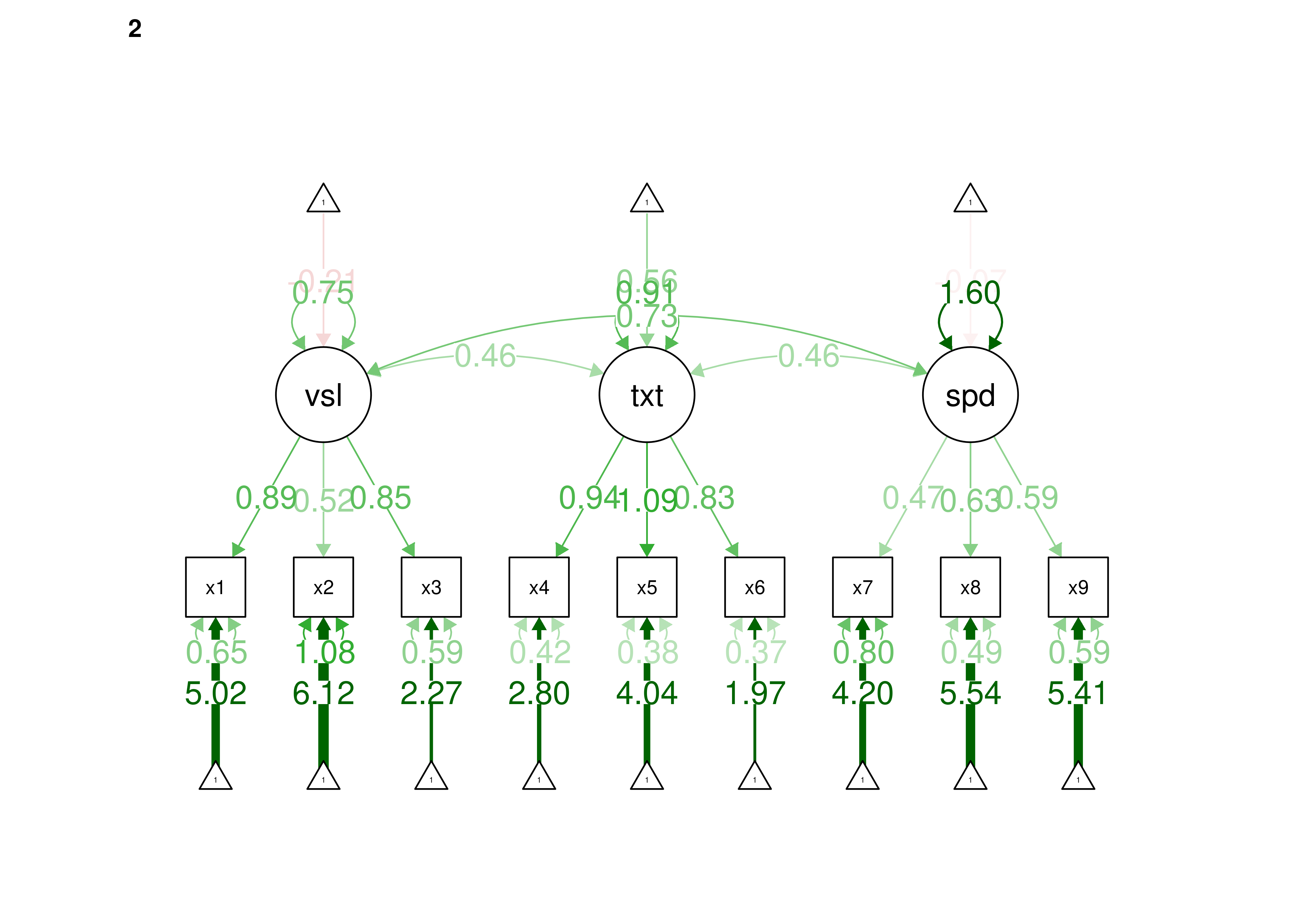
Figure 16.60: Residual Invariance Model in Confirmatory Factor Analysis.
16.10.8 Addressing Measurement Non-Invariance
In the example above, we detected measurement non-invariance of intercepts, but not factor loadings. Thus, we would want to identify which item(s) show non-invariant intercepts across groups. When detecting measurement non-invariance in a given parameter (e.g., factor loadings, intercepts, or residuals), one can identify the specific items that show measurement non-invariance in one of two primary ways: (1) starting with a model that allows the given parameter to differ across groups, a researcher can iteratively add constraints to identify the item(s) for which measurement invariance fails, or (2) starting with a model that constrains the given parameter to be the same across groups, a researcher can iteratively remove constraints to identify the item(s) for which measurement invariance becomes established (and by process of elimination, the items for which measurement invariance does not become established).
16.10.8.1 Iteratively add constraints to identify measurement non-invariance
In the example above, we detected measurement non-invariance of intercepts. One approach to identify measurement non-invariance is to iteratively add constraints to a model that allows the given parameter (intercepts) to differ across groups. So, we will use the metric invariance model as the baseline model (in which item intercepts are allowed to differ across groups), and iteratively add constraints to identify which item(s) show non-invariant intercepts across groups.
16.10.8.1.1 Variable 1
Variable 1 does not have non-invariant intercepts.
Code
cfaModel_metricInvarianceV1 <- '
#Fix factor loadings to be the same across groups
visual =~ c(lambdax1,lambdax1)*x1 + c(lambdax2,lambdax2)*x2 + c(lambdax3,lambdax3)*x3
textual =~ c(lambdax4,lambdax4)*x4 + c(lambdax5,lambdax5)*x5 + c(lambdax6,lambdax6)*x6
speed =~ c(lambdax7,lambdax7)*x7 + c(lambdax8,lambdax8)*x8 + c(lambdax9,lambdax9)*x9
#Fix latent means to zero
visual ~ 0
textual ~ 0
speed ~ 0
#Fix latent variances to one in group 1; free latent variances in group 2
visual ~~ c(1, NA)*visual
textual ~~ c(1, NA)*textual
speed ~~ c(1, NA)*speed
#Estimate covariances among latent variables
visual ~~ textual
visual ~~ speed
textual ~~ speed
#Estimate residual variances of manifest variables
x1 ~~ x1
x2 ~~ x2
x3 ~~ x3
x4 ~~ x4
x5 ~~ x5
x6 ~~ x6
x7 ~~ x7
x8 ~~ x8
x9 ~~ x9
#Iteratively fix intercepts of manifest variables across groups
x1 ~ c(intx1, intx1)*1
x2 ~ NA*1
x3 ~ NA*1
x4 ~ NA*1
x5 ~ NA*1
x6 ~ NA*1
x7 ~ NA*1
x8 ~ NA*1
x9 ~ NA*1
'
cfaModel_metricInvarianceV1_fit <- lavaan(
cfaModel_metricInvarianceV1,
data = HolzingerSwineford1939,
group = "school",
missing = "ML",
estimator = "MLR")
anova(metricInvarianceModel_fit, cfaModel_metricInvarianceV1_fit)
16.10.8.1.2 Variable 2
Variable 2 has non-invariant intercepts.
Code
cfaModel_metricInvarianceV2 <- '
#Fix factor loadings to be the same across groups
visual =~ c(lambdax1,lambdax1)*x1 + c(lambdax2,lambdax2)*x2 + c(lambdax3,lambdax3)*x3
textual =~ c(lambdax4,lambdax4)*x4 + c(lambdax5,lambdax5)*x5 + c(lambdax6,lambdax6)*x6
speed =~ c(lambdax7,lambdax7)*x7 + c(lambdax8,lambdax8)*x8 + c(lambdax9,lambdax9)*x9
#Fix latent means to zero
visual ~ 0
textual ~ 0
speed ~ 0
#Fix latent variances to one in group 1; free latent variances in group 2
visual ~~ c(1, NA)*visual
textual ~~ c(1, NA)*textual
speed ~~ c(1, NA)*speed
#Estimate covariances among latent variables
visual ~~ textual
visual ~~ speed
textual ~~ speed
#Estimate residual variances of manifest variables
x1 ~~ x1
x2 ~~ x2
x3 ~~ x3
x4 ~~ x4
x5 ~~ x5
x6 ~~ x6
x7 ~~ x7
x8 ~~ x8
x9 ~~ x9
#Iteratively fix intercepts of manifest variables across groups
x1 ~ c(intx1, intx1)*1
x2 ~ c(intx1, intx1)*1
x3 ~ NA*1
x4 ~ NA*1
x5 ~ NA*1
x6 ~ NA*1
x7 ~ NA*1
x8 ~ NA*1
x9 ~ NA*1
'
cfaModel_metricInvarianceV2_fit <- lavaan(
cfaModel_metricInvarianceV2,
data = HolzingerSwineford1939,
group = "school",
missing = "ML",
estimator = "MLR")
anova(metricInvarianceModel_fit, cfaModel_metricInvarianceV2_fit)
Variable 2 appears to have larger intercepts in group 2 than in group 1:
lavaan 0.6-19 ended normally after 73 iterations
Estimator ML
Optimization method NLMINB
Number of model parameters 63
Number of equality constraints 9
Number of observations per group:
Pasteur 156
Grant-White 145
Number of missing patterns per group:
Pasteur 9
Grant-White 9
Model Test User Model:
Standard Scaled
Test Statistic 116.313 117.955
Degrees of freedom 54 54
P-value (Chi-square) 0.000 0.000
Scaling correction factor 0.986
Yuan-Bentler correction (Mplus variant)
Test statistic for each group:
Pasteur 64.366 64.366
Grant-White 53.590 53.590
Parameter Estimates:
Standard errors Sandwich
Information bread Observed
Observed information based on Hessian
Group 1 [Pasteur]:
Latent Variables:
Estimate Std.Err z-value P(>|z|)
visual =~
x1 (l.1_) 0.885 0.122 7.258 0.000
x2 (l.2_) 0.536 0.088 6.106 0.000
x3 (l.3_) 0.707 0.087 8.148 0.000
textual =~
x4 (l.4_) 0.935 0.072 12.929 0.000
x5 (l.5_) 1.045 0.076 13.740 0.000
x6 (l.6_) 0.874 0.070 12.496 0.000
speed =~
x7 (l.7_) 0.550 0.075 7.346 0.000
x8 (l.8_) 0.675 0.087 7.784 0.000
x9 (l.9_) 0.602 0.088 6.867 0.000
Covariances:
Estimate Std.Err z-value P(>|z|)
visual ~~
textul (p.2_) 0.473 0.105 4.484 0.000
speed (p.3_1) 0.347 0.125 2.778 0.005
textual ~~
speed (p.3_2) 0.320 0.096 3.342 0.001
Intercepts:
Estimate Std.Err z-value P(>|z|)
.x1 (n.1.) 4.948 0.095 52.314 0.000
.x2 (n.2.) 5.984 0.098 60.949 0.000
.x3 (n.3.) 2.510 0.093 26.891 0.000
.x4 (n.4.) 2.814 0.091 30.886 0.000
.x5 (n.5.) 4.002 0.105 38.265 0.000
.x6 (n.6.) 1.929 0.079 24.377 0.000
.x7 (n.7.) 4.439 0.087 51.128 0.000
.x8 (n.8.) 5.564 0.079 70.170 0.000
.x9 (n.9.) 5.426 0.080 67.539 0.000
visual (a.1.) 0.000
textual (a.2.) 0.000
speed (a.3.) 0.000
Variances:
Estimate Std.Err z-value P(>|z|)
.x1 (t.1_) 0.577 0.166 3.482 0.000
.x2 (t.2_) 1.252 0.162 7.718 0.000
.x3 (t.3_) 0.853 0.127 6.737 0.000
.x4 (t.4_) 0.447 0.072 6.189 0.000
.x5 (t.5_) 0.486 0.075 6.445 0.000
.x6 (t.6_) 0.258 0.057 4.499 0.000
.x7 (t.7_) 0.860 0.114 7.549 0.000
.x8 (t.8_) 0.512 0.097 5.296 0.000
.x9 (t.9_) 0.635 0.118 5.376 0.000
visual (p.1_) 1.000
textual (p.2_) 1.000
speed (p.3_) 1.000
Group 2 [Grant-White]:
Latent Variables:
Estimate Std.Err z-value P(>|z|)
visual =~
x1 (l.1_) 0.885 0.122 7.258 0.000
x2 (l.2_) 0.536 0.088 6.106 0.000
x3 (l.3_) 0.707 0.087 8.148 0.000
textual =~
x4 (l.4_) 0.935 0.072 12.929 0.000
x5 (l.5_) 1.045 0.076 13.740 0.000
x6 (l.6_) 0.874 0.070 12.496 0.000
speed =~
x7 (l.7_) 0.550 0.075 7.346 0.000
x8 (l.8_) 0.675 0.087 7.784 0.000
x9 (l.9_) 0.602 0.088 6.867 0.000
Covariances:
Estimate Std.Err z-value P(>|z|)
visual ~~
textul (p.2_) 0.520 0.134 3.867 0.000
speed (p.3_1) 0.638 0.211 3.023 0.002
textual ~~
speed (p.3_2) 0.429 0.193 2.219 0.026
Intercepts:
Estimate Std.Err z-value P(>|z|)
.x1 (n.1.) 4.930 0.096 51.453 0.000
.x2 (n.2.) 6.198 0.092 67.063 0.000
.x3 (n.3.) 1.992 0.086 23.042 0.000
.x4 (n.4.) 3.318 0.093 35.501 0.000
.x5 (n.5.) 4.714 0.097 48.693 0.000
.x6 (n.6.) 2.467 0.094 26.165 0.000
.x7 (n.7.) 3.923 0.087 45.299 0.000
.x8 (n.8.) 5.488 0.087 63.174 0.000
.x9 (n.9.) 5.338 0.085 62.628 0.000
visual (a.1.) 0.000
textual (a.2.) 0.000
speed (a.3.) 0.000
Variances:
Estimate Std.Err z-value P(>|z|)
.x1 (t.1_) 0.652 0.168 3.877 0.000
.x2 (t.2_) 0.940 0.145 6.473 0.000
.x3 (t.3_) 0.606 0.113 5.376 0.000
.x4 (t.4_) 0.348 0.066 5.257 0.000
.x5 (t.5_) 0.371 0.074 5.021 0.000
.x6 (t.6_) 0.439 0.076 5.738 0.000
.x7 (t.7_) 0.622 0.098 6.338 0.000
.x8 (t.8_) 0.410 0.148 2.765 0.006
.x9 (t.9_) 0.505 0.128 3.957 0.000
visual (p.1_) 0.910 0.234 3.895 0.000
textual (p.2_) 1.004 0.191 5.257 0.000
speed (p.3_) 1.498 0.357 4.201 0.000
Thus, in subsequent models, we would allow variable 2 to have different intercepts across groups.
16.10.8.1.3 Variable 3
Variable 3 has non-invariant intercepts.
Code
cfaModel_metricInvarianceV3 <- '
#Fix factor loadings to be the same across groups
visual =~ c(lambdax1,lambdax1)*x1 + c(lambdax2,lambdax2)*x2 + c(lambdax3,lambdax3)*x3
textual =~ c(lambdax4,lambdax4)*x4 + c(lambdax5,lambdax5)*x5 + c(lambdax6,lambdax6)*x6
speed =~ c(lambdax7,lambdax7)*x7 + c(lambdax8,lambdax8)*x8 + c(lambdax9,lambdax9)*x9
#Fix latent means to zero
visual ~ 0
textual ~ 0
speed ~ 0
#Fix latent variances to one in group 1; free latent variances in group 2
visual ~~ c(1, NA)*visual
textual ~~ c(1, NA)*textual
speed ~~ c(1, NA)*speed
#Estimate covariances among latent variables
visual ~~ textual
visual ~~ speed
textual ~~ speed
#Estimate residual variances of manifest variables
x1 ~~ x1
x2 ~~ x2
x3 ~~ x3
x4 ~~ x4
x5 ~~ x5
x6 ~~ x6
x7 ~~ x7
x8 ~~ x8
x9 ~~ x9
#Iteratively fix intercepts of manifest variables across groups
x1 ~ c(intx1, intx1)*1
x2 ~ NA*1
x3 ~ c(intx1, intx1)*1
x4 ~ NA*1
x5 ~ NA*1
x6 ~ NA*1
x7 ~ NA*1
x8 ~ NA*1
x9 ~ NA*1
'
cfaModel_metricInvarianceV3_fit <- lavaan(
cfaModel_metricInvarianceV3,
data = HolzingerSwineford1939,
group = "school",
missing = "ML",
estimator = "MLR")
anova(metricInvarianceModel_fit, cfaModel_metricInvarianceV3_fit)
16.10.8.1.4 Variable 4
Variable 4 has non-invariant intercepts.
Code
cfaModel_metricInvarianceV4 <- '
#Fix factor loadings to be the same across groups
visual =~ c(lambdax1,lambdax1)*x1 + c(lambdax2,lambdax2)*x2 + c(lambdax3,lambdax3)*x3
textual =~ c(lambdax4,lambdax4)*x4 + c(lambdax5,lambdax5)*x5 + c(lambdax6,lambdax6)*x6
speed =~ c(lambdax7,lambdax7)*x7 + c(lambdax8,lambdax8)*x8 + c(lambdax9,lambdax9)*x9
#Fix latent means to zero
visual ~ 0
textual ~ 0
speed ~ 0
#Fix latent variances to one in group 1; free latent variances in group 2
visual ~~ c(1, NA)*visual
textual ~~ c(1, NA)*textual
speed ~~ c(1, NA)*speed
#Estimate covariances among latent variables
visual ~~ textual
visual ~~ speed
textual ~~ speed
#Estimate residual variances of manifest variables
x1 ~~ x1
x2 ~~ x2
x3 ~~ x3
x4 ~~ x4
x5 ~~ x5
x6 ~~ x6
x7 ~~ x7
x8 ~~ x8
x9 ~~ x9
#Iteratively fix intercepts of manifest variables across groups
x1 ~ c(intx1, intx1)*1
x2 ~ NA*1
x3 ~ NA*1
x4 ~ c(intx1, intx1)*1
x5 ~ NA*1
x6 ~ NA*1
x7 ~ NA*1
x8 ~ NA*1
x9 ~ NA*1
'
cfaModel_metricInvarianceV4_fit <- lavaan(
cfaModel_metricInvarianceV4,
data = HolzingerSwineford1939,
group = "school",
missing = "ML",
estimator = "MLR")
anova(metricInvarianceModel_fit, cfaModel_metricInvarianceV4_fit)
16.10.8.1.5 Variable 5
Variable 5 has non-invariant intercepts.
Code
cfaModel_metricInvarianceV5 <- '
#Fix factor loadings to be the same across groups
visual =~ c(lambdax1,lambdax1)*x1 + c(lambdax2,lambdax2)*x2 + c(lambdax3,lambdax3)*x3
textual =~ c(lambdax4,lambdax4)*x4 + c(lambdax5,lambdax5)*x5 + c(lambdax6,lambdax6)*x6
speed =~ c(lambdax7,lambdax7)*x7 + c(lambdax8,lambdax8)*x8 + c(lambdax9,lambdax9)*x9
#Fix latent means to zero
visual ~ 0
textual ~ 0
speed ~ 0
#Fix latent variances to one in group 1; free latent variances in group 2
visual ~~ c(1, NA)*visual
textual ~~ c(1, NA)*textual
speed ~~ c(1, NA)*speed
#Estimate covariances among latent variables
visual ~~ textual
visual ~~ speed
textual ~~ speed
#Estimate residual variances of manifest variables
x1 ~~ x1
x2 ~~ x2
x3 ~~ x3
x4 ~~ x4
x5 ~~ x5
x6 ~~ x6
x7 ~~ x7
x8 ~~ x8
x9 ~~ x9
#Iteratively fix intercepts of manifest variables across groups
x1 ~ c(intx1, intx1)*1
x2 ~ NA*1
x3 ~ NA*1
x4 ~ NA*1
x5 ~ c(intx1, intx1)*1
x6 ~ NA*1
x7 ~ NA*1
x8 ~ NA*1
x9 ~ NA*1
'
cfaModel_metricInvarianceV5_fit <- lavaan(
cfaModel_metricInvarianceV5,
data = HolzingerSwineford1939,
group = "school",
missing = "ML",
estimator = "MLR")
anova(metricInvarianceModel_fit, cfaModel_metricInvarianceV5_fit)
16.10.8.1.6 Variable 6
Variable 6 has non-invariant intercepts.
Code
cfaModel_metricInvarianceV6 <- '
#Fix factor loadings to be the same across groups
visual =~ c(lambdax1,lambdax1)*x1 + c(lambdax2,lambdax2)*x2 + c(lambdax3,lambdax3)*x3
textual =~ c(lambdax4,lambdax4)*x4 + c(lambdax5,lambdax5)*x5 + c(lambdax6,lambdax6)*x6
speed =~ c(lambdax7,lambdax7)*x7 + c(lambdax8,lambdax8)*x8 + c(lambdax9,lambdax9)*x9
#Fix latent means to zero
visual ~ 0
textual ~ 0
speed ~ 0
#Fix latent variances to one in group 1; free latent variances in group 2
visual ~~ c(1, NA)*visual
textual ~~ c(1, NA)*textual
speed ~~ c(1, NA)*speed
#Estimate covariances among latent variables
visual ~~ textual
visual ~~ speed
textual ~~ speed
#Estimate residual variances of manifest variables
x1 ~~ x1
x2 ~~ x2
x3 ~~ x3
x4 ~~ x4
x5 ~~ x5
x6 ~~ x6
x7 ~~ x7
x8 ~~ x8
x9 ~~ x9
#Iteratively fix intercepts of manifest variables across groups
x1 ~ c(intx1, intx1)*1
x2 ~ NA*1
x3 ~ NA*1
x4 ~ NA*1
x5 ~ NA*1
x6 ~ c(intx1, intx1)*1
x7 ~ NA*1
x8 ~ NA*1
x9 ~ NA*1
'
cfaModel_metricInvarianceV6_fit <- lavaan(
cfaModel_metricInvarianceV6,
data = HolzingerSwineford1939,
group = "school",
missing = "ML",
estimator = "MLR")
anova(metricInvarianceModel_fit, cfaModel_metricInvarianceV6_fit)
16.10.8.1.7 Variable 7
Variable 7 has non-invariant intercepts.
Code
cfaModel_metricInvarianceV7 <- '
#Fix factor loadings to be the same across groups
visual =~ c(lambdax1,lambdax1)*x1 + c(lambdax2,lambdax2)*x2 + c(lambdax3,lambdax3)*x3
textual =~ c(lambdax4,lambdax4)*x4 + c(lambdax5,lambdax5)*x5 + c(lambdax6,lambdax6)*x6
speed =~ c(lambdax7,lambdax7)*x7 + c(lambdax8,lambdax8)*x8 + c(lambdax9,lambdax9)*x9
#Fix latent means to zero
visual ~ 0
textual ~ 0
speed ~ 0
#Fix latent variances to one in group 1; free latent variances in group 2
visual ~~ c(1, NA)*visual
textual ~~ c(1, NA)*textual
speed ~~ c(1, NA)*speed
#Estimate covariances among latent variables
visual ~~ textual
visual ~~ speed
textual ~~ speed
#Estimate residual variances of manifest variables
x1 ~~ x1
x2 ~~ x2
x3 ~~ x3
x4 ~~ x4
x5 ~~ x5
x6 ~~ x6
x7 ~~ x7
x8 ~~ x8
x9 ~~ x9
#Iteratively fix intercepts of manifest variables across groups
x1 ~ c(intx1, intx1)*1
x2 ~ NA*1
x3 ~ NA*1
x4 ~ NA*1
x5 ~ NA*1
x6 ~ NA*1
x7 ~ c(intx1, intx1)*1
x8 ~ NA*1
x9 ~ NA*1
'
cfaModel_metricInvarianceV7_fit <- lavaan(
cfaModel_metricInvarianceV7,
data = HolzingerSwineford1939,
group = "school",
missing = "ML",
estimator = "MLR")
anova(metricInvarianceModel_fit, cfaModel_metricInvarianceV7_fit)
16.10.8.1.8 Variable 8
Variable 8 has non-invariant intercepts.
Code
cfaModel_metricInvarianceV8 <- '
#Fix factor loadings to be the same across groups
visual =~ c(lambdax1,lambdax1)*x1 + c(lambdax2,lambdax2)*x2 + c(lambdax3,lambdax3)*x3
textual =~ c(lambdax4,lambdax4)*x4 + c(lambdax5,lambdax5)*x5 + c(lambdax6,lambdax6)*x6
speed =~ c(lambdax7,lambdax7)*x7 + c(lambdax8,lambdax8)*x8 + c(lambdax9,lambdax9)*x9
#Fix latent means to zero
visual ~ 0
textual ~ 0
speed ~ 0
#Fix latent variances to one in group 1; free latent variances in group 2
visual ~~ c(1, NA)*visual
textual ~~ c(1, NA)*textual
speed ~~ c(1, NA)*speed
#Estimate covariances among latent variables
visual ~~ textual
visual ~~ speed
textual ~~ speed
#Estimate residual variances of manifest variables
x1 ~~ x1
x2 ~~ x2
x3 ~~ x3
x4 ~~ x4
x5 ~~ x5
x6 ~~ x6
x7 ~~ x7
x8 ~~ x8
x9 ~~ x9
#Iteratively fix intercepts of manifest variables across groups
x1 ~ c(intx1, intx1)*1
x2 ~ NA*1
x3 ~ NA*1
x4 ~ NA*1
x5 ~ NA*1
x6 ~ NA*1
x7 ~ NA*1
x8 ~ c(intx1, intx1)*1
x9 ~ NA*1
'
cfaModel_metricInvarianceV8_fit <- lavaan(
cfaModel_metricInvarianceV8,
data = HolzingerSwineford1939,
group = "school",
missing = "ML",
estimator = "MLR")
anova(metricInvarianceModel_fit, cfaModel_metricInvarianceV8_fit)
16.10.8.1.9 Variable 9
Variable 9 has non-invariant intercepts.
Code
cfaModel_metricInvarianceV9 <- '
#Fix factor loadings to be the same across groups
visual =~ c(lambdax1,lambdax1)*x1 + c(lambdax2,lambdax2)*x2 + c(lambdax3,lambdax3)*x3
textual =~ c(lambdax4,lambdax4)*x4 + c(lambdax5,lambdax5)*x5 + c(lambdax6,lambdax6)*x6
speed =~ c(lambdax7,lambdax7)*x7 + c(lambdax8,lambdax8)*x8 + c(lambdax9,lambdax9)*x9
#Fix latent means to zero
visual ~ 0
textual ~ 0
speed ~ 0
#Fix latent variances to one in group 1; free latent variances in group 2
visual ~~ c(1, NA)*visual
textual ~~ c(1, NA)*textual
speed ~~ c(1, NA)*speed
#Estimate covariances among latent variables
visual ~~ textual
visual ~~ speed
textual ~~ speed
#Estimate residual variances of manifest variables
x1 ~~ x1
x2 ~~ x2
x3 ~~ x3
x4 ~~ x4
x5 ~~ x5
x6 ~~ x6
x7 ~~ x7
x8 ~~ x8
x9 ~~ x9
#Iteratively fix intercepts of manifest variables across groups
x1 ~ c(intx1, intx1)*1
x2 ~ NA*1
x3 ~ NA*1
x4 ~ NA*1
x5 ~ NA*1
x6 ~ NA*1
x7 ~ NA*1
x8 ~ NA*1
x9 ~ c(intx1, intx1)*1
'
cfaModel_metricInvarianceV9_fit <- lavaan(
cfaModel_metricInvarianceV9,
data = HolzingerSwineford1939,
group = "school",
missing = "ML",
estimator = "MLR")
anova(metricInvarianceModel_fit, cfaModel_metricInvarianceV9_fit)
16.10.8.1.10 Summary
When iteratively adding constraints, item 1 showed measurement invariance of intercepts, but all other items showed measurement non-invariance of intercepts. This could be due to the selection of starting with item 1 as the first constraint. We could try applying constraints in a different order to see the extent to which the order influences which items are identified as showing measurement non-invariance.
16.10.8.2 Iteratively drop constraints to identify measurement non-invariance
Another approach to identify measurement non-invariance is to iteratively drop constraints to a model that constrains the given parameter (intercepts) to be the same across groups. So, we will use the scalar invariance model as the baseline model (in which item intercepts are constrained to be the same across groups), and we will iteratively drop constraints to identify the items for which measurement invariance becomes established (relative to the metric invariance model which allows item intercepts to differ across groups), and therefore, identifies which item(s) show non-invariant intercepts across groups.
16.10.8.2.1 Variable 1
Measurement invariance does not yet become established when freeing intercepts of variable 1 across groups. This suggests that, whether or not variable 1 shows non-invariant intercepts, there are other items that show non-invariant intercepts.
Code
cfaModel_scalarInvarianceV1 <- '
#Fix factor loadings to be the same across groups
visual =~ c(lambdax1,lambdax1)*x1 + c(lambdax2,lambdax2)*x2 + c(lambdax3,lambdax3)*x3
textual =~ c(lambdax4,lambdax4)*x4 + c(lambdax5,lambdax5)*x5 + c(lambdax6,lambdax6)*x6
speed =~ c(lambdax7,lambdax7)*x7 + c(lambdax8,lambdax8)*x8 + c(lambdax9,lambdax9)*x9
#Fix latent means to zero
visual ~ 0
textual ~ 0
speed ~ 0
#Fix latent variances to one in group 1; free latent variances in group 2
visual ~~ c(1, NA)*visual
textual ~~ c(1, NA)*textual
speed ~~ c(1, NA)*speed
#Estimate covariances among latent variables
visual ~~ textual
visual ~~ speed
textual ~~ speed
#Estimate residual variances of manifest variables
x1 ~~ x1
x2 ~~ x2
x3 ~~ x3
x4 ~~ x4
x5 ~~ x5
x6 ~~ x6
x7 ~~ x7
x8 ~~ x8
x9 ~~ x9
#Iteratively free intercepts across groups
x1 ~ NA*1
x2 ~ c(intx2, intx2)*1
x3 ~ c(intx3, intx3)*1
x4 ~ c(intx4, intx4)*1
x5 ~ c(intx5, intx5)*1
x6 ~ c(intx6, intx6)*1
x7 ~ c(intx7, intx7)*1
x8 ~ c(intx8, intx8)*1
x9 ~ c(intx9, intx9)*1
'
cfaModel_scalarInvarianceV1_fit <- lavaan(
cfaModel_scalarInvarianceV1,
data = HolzingerSwineford1939,
group = "school",
missing = "ML",
estimator = "MLR")
anova(metricInvarianceModel_fit, cfaModel_scalarInvarianceV1_fit)
16.10.8.2.2 Variable 2
Measurement invariance does not yet become established when freeing intercepts of variable 2 across groups. This suggests that, whether or not variables 1 and 2 show non-invariant intercepts, there are other items that show non-invariant intercepts.
Code
cfaModel_scalarInvarianceV2 <- '
#Fix factor loadings to be the same across groups
visual =~ c(lambdax1,lambdax1)*x1 + c(lambdax2,lambdax2)*x2 + c(lambdax3,lambdax3)*x3
textual =~ c(lambdax4,lambdax4)*x4 + c(lambdax5,lambdax5)*x5 + c(lambdax6,lambdax6)*x6
speed =~ c(lambdax7,lambdax7)*x7 + c(lambdax8,lambdax8)*x8 + c(lambdax9,lambdax9)*x9
#Fix latent means to zero
visual ~ 0
textual ~ 0
speed ~ 0
#Fix latent variances to one in group 1; free latent variances in group 2
visual ~~ c(1, NA)*visual
textual ~~ c(1, NA)*textual
speed ~~ c(1, NA)*speed
#Estimate covariances among latent variables
visual ~~ textual
visual ~~ speed
textual ~~ speed
#Estimate residual variances of manifest variables
x1 ~~ x1
x2 ~~ x2
x3 ~~ x3
x4 ~~ x4
x5 ~~ x5
x6 ~~ x6
x7 ~~ x7
x8 ~~ x8
x9 ~~ x9
#Iteratively free intercepts across groups
x1 ~ NA*1
x2 ~ NA*1
x3 ~ c(intx3, intx3)*1
x4 ~ c(intx4, intx4)*1
x5 ~ c(intx5, intx5)*1
x6 ~ c(intx6, intx6)*1
x7 ~ c(intx7, intx7)*1
x8 ~ c(intx8, intx8)*1
x9 ~ c(intx9, intx9)*1
'
cfaModel_scalarInvarianceV2_fit <- lavaan(
cfaModel_scalarInvarianceV2,
data = HolzingerSwineford1939,
group = "school",
missing = "ML",
estimator = "MLR")
anova(metricInvarianceModel_fit, cfaModel_scalarInvarianceV2_fit)
16.10.8.2.3 Variable 3
Measurement invariance does not yet become established when freeing intercepts of variable 3 across groups. This suggests that, whether or not variables 1, 2, and 3 show non-invariant intercepts, there are other items that show non-invariant intercepts.
Code
cfaModel_scalarInvarianceV3 <- '
#Fix factor loadings to be the same across groups
visual =~ c(lambdax1,lambdax1)*x1 + c(lambdax2,lambdax2)*x2 + c(lambdax3,lambdax3)*x3
textual =~ c(lambdax4,lambdax4)*x4 + c(lambdax5,lambdax5)*x5 + c(lambdax6,lambdax6)*x6
speed =~ c(lambdax7,lambdax7)*x7 + c(lambdax8,lambdax8)*x8 + c(lambdax9,lambdax9)*x9
#Fix latent means to zero
visual ~ 0
textual ~ 0
speed ~ 0
#Fix latent variances to one in group 1; free latent variances in group 2
visual ~~ c(1, NA)*visual
textual ~~ c(1, NA)*textual
speed ~~ c(1, NA)*speed
#Estimate covariances among latent variables
visual ~~ textual
visual ~~ speed
textual ~~ speed
#Estimate residual variances of manifest variables
x1 ~~ x1
x2 ~~ x2
x3 ~~ x3
x4 ~~ x4
x5 ~~ x5
x6 ~~ x6
x7 ~~ x7
x8 ~~ x8
x9 ~~ x9
#Iteratively free intercepts across groups
x1 ~ NA*1
x2 ~ NA*1
x3 ~ NA*1
x4 ~ c(intx4, intx4)*1
x5 ~ c(intx5, intx5)*1
x6 ~ c(intx6, intx6)*1
x7 ~ c(intx7, intx7)*1
x8 ~ c(intx8, intx8)*1
x9 ~ c(intx9, intx9)*1
'
cfaModel_scalarInvarianceV3_fit <- lavaan(
cfaModel_scalarInvarianceV3,
data = HolzingerSwineford1939,
group = "school",
missing = "ML",
estimator = "MLR")
anova(metricInvarianceModel_fit, cfaModel_scalarInvarianceV3_fit)
16.10.8.2.4 Variable 4
Measurement invariance does not yet become established when freeing intercepts of variable 4 across groups. This suggests that, whether or not variables 1, 2, 3, and 4 show non-invariant intercepts, there are other items that show non-invariant intercepts.
Code
cfaModel_scalarInvarianceV4 <- '
#Fix factor loadings to be the same across groups
visual =~ c(lambdax1,lambdax1)*x1 + c(lambdax2,lambdax2)*x2 + c(lambdax3,lambdax3)*x3
textual =~ c(lambdax4,lambdax4)*x4 + c(lambdax5,lambdax5)*x5 + c(lambdax6,lambdax6)*x6
speed =~ c(lambdax7,lambdax7)*x7 + c(lambdax8,lambdax8)*x8 + c(lambdax9,lambdax9)*x9
#Fix latent means to zero
visual ~ 0
textual ~ 0
speed ~ 0
#Fix latent variances to one in group 1; free latent variances in group 2
visual ~~ c(1, NA)*visual
textual ~~ c(1, NA)*textual
speed ~~ c(1, NA)*speed
#Estimate covariances among latent variables
visual ~~ textual
visual ~~ speed
textual ~~ speed
#Estimate residual variances of manifest variables
x1 ~~ x1
x2 ~~ x2
x3 ~~ x3
x4 ~~ x4
x5 ~~ x5
x6 ~~ x6
x7 ~~ x7
x8 ~~ x8
x9 ~~ x9
#Iteratively free intercepts across groups
x1 ~ NA*1
x2 ~ NA*1
x3 ~ NA*1
x4 ~ NA*1
x5 ~ c(intx5, intx5)*1
x6 ~ c(intx6, intx6)*1
x7 ~ c(intx7, intx7)*1
x8 ~ c(intx8, intx8)*1
x9 ~ c(intx9, intx9)*1
'
cfaModel_scalarInvarianceV4_fit <- lavaan(
cfaModel_scalarInvarianceV4,
data = HolzingerSwineford1939,
group = "school",
missing = "ML",
estimator = "MLR")
anova(metricInvarianceModel_fit, cfaModel_scalarInvarianceV4_fit)
16.10.8.2.5 Variable 5
Measurement invariance does not yet become established when freeing intercepts of variable 5 across groups. This suggests that, whether or not variables 1, 2, 3, 4, and 5 show non-invariant intercepts, there are other items that show non-invariant intercepts.
Code
cfaModel_scalarInvarianceV5 <- '
#Fix factor loadings to be the same across groups
visual =~ c(lambdax1,lambdax1)*x1 + c(lambdax2,lambdax2)*x2 + c(lambdax3,lambdax3)*x3
textual =~ c(lambdax4,lambdax4)*x4 + c(lambdax5,lambdax5)*x5 + c(lambdax6,lambdax6)*x6
speed =~ c(lambdax7,lambdax7)*x7 + c(lambdax8,lambdax8)*x8 + c(lambdax9,lambdax9)*x9
#Fix latent means to zero
visual ~ 0
textual ~ 0
speed ~ 0
#Fix latent variances to one in group 1; free latent variances in group 2
visual ~~ c(1, NA)*visual
textual ~~ c(1, NA)*textual
speed ~~ c(1, NA)*speed
#Estimate covariances among latent variables
visual ~~ textual
visual ~~ speed
textual ~~ speed
#Estimate residual variances of manifest variables
x1 ~~ x1
x2 ~~ x2
x3 ~~ x3
x4 ~~ x4
x5 ~~ x5
x6 ~~ x6
x7 ~~ x7
x8 ~~ x8
x9 ~~ x9
#Iteratively free intercepts across groups
x1 ~ NA*1
x2 ~ NA*1
x3 ~ NA*1
x4 ~ NA*1
x5 ~ NA*1
x6 ~ c(intx6, intx6)*1
x7 ~ c(intx7, intx7)*1
x8 ~ c(intx8, intx8)*1
x9 ~ c(intx9, intx9)*1
'
cfaModel_scalarInvarianceV5_fit <- lavaan(
cfaModel_scalarInvarianceV5,
data = HolzingerSwineford1939,
group = "school",
missing = "ML",
estimator = "MLR")
anova(metricInvarianceModel_fit, cfaModel_scalarInvarianceV5_fit)
16.10.8.2.6 Variable 6
Measurement invariance does not yet become established when freeing intercepts of variable 6 across groups. This suggests that, whether or not variables 1, 2, 3, 4, 5, and 6 show non-invariant intercepts, there are other items that show non-invariant intercepts.
Code
cfaModel_scalarInvarianceV6 <- '
#Fix factor loadings to be the same across groups
visual =~ c(lambdax1,lambdax1)*x1 + c(lambdax2,lambdax2)*x2 + c(lambdax3,lambdax3)*x3
textual =~ c(lambdax4,lambdax4)*x4 + c(lambdax5,lambdax5)*x5 + c(lambdax6,lambdax6)*x6
speed =~ c(lambdax7,lambdax7)*x7 + c(lambdax8,lambdax8)*x8 + c(lambdax9,lambdax9)*x9
#Fix latent means to zero
visual ~ 0
textual ~ 0
speed ~ 0
#Fix latent variances to one in group 1; free latent variances in group 2
visual ~~ c(1, NA)*visual
textual ~~ c(1, NA)*textual
speed ~~ c(1, NA)*speed
#Estimate covariances among latent variables
visual ~~ textual
visual ~~ speed
textual ~~ speed
#Estimate residual variances of manifest variables
x1 ~~ x1
x2 ~~ x2
x3 ~~ x3
x4 ~~ x4
x5 ~~ x5
x6 ~~ x6
x7 ~~ x7
x8 ~~ x8
x9 ~~ x9
#Iteratively free intercepts across groups
x1 ~ NA*1
x2 ~ NA*1
x3 ~ NA*1
x4 ~ NA*1
x5 ~ NA*1
x6 ~ NA*1
x7 ~ c(intx7, intx7)*1
x8 ~ c(intx8, intx8)*1
x9 ~ c(intx9, intx9)*1
'
cfaModel_scalarInvarianceV6_fit <- lavaan(
cfaModel_scalarInvarianceV6,
data = HolzingerSwineford1939,
group = "school",
missing = "ML",
estimator = "MLR")
anova(metricInvarianceModel_fit, cfaModel_scalarInvarianceV6_fit)
16.10.8.2.7 Variable 7
Measurement invariance becomes established when freeing intercepts of variable 7 across groups. This suggests that item 7 shows non-invariant intercepts across groups, and that measurement invariance holds when constraining items 8 and 9 to be invariant across groups. There may also be other items, especially among the preceding items (variables 1–6), that show non-invariant intercepts across groups.
Code
cfaModel_scalarInvarianceV7 <- '
#Fix factor loadings to be the same across groups
visual =~ c(lambdax1,lambdax1)*x1 + c(lambdax2,lambdax2)*x2 + c(lambdax3,lambdax3)*x3
textual =~ c(lambdax4,lambdax4)*x4 + c(lambdax5,lambdax5)*x5 + c(lambdax6,lambdax6)*x6
speed =~ c(lambdax7,lambdax7)*x7 + c(lambdax8,lambdax8)*x8 + c(lambdax9,lambdax9)*x9
#Fix latent means to zero
visual ~ 0
textual ~ 0
speed ~ 0
#Fix latent variances to one in group 1; free latent variances in group 2
visual ~~ c(1, NA)*visual
textual ~~ c(1, NA)*textual
speed ~~ c(1, NA)*speed
#Estimate covariances among latent variables
visual ~~ textual
visual ~~ speed
textual ~~ speed
#Estimate residual variances of manifest variables
x1 ~~ x1
x2 ~~ x2
x3 ~~ x3
x4 ~~ x4
x5 ~~ x5
x6 ~~ x6
x7 ~~ x7
x8 ~~ x8
x9 ~~ x9
#Iteratively free intercepts across groups
x1 ~ NA*1
x2 ~ NA*1
x3 ~ NA*1
x4 ~ NA*1
x5 ~ NA*1
x6 ~ NA*1
x7 ~ NA*1
x8 ~ c(intx8, intx8)*1
x9 ~ c(intx9, intx9)*1
'
cfaModel_scalarInvarianceV7_fit <- lavaan(
cfaModel_scalarInvarianceV7,
data = HolzingerSwineford1939,
group = "school",
missing = "ML",
estimator = "MLR")
anova(metricInvarianceModel_fit, cfaModel_scalarInvarianceV7_fit)
16.10.8.2.8 Variable 8
Measurement invariance continues to hold when freeing intercepts of variable 8 across groups. This suggests that variable 8 does not show non-invariant intercepts across groups, at least when the other intercepts have been freed.
Code
cfaModel_scalarInvarianceV8 <- '
#Fix factor loadings to be the same across groups
visual =~ c(lambdax1,lambdax1)*x1 + c(lambdax2,lambdax2)*x2 + c(lambdax3,lambdax3)*x3
textual =~ c(lambdax4,lambdax4)*x4 + c(lambdax5,lambdax5)*x5 + c(lambdax6,lambdax6)*x6
speed =~ c(lambdax7,lambdax7)*x7 + c(lambdax8,lambdax8)*x8 + c(lambdax9,lambdax9)*x9
#Fix latent means to zero
visual ~ 0
textual ~ 0
speed ~ 0
#Fix latent variances to one in group 1; free latent variances in group 2
visual ~~ c(1, NA)*visual
textual ~~ c(1, NA)*textual
speed ~~ c(1, NA)*speed
#Estimate covariances among latent variables
visual ~~ textual
visual ~~ speed
textual ~~ speed
#Estimate residual variances of manifest variables
x1 ~~ x1
x2 ~~ x2
x3 ~~ x3
x4 ~~ x4
x5 ~~ x5
x6 ~~ x6
x7 ~~ x7
x8 ~~ x8
x9 ~~ x9
#Iteratively free intercepts across groups
x1 ~ NA*1
x2 ~ NA*1
x3 ~ NA*1
x4 ~ NA*1
x5 ~ NA*1
x6 ~ NA*1
x7 ~ NA*1
x8 ~ NA*1
x9 ~ c(intx9, intx9)*1
'
cfaModel_scalarInvarianceV8_fit <- lavaan(
cfaModel_scalarInvarianceV8,
data = HolzingerSwineford1939,
group = "school",
missing = "ML",
estimator = "MLR")
anova(metricInvarianceModel_fit, cfaModel_scalarInvarianceV8_fit)
16.10.8.2.9 Variable 9
Measurement invariance continues to hold when freeing intercepts of variable 9 across groups (even after constraining intercepts of variable 8 across groups). This suggests that variable 9 does not show non-invariant intercepts across groups, at least when the other intercepts have been freed.
Code
cfaModel_scalarInvarianceV9 <- '
#Fix factor loadings to be the same across groups
visual =~ c(lambdax1,lambdax1)*x1 + c(lambdax2,lambdax2)*x2 + c(lambdax3,lambdax3)*x3
textual =~ c(lambdax4,lambdax4)*x4 + c(lambdax5,lambdax5)*x5 + c(lambdax6,lambdax6)*x6
speed =~ c(lambdax7,lambdax7)*x7 + c(lambdax8,lambdax8)*x8 + c(lambdax9,lambdax9)*x9
#Fix latent means to zero
visual ~ 0
textual ~ 0
speed ~ 0
#Fix latent variances to one in group 1; free latent variances in group 2
visual ~~ c(1, NA)*visual
textual ~~ c(1, NA)*textual
speed ~~ c(1, NA)*speed
#Estimate covariances among latent variables
visual ~~ textual
visual ~~ speed
textual ~~ speed
#Estimate residual variances of manifest variables
x1 ~~ x1
x2 ~~ x2
x3 ~~ x3
x4 ~~ x4
x5 ~~ x5
x6 ~~ x6
x7 ~~ x7
x8 ~~ x8
x9 ~~ x9
#Iteratively free intercepts across groups
x1 ~ NA*1
x2 ~ NA*1
x3 ~ NA*1
x4 ~ NA*1
x5 ~ NA*1
x6 ~ NA*1
x7 ~ NA*1
x8 ~ c(intx8, intx8)*1
x9 ~ NA*1
'
cfaModel_scalarInvarianceV9_fit <- lavaan(
cfaModel_scalarInvarianceV9,
data = HolzingerSwineford1939,
group = "school",
missing = "ML",
estimator = "MLR")
anova(metricInvarianceModel_fit, cfaModel_scalarInvarianceV9_fit)
16.10.8.2.10 Summary
When iteratively dropping constraints, measurement invariance became established when freeing the intercepts for items 1–7 across groups. This suggests that items 7, 8, and 9 show measurement invariance of intercepts across groups. This could be due to the selection of starting with item 1 as the first constraint to be freed. We could try freeing constraints in a different order to see the extent to which the order influences which items are identified as showing measurement non-invariance.
16.10.8.3 Next Steps
After identifying which item(s) show measurement non-invariance, we would evaluate the measurement non-invariance according to theory and effect sizes. We might start with the item with the largest measurement non-invariance. If we deem the measurement non-invariance for this item is non-negligible, we have three primary options: (1) drop the item for both groups, (2) drop the item for one group but keep it for the other group, or (3) freely estimate parameters for the item across groups. We would do this iteratively for the remaining items, by magnitude, that show non-negligible measurement non-invariance. Thus, our model might show partial scalar invariance—some items might have intercepts that are constrained to be the same across groups, whereas other items might have intercepts that are allowed to differ across groups. Then, we would test subsequent measurement invariance models (e.g., residual invariance) while keeping the partially freed constaints from the partial scalar invariance model. Once we establish the best fitting model that makes as many constraints that are theoretically and empirically justified for the purposes of the study, we would use that model in subsequent tests. As a reminder, full or partial metric invariance is helpful for comparing associations across groups, whereas full or partial scalar invariance is helpful for comparing mean levels across groups.
16.11 Conclusion
Bias is a systematic error. Test bias refers to a systematic error (in measurement, prediction, etc.) as a function of group membership. The two broad categories of test bias include predictive bias and test structure bias. Predictive bias refers to differences between groups in the relation between the test and criterion—i.e., different intercepts and/or slopes of the regression line between the test and the criterion. Test structure bias refers to differences in the internal test characteristics across groups, and can be evaluated with approaches such as measurement invariance and differential item function. When detecting test bias, it is important to address it, and there are a number of ways of correcting for bias, including score adjustment and other approaches that do not involve score adjustment. However, even if a test does not show bias, it does not mean that the test is fair. Fairness is not a scientific question but rather a moral, societal, and ethical question; there are many different ways of operationalizing fairness. Fairness is a complex question, so do the best you can and try to minimize any negative impact of the assessment procedures.
16.12 Suggested Readings
Putnick & Bornstein (2016); N. S. Cole (1981); Fernández & Abe (2018); Reynolds & Suzuki (2012); Sackett & Wilk (1994); Jonson & Geisinger (2022)
16.13 Exercises
16.13.1 Questions
Note: Several of the following questions use data from the Children of the National Longitudinal Survey of Youth Survey (CNLSY). The CNLSY is a publicly available longitudinal data set provided by the Bureau of Labor Statistics (https://www.bls.gov/nls/nlsy79-children.htm#topical-guide; archived at https://perma.cc/EH38-HDRN). The CNLSY data file for these exercises is located on the book’s page of the Open Science Framework (https://osf.io/3pwza). Children’s behavior problems were rated in 1988 (time 1: T1) and then again in 1990 (time 2: T2) on the Behavior Problems Index (BPI). Below are the items corresponding to the Antisocial subscale of the BPI:
- cheats or tells lies
- bullies or is cruel/mean to others
- does not seem to feel sorry after misbehaving
- breaks things deliberately
- is disobedient at school
- has trouble getting along with teachers
- has sudden changes in mood or feeling
- Determine whether there is predictive bias, as a function of sex, in predicting antisocial behavior at T2 using antisocial behavior at T2. Describe any predictive bias observed.
- How could you address this predictive bias?
- Assume that children are selected to receive preventative services if their score on the Antisocial scale at T1 is > 6. Assume that children at T2 have an antisocial disorder if their score at T2 is > 6. Does the Antisocial scale show fairness in terms of equal outcomes between boys and girls?
- A lawyer argues in court that a test that is used to select students for college admissions should be able to be used because it has been demonstrated to show no evidence of bias against particular groups. However, you know that just because a measure may be unbiased does not mean that using the measure’s scores for that purpose is fair. How could such a measure be unbiased yet unfair?
- Fit a multi-group item response theory model to the Antisocial subscale at time 2, with the participant’s sex as a grouping factor.
- Does a model with item parameters constrained across sexes fit significantly worse than a model with item parameters that are allowed to vary across sexes?
- Starting with an unconstrained model that allows item parameters to vary across sexes, sequentially add constraints to item parameters across groups. Which items differed in discrimination across groups? Which items differed in severity across groups? Describe any differences.
- How could any differential item functioning be addressed?
- Examine the longitudinal measurement invariance of the Antisocial scale across T1 and T2. Use full information maximum likelihood (FIML) to account for missing data. Use robust standard errors to account for non-normally distributed data. Which type(s) of longitudinal measurement invariance failed? Which items and parameters appeared to differ across ages?
16.13.2 Answers
- There is predictive bias in terms of different slopes across sexes. The slope is steeper for boys than girls, indicating that the measure is not as predictively accurate for girls as it is for boys.
- You could address this predictive bias by using a different predictive measure for girls than boys, changing the criterion, or by using within-group norming.
- No, the Antisocial scale does not show equal outcomes in terms of selection rate. The selection ratio is much lower in girls \((0.10)\) compared to boys \((0.05)\).
- The measure could be unbiased if it shows the same intercepts and slopes across groups in predicting college performance. However, a measure can still be unfair even it is unbiased. If there are different types of errors in different groups, the measure may not be fair. For instance, if there are more false negative errors in Black applicants compared to White applicants and more false positive errors in White applicants than Black applicants, the measure would be unfair against Black applicants. Even if the percent accuracy of prediction is the same for Black and White applicants, the errors have different implications for each group—White applicants who would have failed in college are more likely to be accepted whereas strong Black applicants who would have succeeded are less likely to be accepted. To address the unfairness of the test, it will be important to use one of the various operationalizations of fairness: equal outcomes, equal opportunity, or equal odds.
- The model with item parameters constrained across sexes fit significantly worse than a model with item parameters that were allowed to vary across sexes, \(\chi^2[-19] = -119.12, p < .001\). This suggests that the item parameters show differential item functioning across boys and girls.
- No items showed significant differences in discrimination across sexes, suggesting that items did not differ in their relation to the latent factor across boys and girls. Items 1, 2, 3, 4, 5, and 6 differed in severity across sexes. Items showed greater severity for girls than boys. It took a higher level of antisocial behavior for a girl to be endorsed as engaging in one of the following behaviors: cheats or tells lies, bullies or is cruel/mean to others, does not seem to feel sorry after misbehaving, breaks things deliberately, is disobedient at school, and has trouble getting along with teachers.
- The differences in item severity across boys and girls could be addressed by resolving DIF. That is, the items that show severity DIF (items 1–6) would be allowed to differ in their severity parameter across boys and girls, but these items would be constrained to have the same discrimination across groups; non-DIF items would have severity and discrimination parameters constrained to be the same across groups.
- The configural invariance model fit well according to RMSEA \((0.09)\) and SRMR \((0.06)\), but fit less well according to CFI \((0.77)\). The metric invariance model did not fit significantly worse than the configural invariance model \((\Delta\chi^2[6] = 8.77, p = 0.187)\). However, the scalar invariance model fit significantly worse than the metric invariance model \((\Delta\chi^2[6] = 21.28, p = 0.002)\), and the residual invariance model fit significantly worse than the scalar invariance model \((\Delta\chi^2[7] = 42.80, p < .001)\). Therefore, scalar and residual invariance failed. The following items showed larger intercepts at T1 compared to T2: items 1, 2, 3, 4, 5, and 7. Only item 6 showed larger intercepts at T2 than T1. The following items showed a larger residual at T1 compared to T2: items 2–7. Only item 1 showed a larger residual at T2 compared to T1.